Differential Approximation and Sprinting for Multi-Priority Big Data Engines
arXiv (Cornell University)(2019)
摘要
Today's big data clusters based on the MapReduce paradigm are capable of executing analysis jobs with multiple priorities, providing differential latency guarantees. Traces from production systems show that the latency advantage of high priority jobs comes at the cost of severe latency degradation of low-priority jobs as well as daunting resource waste caused by repetitive eviction and re-execution of low-priority jobs. We advocate a new resource management design that exploits the idea of differential approximation and sprinting. The unique combination of approximation and sprinting avoids the eviction of low-priority jobs and its consequent latency degradation and resource waste. To this end, we designed, implemented and evaluated DIAS, an extension of the Spark processing engine to support deflate jobs by dropping tasks and to sprint jobs. Our experiments on scenarios with two and three priority classes indicate that DIAS achieves up to 90% and 60% latency reduction for low- and high-priority jobs, respectively. DIAS not only eliminates resource waste but also (surprisingly) lowers energy consumption up to 30% at only a marginal accuracy loss for low-priority jobs.
更多查看译文
关键词
differential approximation,sprinting,priorities,Spark,energy savings
AI 理解论文
溯源树
样例
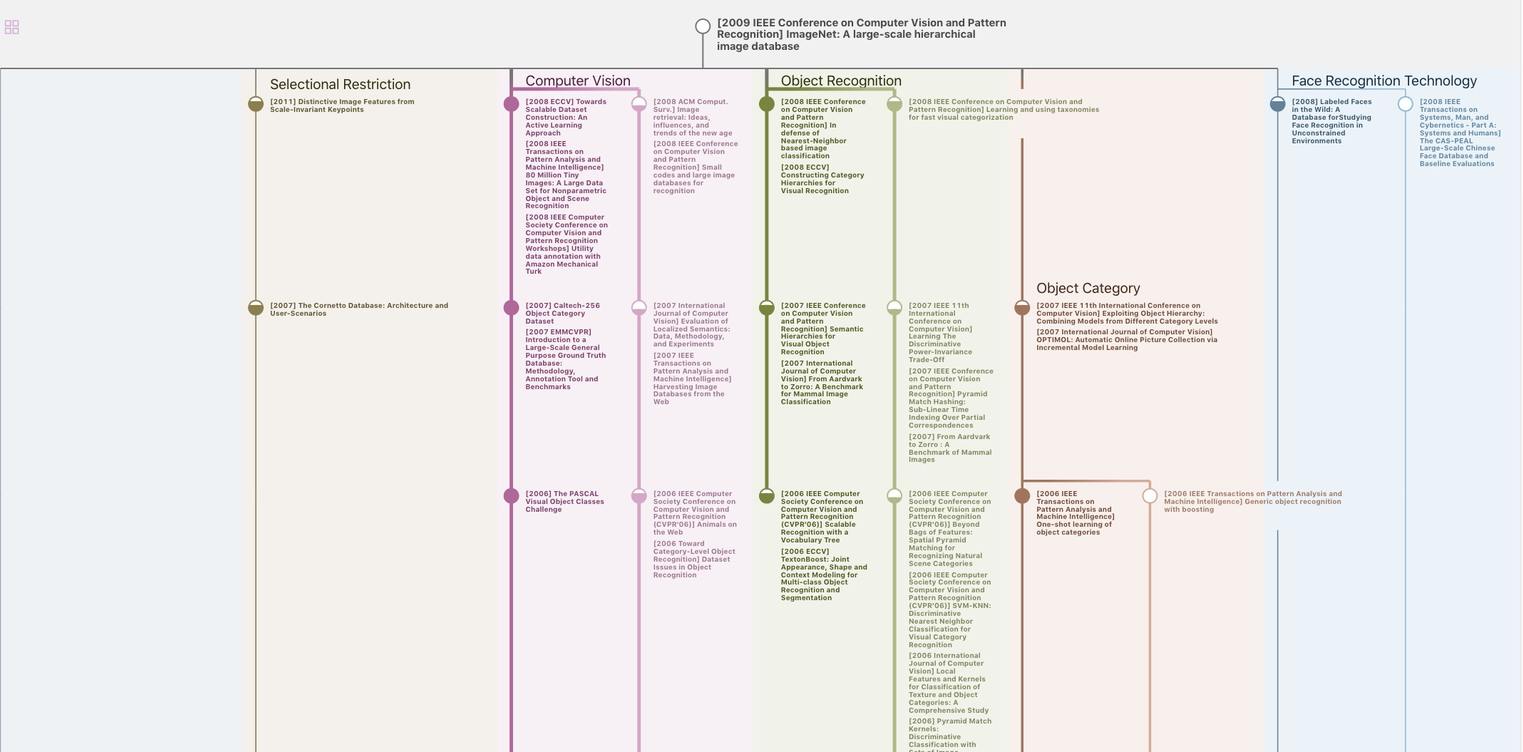
生成溯源树,研究论文发展脉络
Chat Paper
正在生成论文摘要