Dual Learning-Based Siamese Framework For Change Detection Using Bi-Temporal Vhr Optical Remote Sensing Images
REMOTE SENSING(2019)
摘要
As a fundamental and profound task in remote sensing, change detection from very-high-resolution (VHR) images plays a vital role in a wide range of applications and attracts considerable attention. Current methods generally focus on the research of simultaneously modeling and discriminating the changed and unchanged features. In practice, for bi-temporal VHR optical remote sensing images, the temporal spectral variability tends to exist in all bands throughout the entire paired images, making it difficult to distinguish none-changes and changes with a single model. In this paper, motivated by this observation, we propose a novel hybrid end-to-end framework named dual learning-based Siamese framework (DLSF) for change detection. The framework comprises two parallel streams which are dual learning-based domain transfer and Siamese-based change decision. The former stream is aimed at reducing the domain differences of two paired images and retaining the intrinsic information by translating them into each other's domain. While the latter stream is aimed at learning a decision strategy to decide the changes in two domains, respectively. By training our proposed framework with certain change map references, this method learns a cross-domain translation in order to suppress the differences of unchanged regions and highlight the differences of changed regions in two domains, respectively, then focus on the detection of changed regions. To the best of our knowledge, the idea of incorporating dual learning framework and Siamese network for change detection is novel. The experimental results on two datasets and the comparison with other state-of-the-art methods verify the efficiency and superiority of our proposed DLSF.
更多查看译文
关键词
VHR optical remote sensing images,change detection,deep learning technology,dual learning framework,Siamese network
AI 理解论文
溯源树
样例
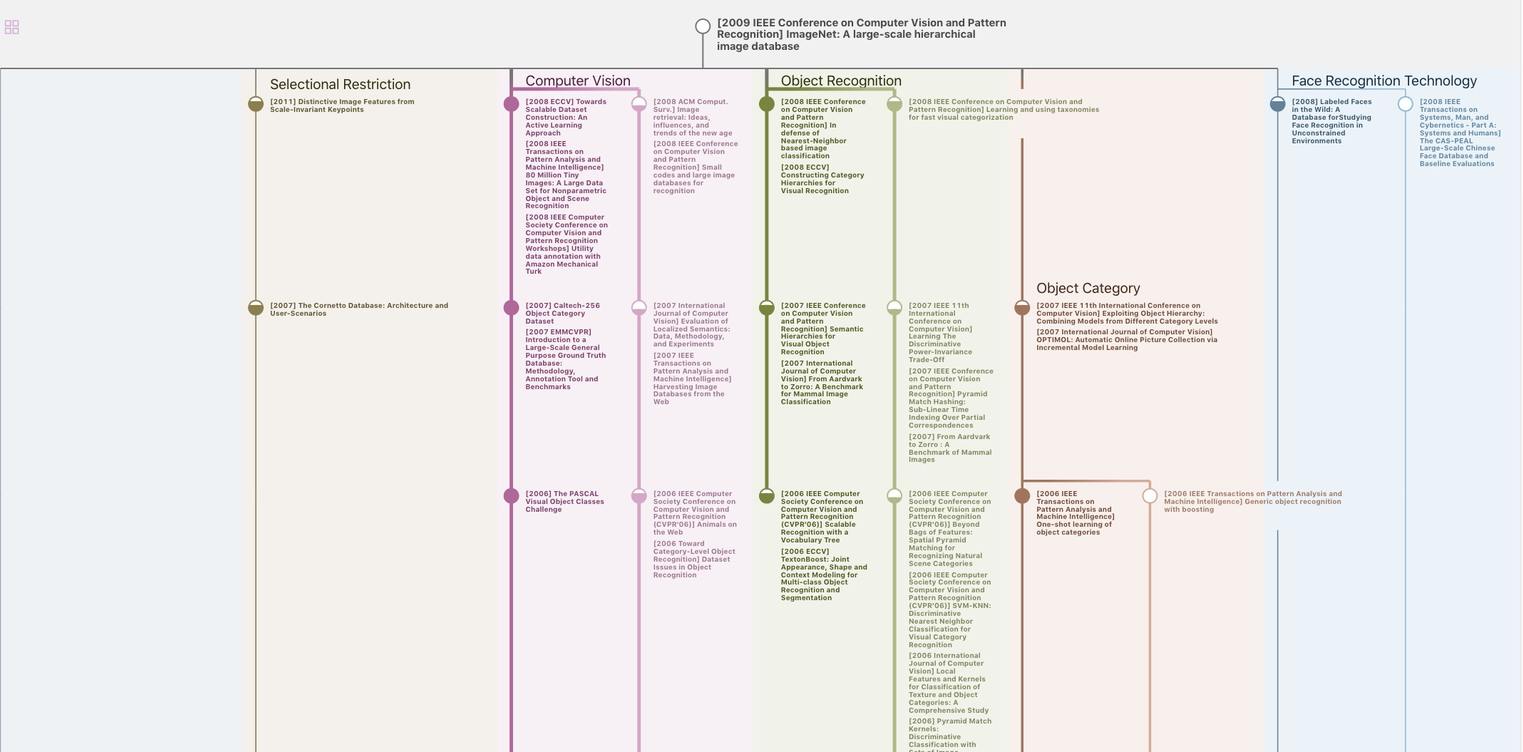
生成溯源树,研究论文发展脉络
Chat Paper
正在生成论文摘要