Attention on Attention for Image Captioning
IEEE International Conference on Computer Vision(2019)
摘要
Attention mechanisms are widely used in current encoder/decoder frameworks of image captioning, where a weighted average on encoded vectors is generated at each time step to guide the caption decoding process. However, the decoder has little idea of whether or how well the attended vector and the given attention query are related, which could make the decoder give misled results. In this paper, we propose an Attention on Attention (AoA) module, which extends the conventional attention mechanisms to determine the relevance between attention results and queries. AoA first generates an information vector and an attention gate using the attention result and the current context, then adds another attention by applying element-wise multiplication to them and finally obtains the attended information, the expected useful knowledge. We apply AoA to both the encoder and the decoder of our image captioning model, which we name as AoA Network (AoANet). Experiments show that AoANet outperforms all previously published methods and achieves a new state-of-the-art performance of 129.8 CIDEr-D score on MS COCO Karpathy offline test split and 129.6 CIDEr-D (C40) score on the official online testing server. Code is available at https://github.com/husthuaan/AoANet.
更多查看译文
关键词
element-wise multiplication,image captioning,weighted average,encoded vectors,caption decoding process,attended vector,attention module,information vector,attention gate,attention query,AoA network,attention mechanisms,encoder/decoder frameworks,attention on attention module,AoANet
AI 理解论文
溯源树
样例
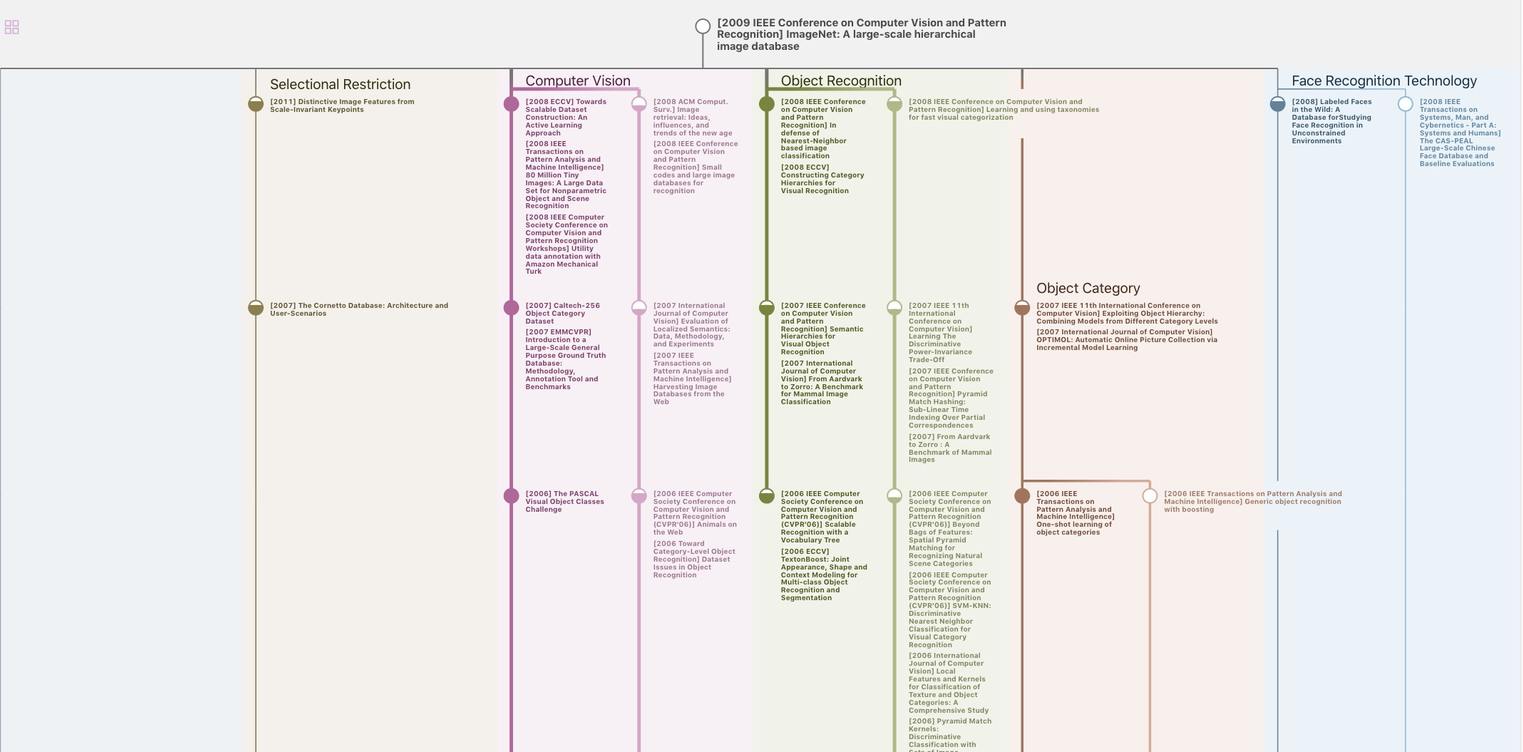
生成溯源树,研究论文发展脉络
Chat Paper
正在生成论文摘要