Forecasting under Long Memory and Nonstationarity
arXiv (Cornell University)(2019)
摘要
Long memory in the sense of slowly decaying autocorrelations is a stylized fact in many time series from economics and finance. The fractionally integrated process is the workhorse model for the analysis of these time series. Nevertheless, there is mixed evidence in the literature concerning its usefulness for forecasting and how forecasting based on it should be implemented. Employing pseudo-out-of-sample forecasting on inflation and realized volatility time series and simulations we show that methods based on fractional integration clearly are superior to alternative methods not accounting for long memory, including autoregressions and exponential smoothing. Our proposal of choosing a fixed fractional integration parameter of $d=0.5$ a priori yields the best results overall, capturing long memory behavior, but overcoming the deficiencies of methods using an estimated parameter. Regarding the implementation of forecasting methods based on fractional integration, we use simulations to compare local and global semiparametric and parametric estimators of the long memory parameter from the Whittle family and provide asymptotic theory backed up by simulations to compare different mean estimators. Both of these analyses lead to new results, which are also of interest outside the realm of forecasting.
更多查看译文
关键词
Multivariate Analysis
AI 理解论文
溯源树
样例
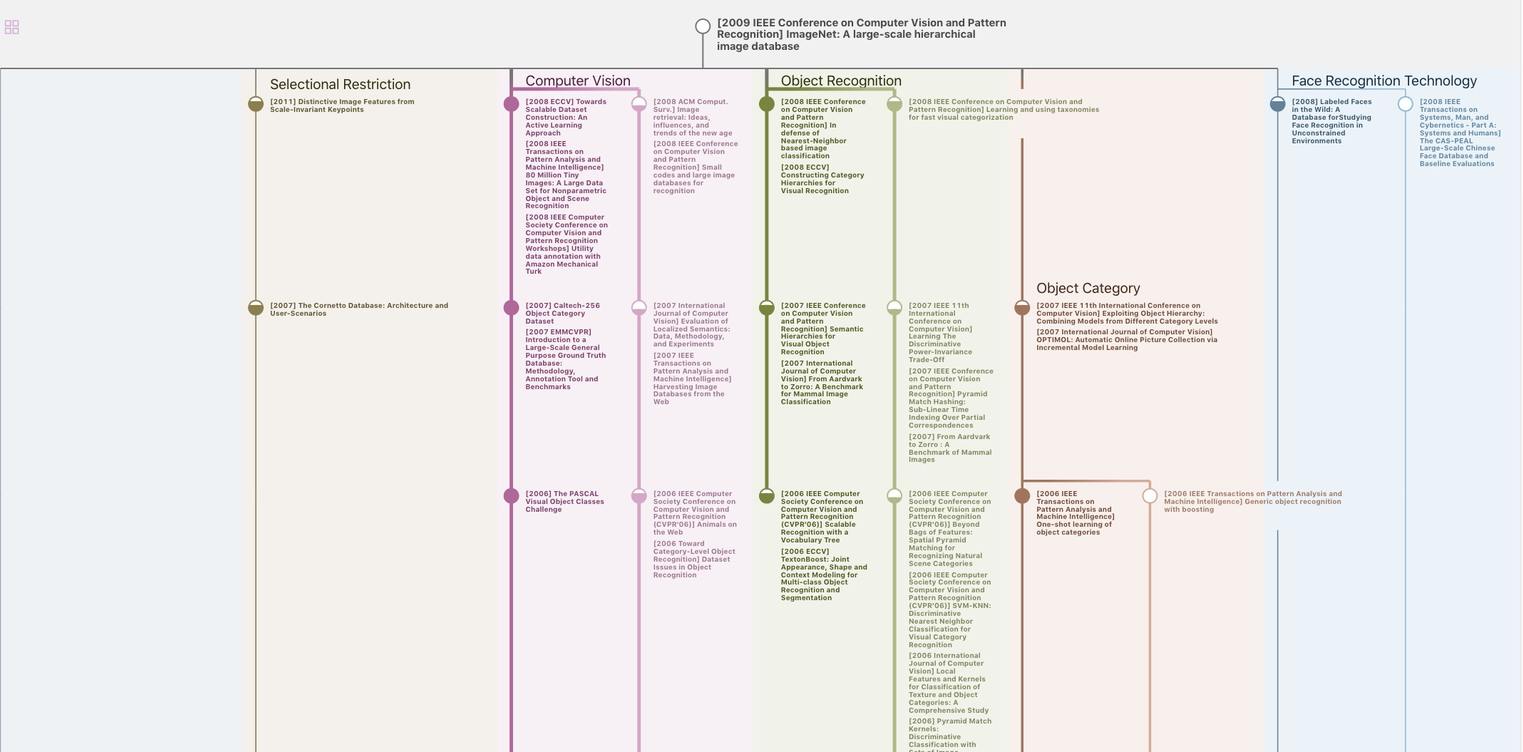
生成溯源树,研究论文发展脉络
Chat Paper
正在生成论文摘要