Multi-view correlation tracking with adaptive memory-improved update model
Neural Computing and Applications(2019)
摘要
Recently, some researchers concentrate on applying multi-view learning to the correlation filter tracking to achieve both the efficiency and accuracy. However, most of them fail to effectively collaborate multiple views to deal with more complex environment. Moreover, their methods are prone to drift in case of long-term occlusion due to the memory loss. In this paper, we propose a novel multi-view correlation filters-based tracker for robust visual tracking. First, we present an adaptive multi-view collaboration strategy to highlight different contributions of different views by jointly considering the reliability and discrimination. Second, an effective memory-improved model update rule is introduced to avoid falling into a contaminated target model. Compared with the conventional linear interpolation update rule, it can effectively deal with long-term occlusion by improving the memory of historical models. Furthermore, instead of assigning a unified learning rate for all views in each frame, we design varying learning rates for different views according to their respective evaluations on the current tracking result, which can prevent the target models of all views from being contaminated at the same time. Finally, a failure-aware scale update scheme is developed to avoid noisy scale estimation in case of temporal tracking failure. Extensive experimental results on the recent benchmark demonstrate that our tracker performs favorably against other state-of-the-art trackers with a real-time performance.
更多查看译文
关键词
Visual tracking,Correlation filter,Multi-view learning,Scale estimation
AI 理解论文
溯源树
样例
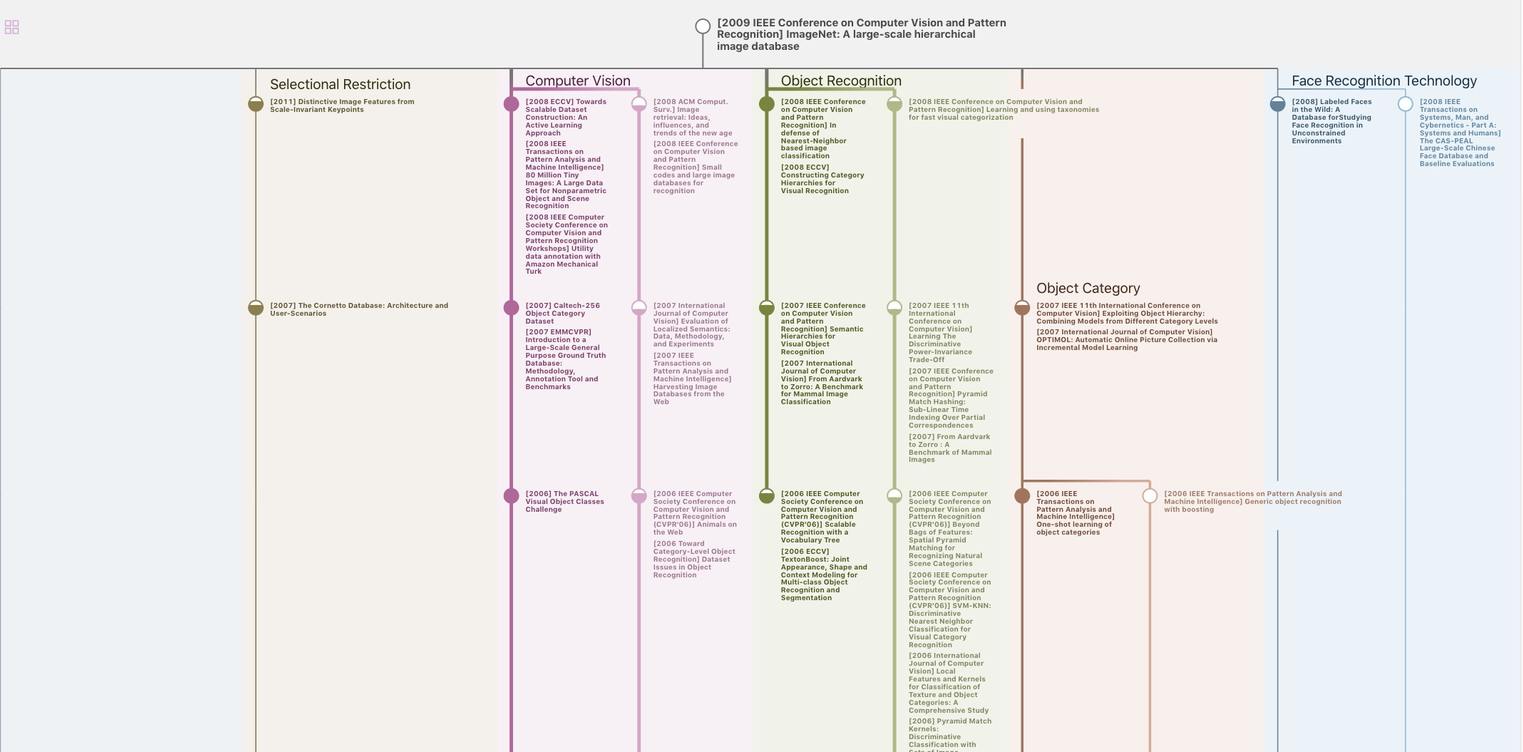
生成溯源树,研究论文发展脉络
Chat Paper
正在生成论文摘要