Acoustic Evaluation of Behavioral States Predicted from GPS Tracking: a Case Study of a Marine Fishing Bat
Movement ecology(2019)SCI 2区
University of Maryland | Tel-Aviv University | Universidad Nacional Autónoma de México
Abstract
Multiple methods have been developed to infer behavioral states from animal movement data, but rarely has their accuracy been assessed from independent evidence, especially for location data sampled with high temporal resolution. Here we evaluate the performance of behavioral segmentation methods using acoustic recordings that monitor prey capture attempts. We recorded GPS locations and ultrasonic audio during the foraging trips of 11 Mexican fish-eating bats, Myotis vivesi, using miniature bio-loggers. We then applied five different segmentation algorithms (k-means clustering, expectation-maximization and binary clustering, first-passage time, hidden Markov models, and correlated velocity change point analysis) to infer two behavioral states, foraging and commuting, from the GPS data. To evaluate the inference, we independently identified characteristic patterns of biosonar calls (“feeding buzzes”) that occur during foraging in the audio recordings. We then compared segmentation methods on how well they correctly identified the two behaviors and if their estimates of foraging movement parameters matched those for locations with buzzes. While the five methods differed in the median percentage of buzzes occurring during predicted foraging events, or true positive rate (44–75%), a two-state hidden Markov model had the highest median balanced accuracy (67%). Hidden Markov models and first-passage time predicted foraging flight speeds and turn angles similar to those measured at locations with feeding buzzes and did not differ in the number or duration of predicted foraging events. The hidden Markov model method performed best at identifying fish-eating bat foraging segments; however, first-passage time was not significantly different and gave similar parameter estimates. This is the first attempt to evaluate segmentation methodologies in echolocating bats and provides an evaluation framework that can be used on other species.
MoreTranslated text
Key words
Behavioral change point analysis,Correlated velocity movement,Expectation maximization and binary clustering,First-passage time,Foraging,GPS telemetry,Hidden Markov models,K-means,Path segmentation
PDF
View via Publisher
AI Read Science
AI Summary
AI Summary is the key point extracted automatically understanding the full text of the paper, including the background, methods, results, conclusions, icons and other key content, so that you can get the outline of the paper at a glance.
Example
Background
Key content
Introduction
Methods
Results
Related work
Fund
Key content
- Pretraining has recently greatly promoted the development of natural language processing (NLP)
- We show that M6 outperforms the baselines in multimodal downstream tasks, and the large M6 with 10 parameters can reach a better performance
- We propose a method called M6 that is able to process information of multiple modalities and perform both single-modal and cross-modal understanding and generation
- The model is scaled to large model with 10 billion parameters with sophisticated deployment, and the 10 -parameter M6-large is the largest pretrained model in Chinese
- Experimental results show that our proposed M6 outperforms the baseline in a number of downstream tasks concerning both single modality and multiple modalities We will continue the pretraining of extremely large models by increasing data to explore the limit of its performance
Try using models to generate summary,it takes about 60s
Must-Reading Tree
Example
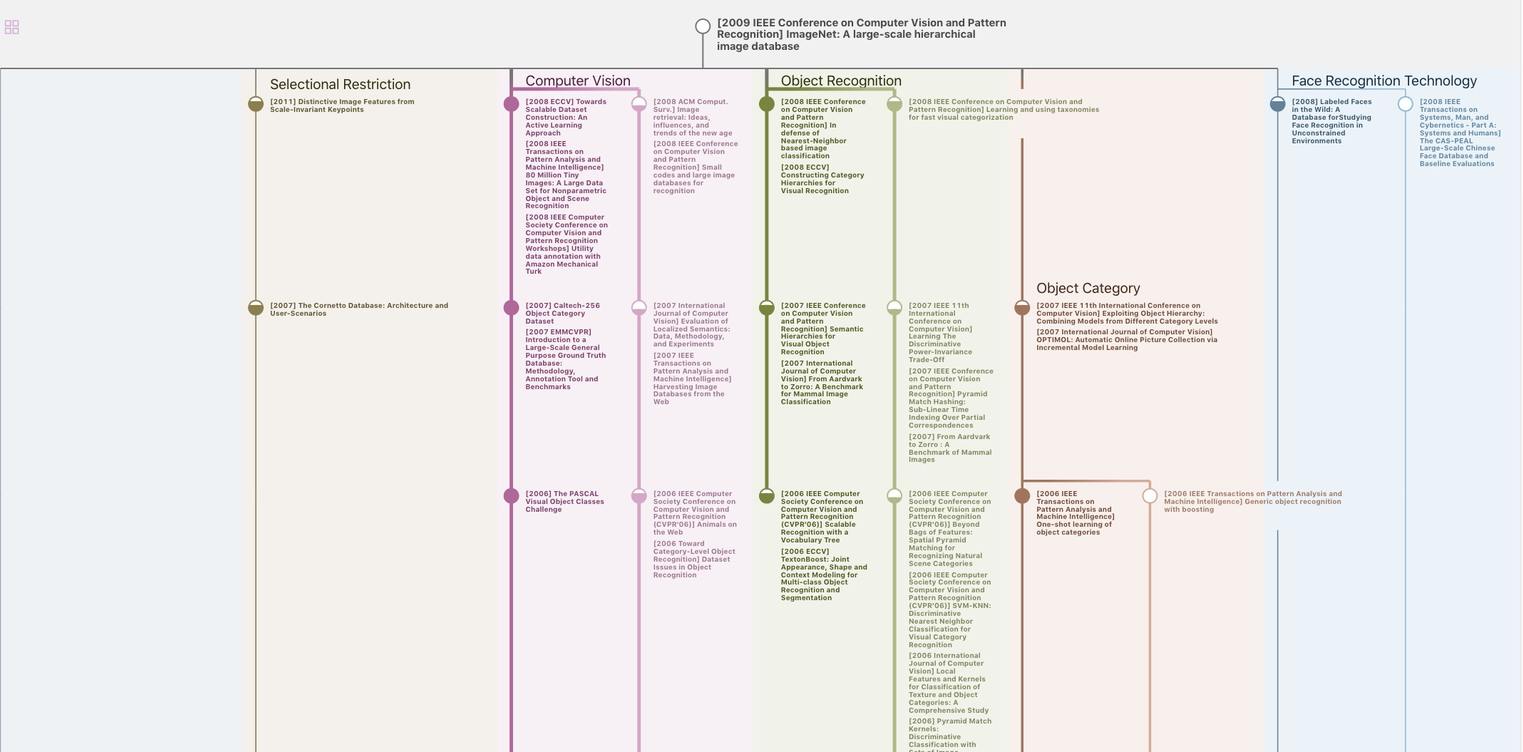
Generate MRT to find the research sequence of this paper
Related Papers
2007
被引用290 | 浏览
2004
被引用638 | 浏览
2010
被引用52 | 浏览
2008
被引用101 | 浏览
1995
被引用280 | 浏览
2008
被引用137 | 浏览
2013
被引用351 | 浏览
2014
被引用74 | 浏览
2015
被引用180 | 浏览
2016
被引用220 | 浏览
2013
被引用29 | 浏览
2015
被引用56 | 浏览
2017
被引用44 | 浏览
Data Disclaimer
The page data are from open Internet sources, cooperative publishers and automatic analysis results through AI technology. We do not make any commitments and guarantees for the validity, accuracy, correctness, reliability, completeness and timeliness of the page data. If you have any questions, please contact us by email: report@aminer.cn
Chat Paper
去 AI 文献库 对话