A Humanized Yeast Phenomic Model of Deoxycytidine Kinase to Predict Genetic Buffering of Nucleoside Analog Cytotoxicity.
GENES(2019)
Abstract
Knowledge about synthetic lethality can be applied to enhance the efficacy of anticancer therapies in individual patients harboring genetic alterations in their cancer that specifically render it vulnerable. We investigated the potential for high-resolution phenomic analysis in yeast to predict such genetic vulnerabilities by systematic, comprehensive, and quantitative assessment of drug-gene interaction for gemcitabine and cytarabine, substrates of deoxycytidine kinase that have similar molecular structures yet distinct antitumor efficacy. Human deoxycytidine kinase (dCK) was conditionally expressed in the Saccharomyces cerevisiae genomic library of knockout and knockdown (YKO/KD) strains, to globally and quantitatively characterize differential drug-gene interaction for gemcitabine and cytarabine. Pathway enrichment analysis revealed that autophagy, histone modification, chromatin remodeling, and apoptosis-related processes influence gemcitabine specifically, while drug-gene interaction specific to cytarabine was less enriched in gene ontology. Processes having influence over both drugs were DNA repair and integrity checkpoints and vesicle transport and fusion. Non-gene ontology (GO)-enriched genes were also informative. Yeast phenomic and cancer cell line pharmacogenomics data were integrated to identify yeast-human homologs with correlated differential gene expression and drug efficacy, thus providing a unique resource to predict whether differential gene expression observed in cancer genetic profiles are causal in tumor-specific responses to cytotoxic agents.
MoreTranslated text
Key words
yeast phenomics,gene-drug interaction,genetic buffering,quantitative high throughput cell array phenotyping (Q-HTCP),cell proliferation parameters (CPPs),gemcitabine,cytarabine,recursive expectation-maximization clustering (REMc),pharmacogenomics
AI Read Science
Must-Reading Tree
Example
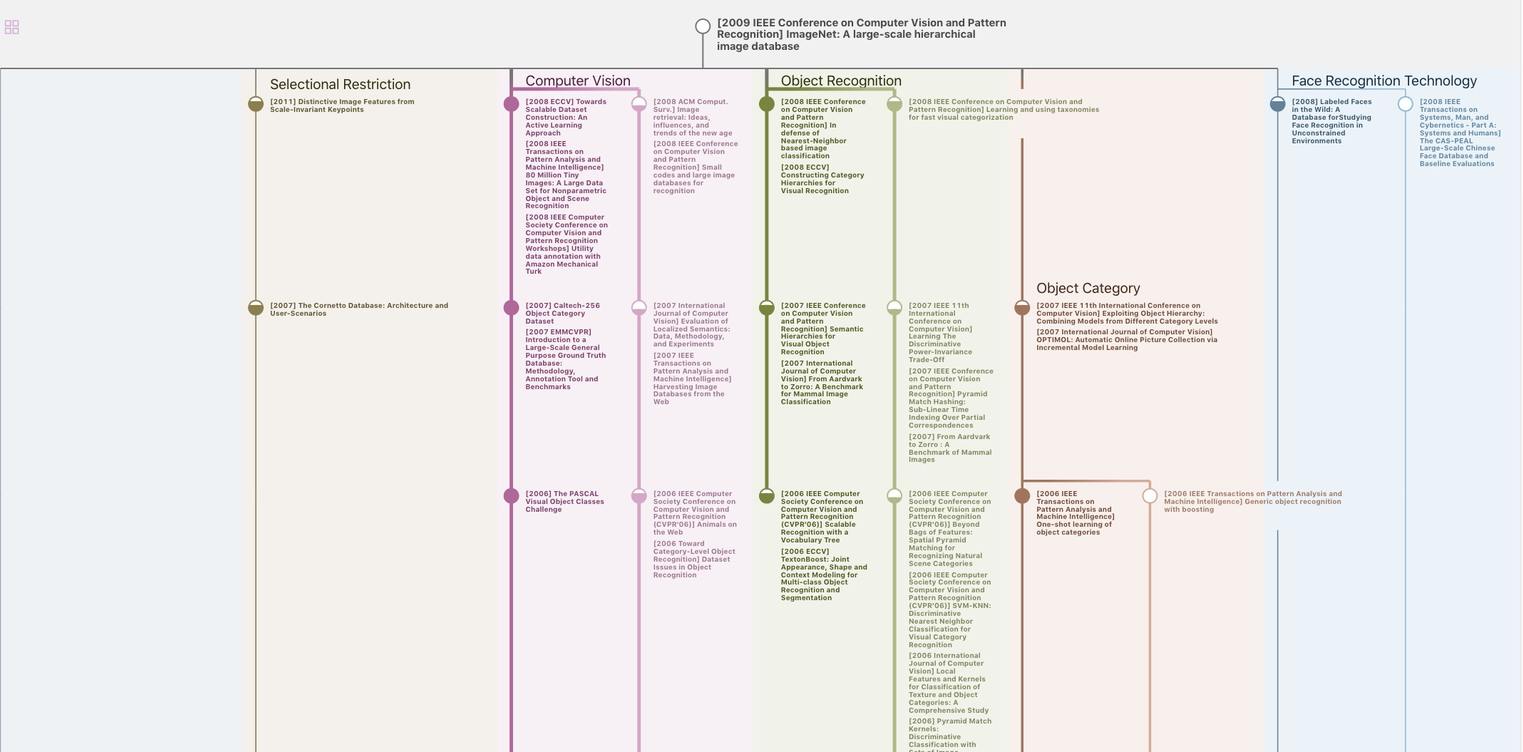
Generate MRT to find the research sequence of this paper
Chat Paper
Summary is being generated by the instructions you defined