A Framework for Turning Behavior Classification at Intersections Using 3D LIDAR
IEEE Transactions on Vehicular Technology(2019)
摘要
Turning behavior classification of oncoming vehicles is a critical step toward high-level scene understanding and motion planning tasks for autonomous vehicles. In this paper, we propose a new method for classifying an oncoming vehicle's turning behavior at intersection using 3D LIDAR, i.e., a Velodyne HDL-64E LIDAR, which collects rich point clouds from the surrounding environment. First, the vehicle pose is measured via a novel rectangle-based multi-frame fitting method applied to the vehicle point cloud extracted by a data pre-process procedure. Then, a Kalman filter integrates these measured vehicle poses to estimate and update vehicle kinematic parameters. Next, a hidden Markov model and Bayesian filtering are combined to recognize the vehicle's turning behavior using the heading angle and the acceleration components of the kinematic model. Real point clouds from intersection environments are used to train and validate the proposed turning behavior classification algorithm in the experiments. The results of the performance analysis and classification time measurements confirm that the proposed method can recognize the behavior of the oncoming vehicle at intersections correctly at an early stage of entering an intersection.
更多查看译文
关键词
3D LIDAR,Turning behavior classification,point clouds,pose estimation,hidden Markov model,Bayesian filtering
AI 理解论文
溯源树
样例
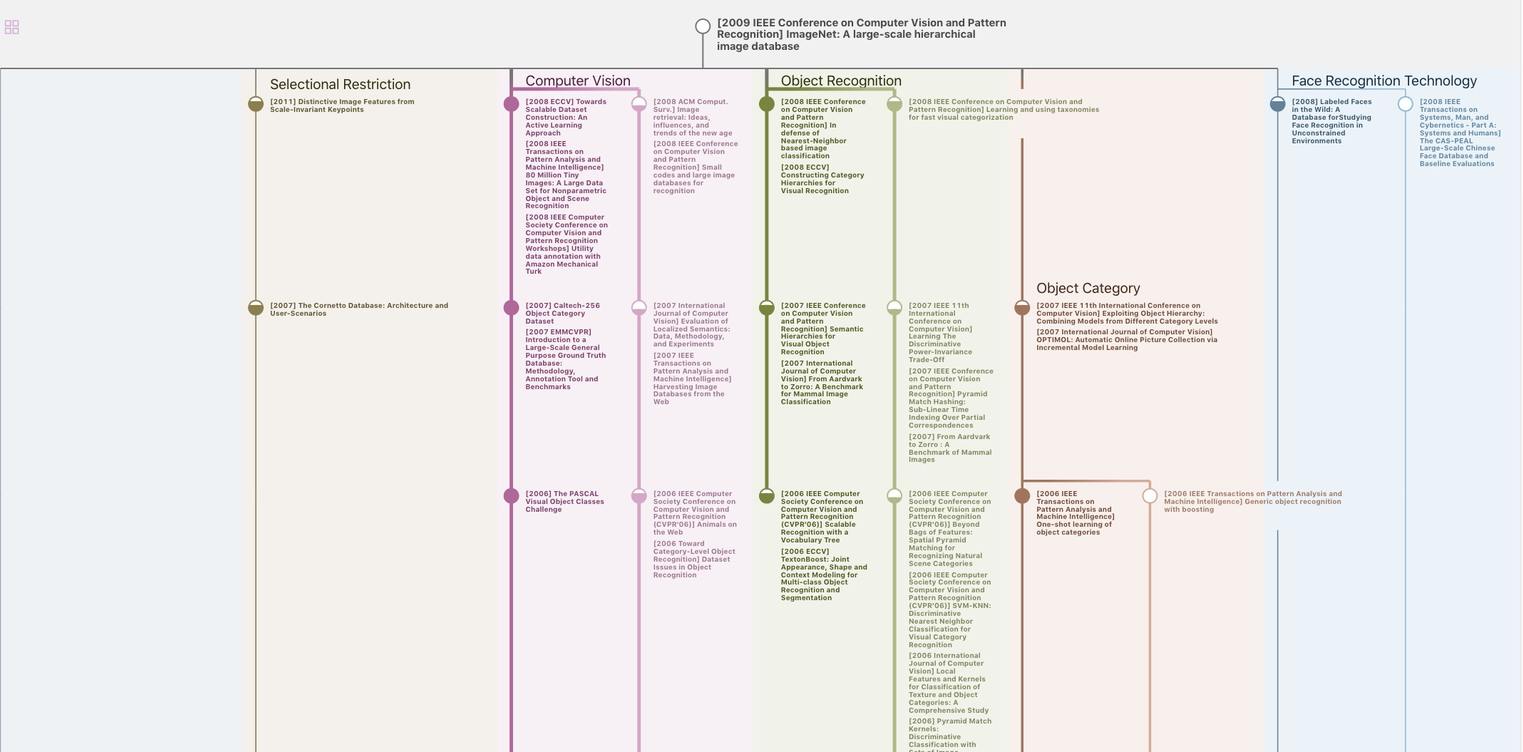
生成溯源树,研究论文发展脉络
Chat Paper
正在生成论文摘要