Predictive Dosimetry Using Machine Learning to Guide Dental Management and Extractions Prior to Head and Neck Radiotherapy
International journal of radiation oncology, biology, physics(2019)
摘要
Dental decay and osteoradionecrosis are major late sequelae of head and neck radiotherapy but there is little concrete basis to counsel patients about their individual risk. At our institution, dental oncologists may treat or extract teeth when the anticipated dose to the region is high, e.g. >50 Gy. We investigated the feasibility of dose prediction using either a complete or a limited contour set to determine if predictive accuracy could be maintained while preserving efficiency. We also investigated the feasibility of using diagnostic imaging to predict doses to the mandible. A machine learning (ML) dose prediction model was trained using 86 oropharyngeal cancer radiation treatment plans delivered at a high-volume academic center. The model uses a boosting-based method, which forms a final strong classifier with respect to a distribution through iterative learning of weak classifiers. A test set of 14 treatment planning CTs was processed using a commercial ML decision support software with either a complete set of all targets and organs at risk (OARs) or a limited contour set comprised of the gross tumor planning target volume (PTV) and mandible aloneAll contours were manually delineated with the exception of mandible sub-structures, which were auto-segmented using commercial software. Predictive accuracy was defined as the mean absolute error (MAE) between delivered and predicted dose. The trained ML algorithm was then used to predict dosimetry for two patients using limited contour sets contoured on diagnostic CTs. Max doses could be predicted within MAE of 2.41 Gy for mandible and on average, within 6.19 Gy for mandible sub-structures using complete contour sets (Table 1). Although dose prediction for 8 out of 10 indices was improved using complete rather than limited contour sets, MAE on average improved by only 7.5%. As proof of principle, limited contour sets were delineated on diagnostic CTs for two patients for dose prediction prior to CT simulation. For these patients, while the MAE for 14 dental OARs was relatively high at 6.94 Gy, the errors were most pronounced below the 50 Gy threshold in regions far from the targets and OARs; only 1 of 14 (7%) total OARs was incorrectly predicted to receive a dose >50 Gy. Diagnostic imaging can be used with minimal contouring for dose prediction of high-risk OARs using To our knowledge, this represents the first meaningful applied prospective use of ML-based clinical counseling prior to radiation treatment.Abstract 3819; Table 1Dose prediction using a complete contour set or mandible and PTV alone. MAE and standard deviation (SD) are shownStructureComplete Contour SetMand/ PTV AloneMAE (Gy)SD (Gy)MAE (Gy)SD (Gy)L Ant Mand Max5.932.977.444.79R Ant Mand Max6.564.966.926.58L Mid Mand Max5.954.398.223.20R Mid Mand Max7.304.377.523.91L Post Mand Max4.704.044.543.75R Post Mand Max6.713.607.233.31Mand Max2.412.642.312.71PTV Max1.070.771.050.72PTV D99%3.004.483.484.67PTV D95%0.921.121.001.07 Open table in a new tab
更多查看译文
AI 理解论文
溯源树
样例
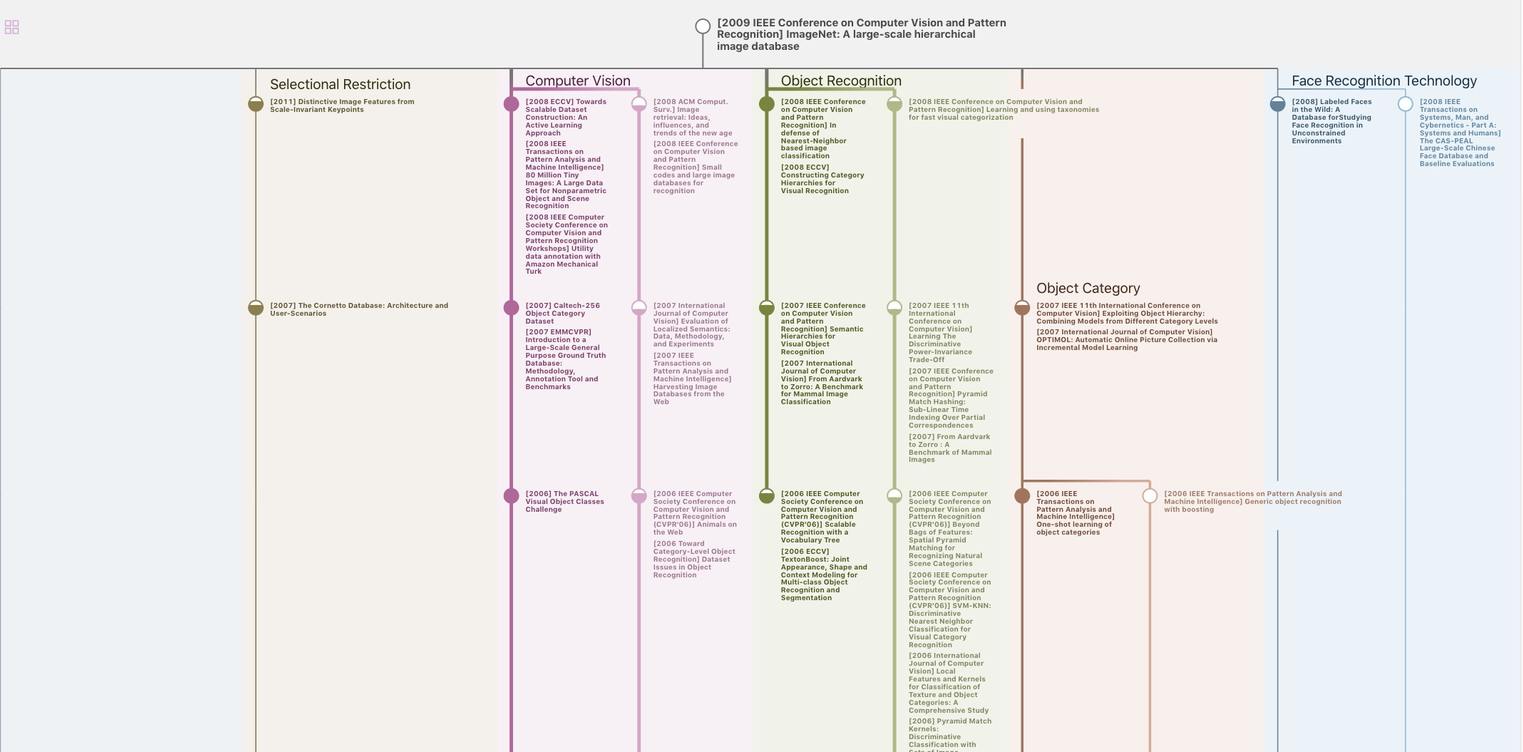
生成溯源树,研究论文发展脉络
Chat Paper
正在生成论文摘要