Robust training with ensemble consensus
CoRR(2020)
摘要
Since deep neural networks are over-parameterized, they can memorize noisy examples. We address such memorizing issue in the presence of annotation noise. From the fact that deep neural networks cannot generalize neighborhoods of the features acquired via memorization, we hypothesize that noisy examples do not consistently incur small losses on the network under a certain perturbation. Based on this, we propose a novel training method called Learning with Ensemble Consensus (LEC) that prevents overfitting noisy examples by eliminating them using the consensus of an ensemble of perturbed networks. One of the proposed LECs, LTEC outperforms the current state-of-the-art methods on noisy MNIST, CIFAR-10, and CIFAR-100 in an efficient manner.
更多查看译文
关键词
Annotation noise, Noisy label, Robustness, Ensemble, Perturbation
AI 理解论文
溯源树
样例
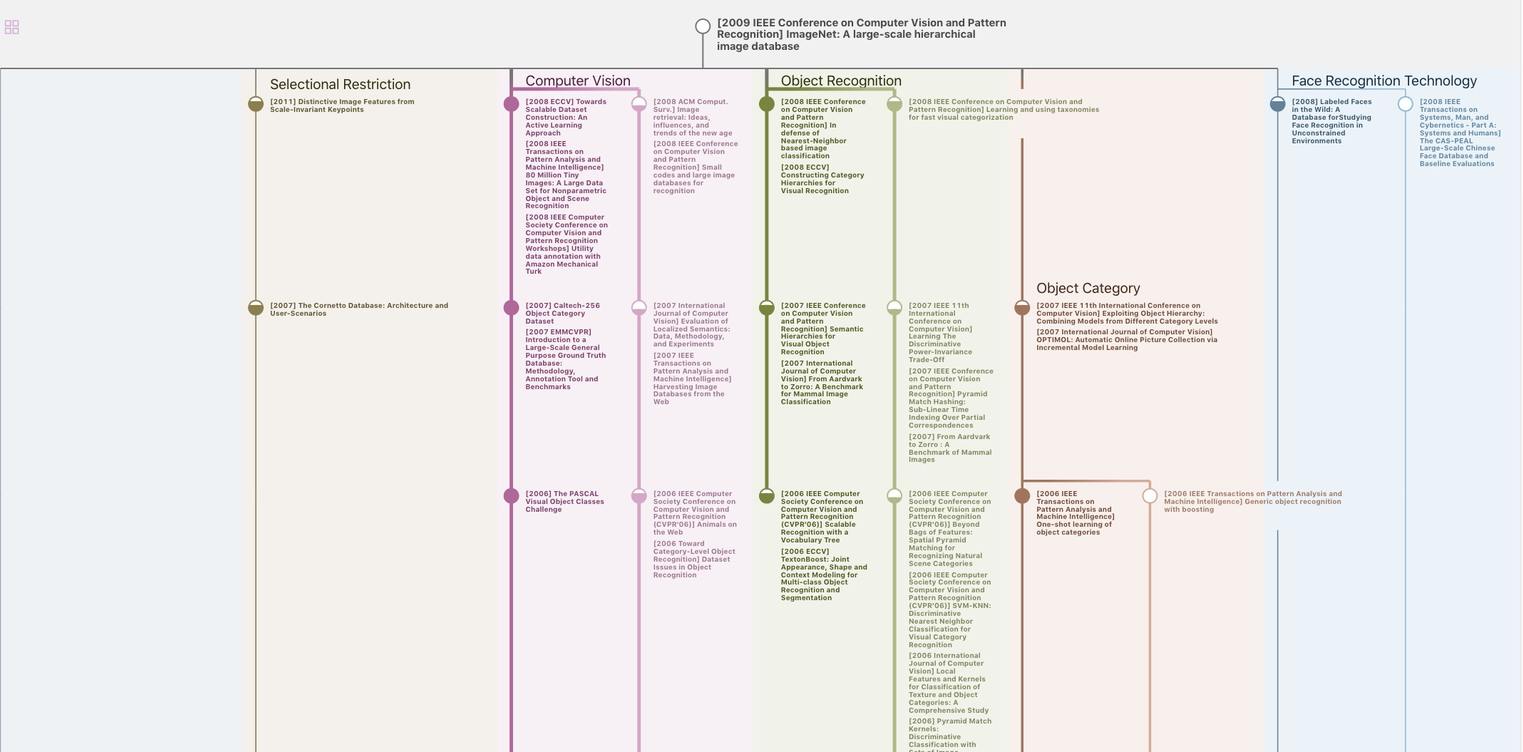
生成溯源树,研究论文发展脉络
Chat Paper
正在生成论文摘要