Deformable Generator Networks: Unsupervised Disentanglement of Appearance and Geometry
IEEE transactions on pattern analysis and machine intelligence(2022)
摘要
We present a deformable generator model to disentangle the appearance and geometric information for both image and video data in a purely unsupervised manner. The appearance generator network models the information related to appearance, including color, illumination, identity or category, while the geometric generator performs geometric warping, such as rotation and stretching, through generating deformation field which is used to warp the generated appearance to obtain the final image or video sequences. Two generators take independent latent vectors as input to disentangle the appearance and geometric information from image or video sequences. For video data, a nonlinear transition model is introduced to both the appearance and geometric generators to capture the dynamics over time. The proposed scheme is general and can be easily integrated into different generative models. An extensive set of qualitative and quantitative experiments shows that the appearance and geometric information can be well disentangled, and the learned geometric generator can be conveniently transferred to other image datasets that share similar structure regularity to facilitate knowledge transfer tasks.
更多查看译文
关键词
Generators,Deformable models,Data models,Shape,Interpolation,Analytical models,Image color analysis,Unsupervised learning,deep generative model,deformable model
AI 理解论文
溯源树
样例
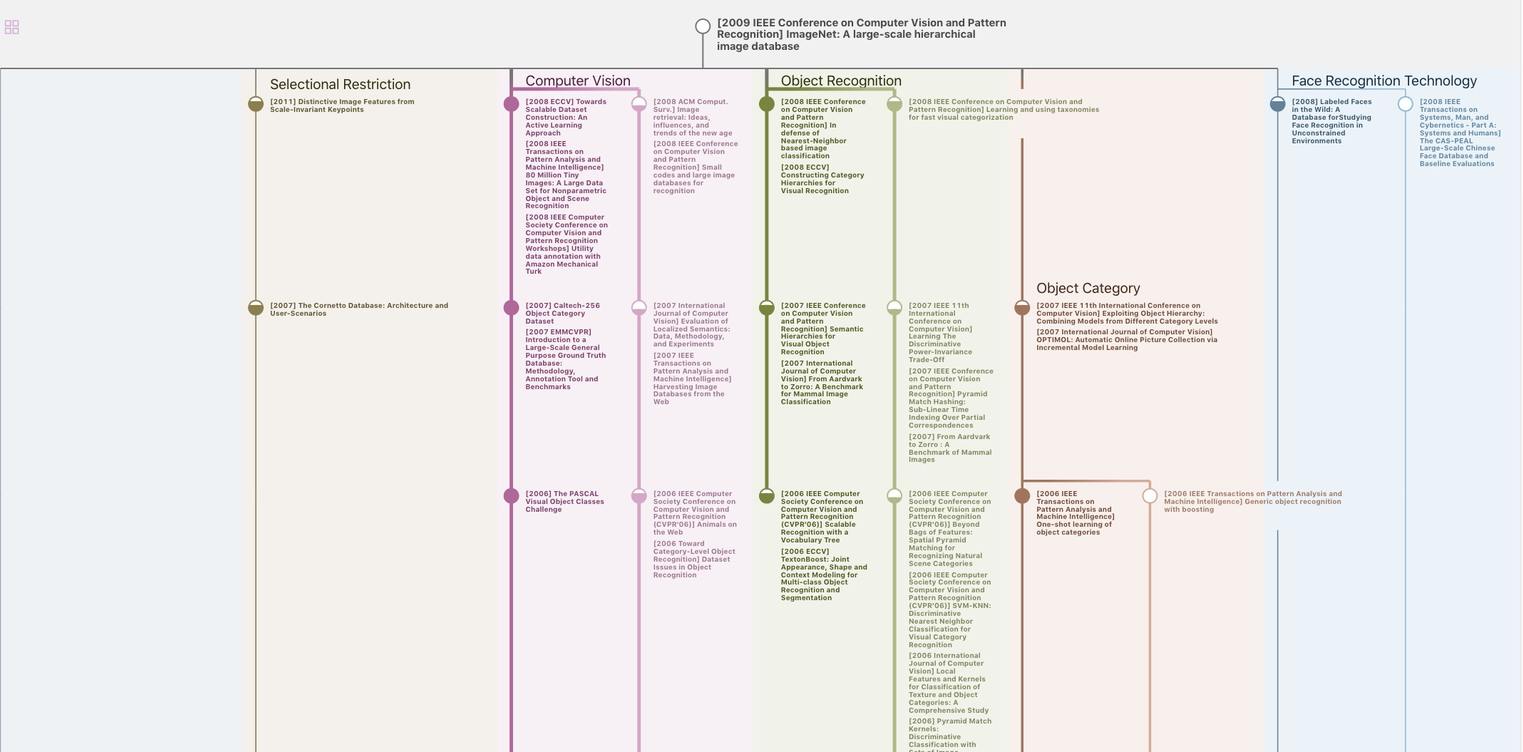
生成溯源树,研究论文发展脉络
Chat Paper
正在生成论文摘要