Eliminating Overfitting of Probabilistic Topic Models on Short and Noisy Text: the Role of Dropout.
International journal of approximate reasoning(2019)
摘要
Probabilistic topic models are powerful tools for discovering hidden structures/semantics in discrete data, e.g., texts, images, links. However, on short and noisy texts, directly applying topic models may not work well or face severe overfitting. In this article, we investigate the benefits of dropout for preventing topic models from overfitting. We integrate dropout into several stochastic methods for learning latent Dirichlet allocation (LDA). From extensive experiments on four large-scale datasets, our findings are: (1) dropout helps to prevent overfitting and significantly enhance predictiveness and generalization of LDA on short texts; (2) for long documents, dropout may provide little benefit; (3) dropout can be easily integrated into any learning methods to avoid overfitting for short and noisy text. Furthermore, dropout can be straightforwardly employed in a wide range of topic models. In evidence, we apply dropout to BTM (Biterm topic model), one of the state-of-the-art models for short texts. Our experiments illustrate that BTM with dropout not only remains its good results in term of predictiveness, but also improves the learning time significantly.
更多查看译文
关键词
Topic models,Short text,Dropout
AI 理解论文
溯源树
样例
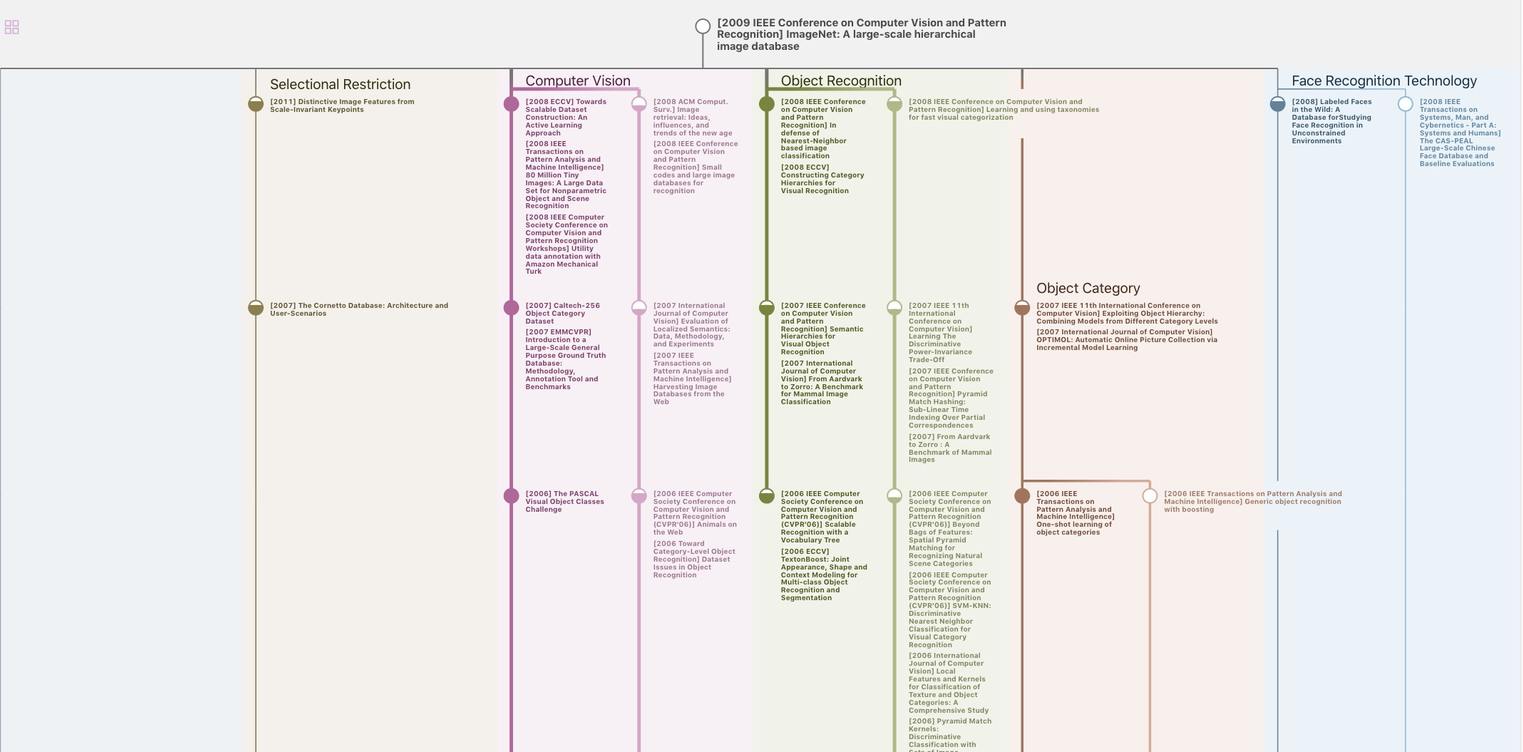
生成溯源树,研究论文发展脉络
Chat Paper
正在生成论文摘要