A 5.1pJ/Neuron 127.3us/Inference RNN-based Speech Recognition Processor using 16 Computing-in-Memory SRAM Macros in 65nm CMOS
2019 Symposium on VLSI Circuits(2019)
摘要
This work presents a 65nm CMOS speech recognition processor, named Thinker-IM, which employs 16 computing-in-memory (SRAM-CIM) macros for binarized recurrent neural network (RNN) computation. Its major contributions are: 1) A novel digital-CIM mixed architecture that runs an output-weight dual stationary (OWDS) dataflow, reducing 85.7% memory accessing; 2) Multi-bit XNOR SRAM-CIM macros and corresponding CIM-aware weight adaptation that reduces 9.9% energy consumption in average; 3) Predictive early batch-normalization (BN) and binarization units (PBUs) that reduce at most 28.3% computations in RNN. Measured results show the processing speed of 127.3us/Inference and over 90.2% accuracy, while achieving neural energy efficiency of 5.1pJ/Neuron, which is 2.8 × better than state-of-the-art.
更多查看译文
关键词
energy consumption,digital-CIM mixed architecture,Thinker-IM,CMOS speech recognition processor,CIM-aware weight adaptation,multibit XNOR SRAM-CIM macros,RNN-based speech recognition processor,batch-normalization,binarized recurrent neural network computation,computing-in-memory SRAM macros,neural energy efficiency,binarization units,output-weight dual stationary dataflow,size 65.0 nm,energy 5.1 pJ
AI 理解论文
溯源树
样例
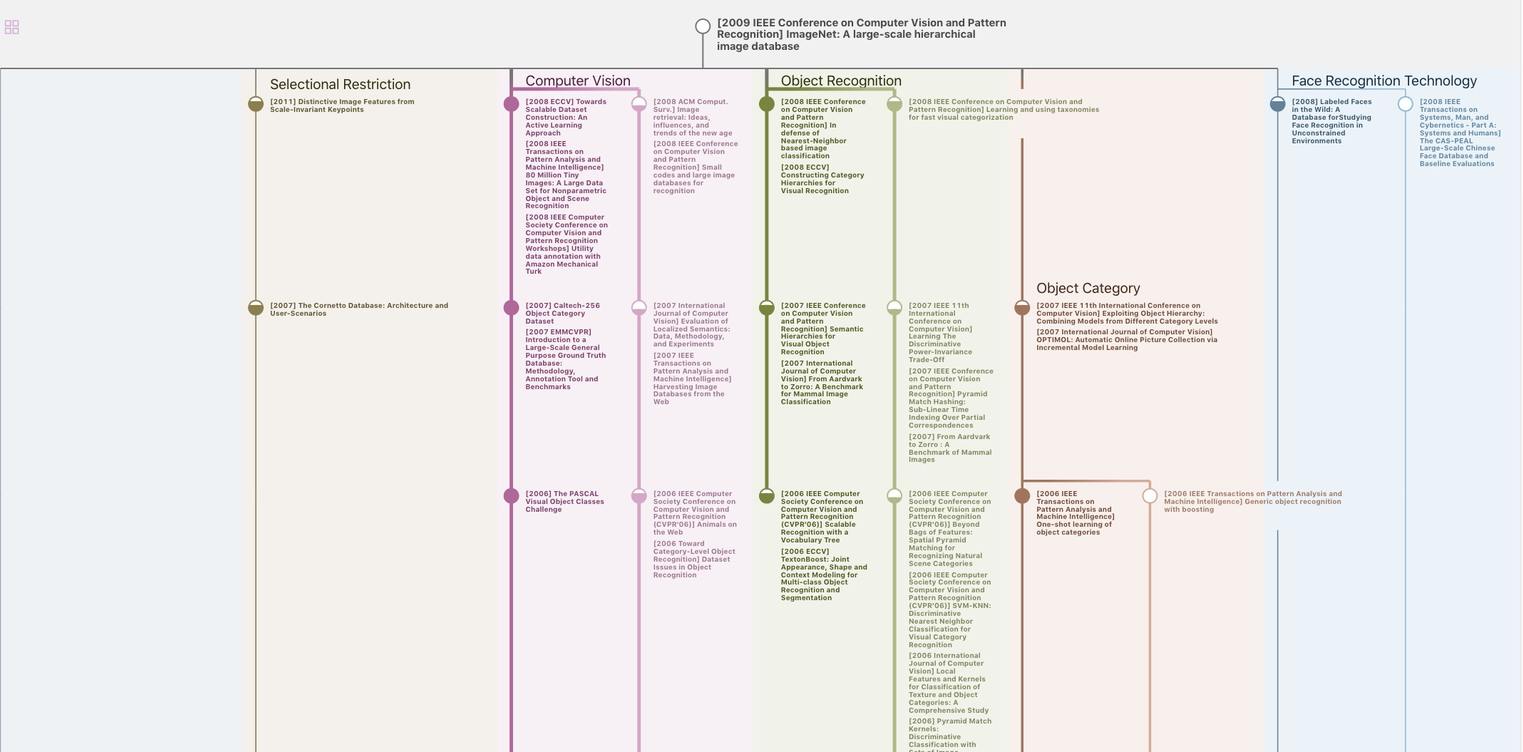
生成溯源树,研究论文发展脉络
Chat Paper
正在生成论文摘要