Semi-Supervised Multi-Modal Multi-Instance Multi-Label Deep Network with Optimal Transport
IEEE TRANSACTIONS ON KNOWLEDGE AND DATA ENGINEERING(2021)
摘要
Complex objects are usually with multiple labels, and can be represented by multiple modal representations, e.g., the complex articles contain text and image information as well as multiple annotations. Previous methods assume that the homogeneous multi-modal data are consistent, while in real applications, the raw data are disordered, e.g., the article constitutes with variable number of inconsistent text and image instances. Therefore, Multi-modal Multi-instance Multi-label (M3) learning provides a framework for handling such task and has exhibited excellent performance. However, M3 learning is facing two main challenges: 1) how to effectively utilize label correlation and 2) how to take advantage of multi-modal learning to process unlabeled instances. To solve these problems, we first propose a novel Multi-modal Multi-instance Multi-label Deep Network (M3DN), which considers M3 learning in an end-to-end multi-modal deep network and utilizes consistency principle among different modal bag-level predictions. Based on the M3DN, we learn the latent ground label metric with the optimal transport. Moreover, we introduce the extrinsic unlabeled multi-modal multi-instance data, and propose the M3DNS, which considers the instance-level auto-encoder for single modality and modified bag-level optimal transport to strengthen the consistency among modalities. Thereby M3DNS can better predict label and exploit label correlation simultaneously. Experiments on benchmark datasets and real world WKG Game-Hub dataset validate the effectiveness of the proposed methods.
更多查看译文
关键词
Semi-supervised learning,multi-modal multi-instance multi-label learning,modal consistency,optimal transport
AI 理解论文
溯源树
样例
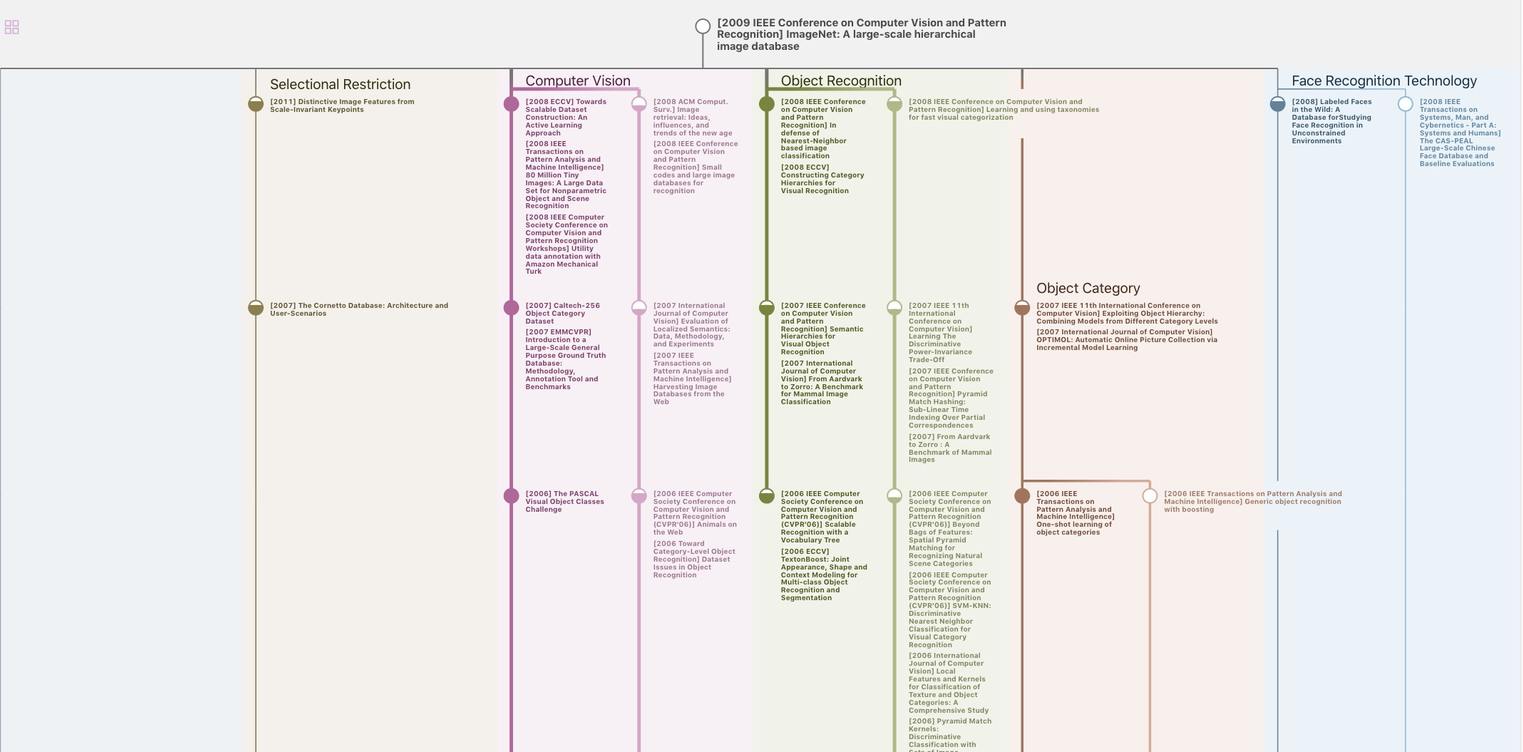
生成溯源树,研究论文发展脉络
Chat Paper
正在生成论文摘要