Swarm Intelligence Optimized Generative Model for Network Performance Prediction.
HPCC/SmartCity/DSS(2019)
摘要
Network state prediction methods are widely used currently to evaluate network performance instead of monitors or detectors because of its low-cost and timeliness. However, existing prediction methods are generally based on historical time-series data and insensitive to changes of external conditions. In this paper, based on the state-of-the-art deep generative model Conditional Variational Auto-Encoder, we propose a Network Performance Prediction method using Generative Model (NPGM). This method builds the distribution of network latency features according to historical data with traffic taken as condition. Generative model can deal with hidden variables when building the model which traditional methods cannot handle. Following the ideology of phase space reconstruction, we conceal the numerous factors and map the inherent properties of network into a hidden vector. In order to speed up training process, we optimize parameters of neural networks with our Self-adaptive Stochastic Particle Swarm Optimization (SPSO) instead of Gradient Descent (GD) algorithms. This approach applies self-adaptive coefficients and mutation operation, which improves training efficiency and searching accuracy. We propose a new criterion called Weighted Matrix Mean Absolute Percentage Error (WM-MAPE) to evaluate the predicting accuracy of latency matrices. We use real commercial backbone traffic data from the Center for Applied Internet Data Analysis database. Results on validation sets show that inference error of our approach is less than 50% of time-series-based methods and SPSO has a significantly better efficiency and convergence compared with GD and PSO algorithms.
更多查看译文
关键词
latency inference,generative model,hidden vector,self-adaptive coefficients,mutation
AI 理解论文
溯源树
样例
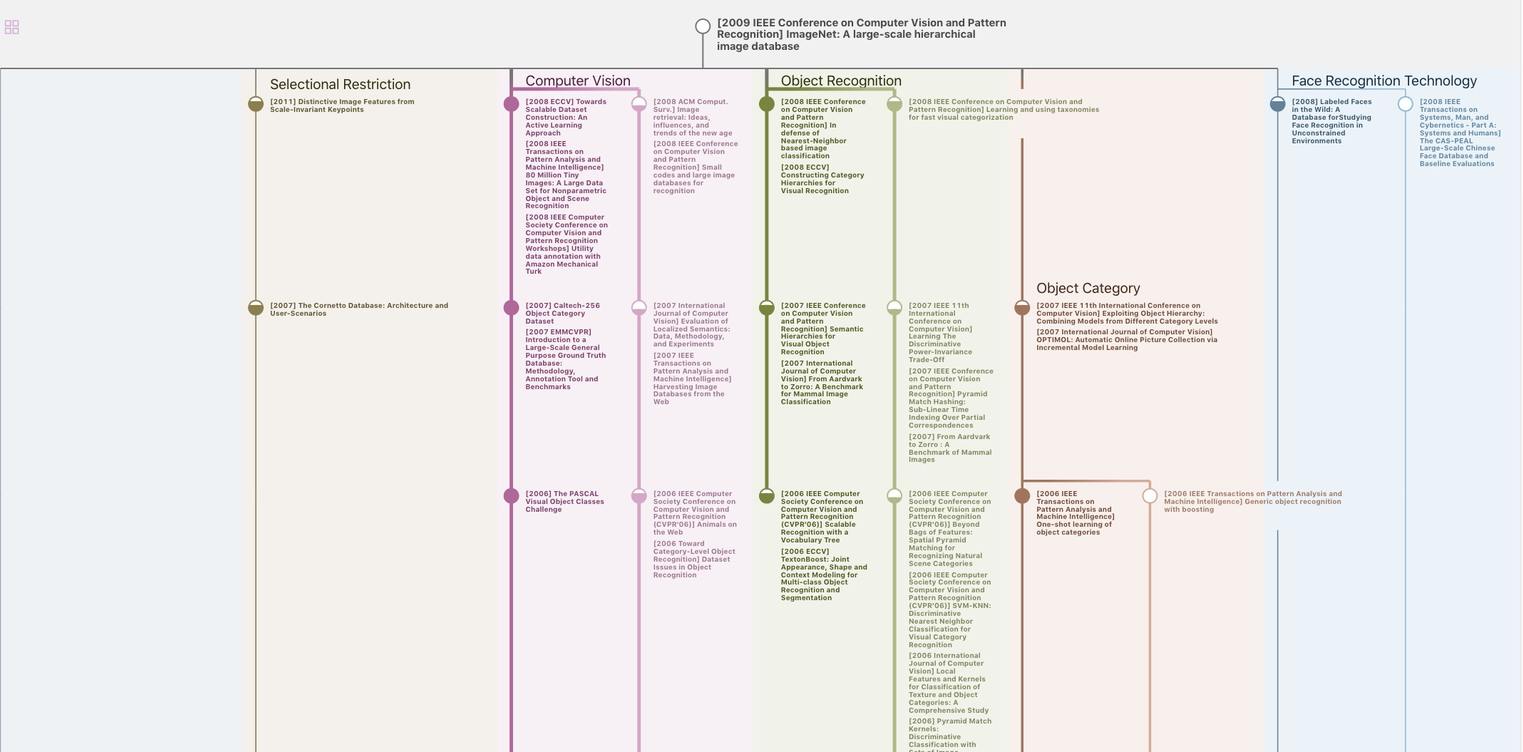
生成溯源树,研究论文发展脉络
Chat Paper
正在生成论文摘要