Non-Convergence and Limit Cycles in the Adam optimizer
ARTIFICIAL NEURAL NETWORKS AND MACHINE LEARNING - ICANN 2019: DEEP LEARNING, PT II(2022)
摘要
One of the most popular training algorithms for deep neural networks is the Adaptive Moment Estimation (Adam) introduced by Kingma and Ba. Despite its success in many applications there is no satisfactory convergence analysis: only local convergence can be shown for batch mode under some restrictions on the hyperparameters, counterexamples exist for incremental mode. Recent results show that for simple quadratic objective functions limit cycles of period 2 exist in batch mode, but only for atypical hyperparameters, and only for the algorithm without bias correction. %More general there are several more adaptive gradient methods which try to estimate a fitting learning rate and / or search direction from the training data to improve the learning process compared to pure gradient descent with fixed learningrate. We extend the convergence analysis for Adam in the batch mode with bias correction and show that even for quadratic objective functions as the simplest case of convex functions 2-limit-cycles exist, for all choices of the hyperparameters. We analyze the stability of these limit cycles and relate our analysis to other results where approximate convergence was shown, but under the additional assumption of bounded gradients which does not apply to quadratic functions. The investigation heavily relies on the use of computer algebra due to the complexity of the equations.
更多查看译文
关键词
Adam optimizer, Convergence, Computer algebra, Dynamical system, Limit cycle
AI 理解论文
溯源树
样例
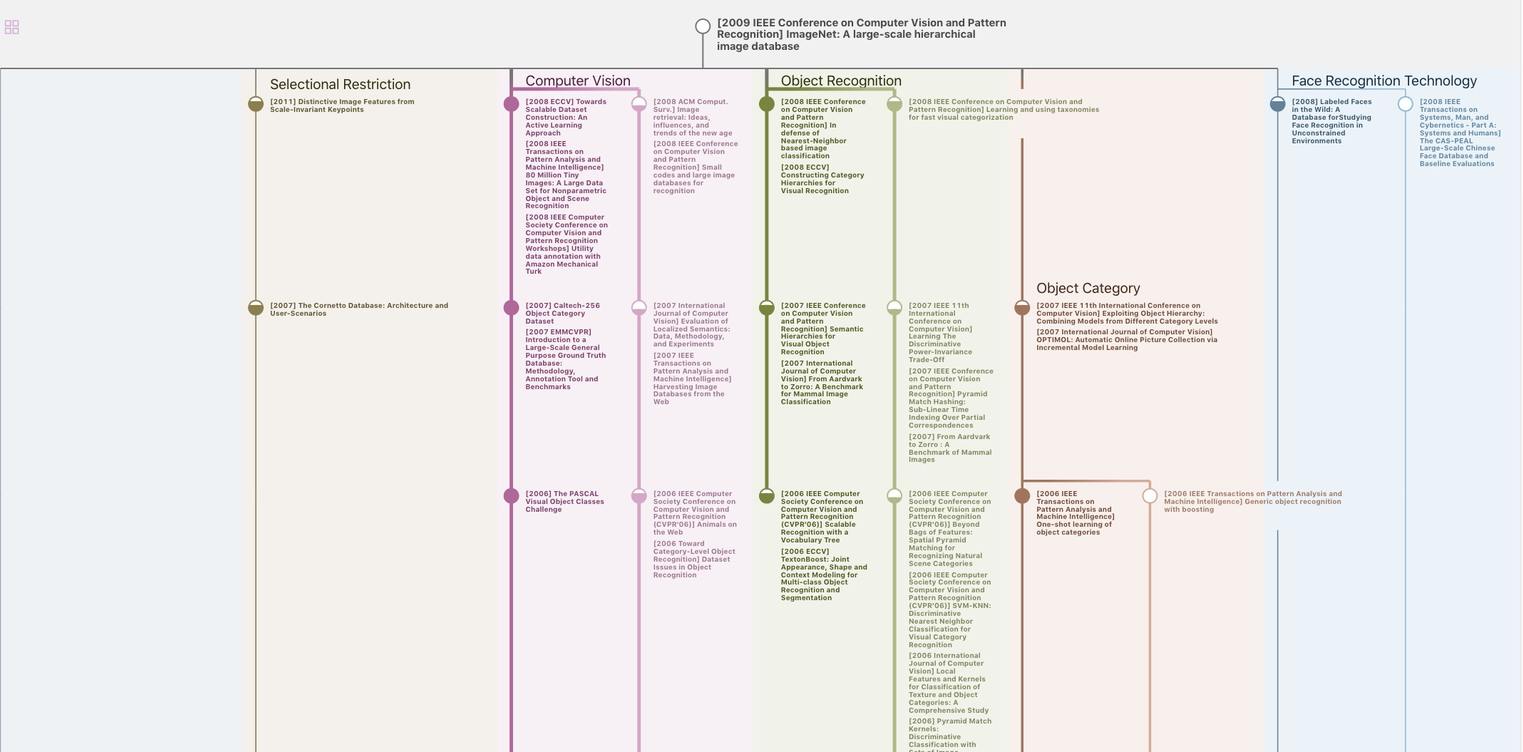
生成溯源树,研究论文发展脉络
Chat Paper
正在生成论文摘要