Geometric Surface Feature Detection Using Statistical Based Metrics
Precision engineering(2019)
摘要
The nature and quality of a component's surface affects its final functionality, expected life span, and perceived value. The economic advantage gained by achieving the desired surface quality drives interest in methods capable of detecting and quantifying surface topography features. This paper presents a new statistically based approach capable of providing insights on the geometric characteristics of a surface, and both detecting and quantifying isolated geometric surface features. In this approach an areal surface measurement, post removal of form and waviness components, is considered as a set of constituent profile measurements, i.e. each column of data is considered as a single profile. Analysis of this data set's variance can detect the presence of surface anisotropy, the spatial wavelength of repeating features, the presence of scratches and localized surface imperfections (dirt or digs). The paper outlines the method's capabilities and limitations, and draws parallels with surface texture parameters described within the ISO 25178-2 standard.
更多查看译文
关键词
Surface metrology,Feature characterization,Statistical analysis
AI 理解论文
溯源树
样例
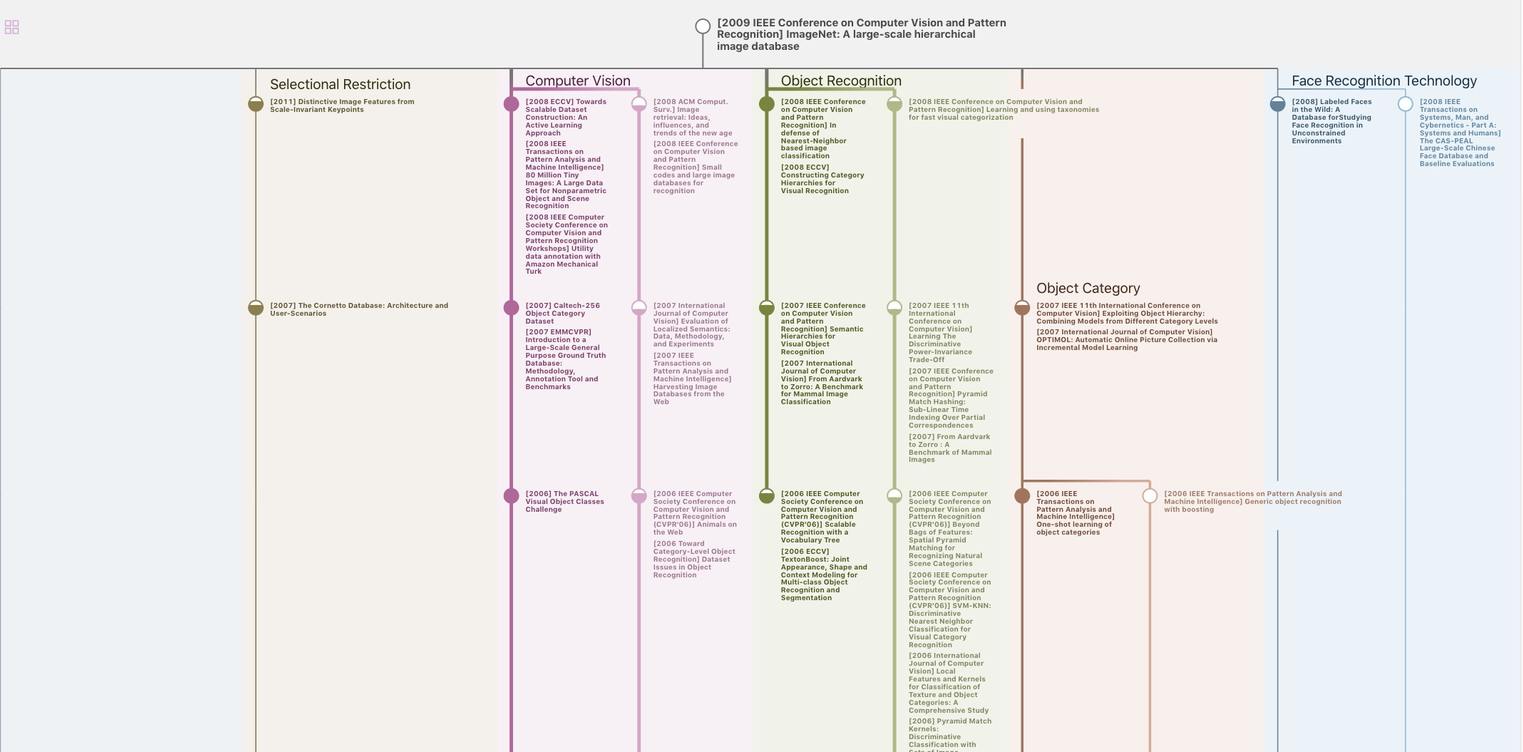
生成溯源树,研究论文发展脉络
Chat Paper
正在生成论文摘要