Machine Learning to Identify Predictors of Glycemic Control in Type 2 Diabetes: An Analysis of Target HbA1c Reduction Using Empagliflozin/Linagliptin Data
Pharmaceutical Medicine(2019)
Abstract
Introduction Outcomes in type 2 diabetes mellitus (T2DM) could be optimized by identifying which treatments are likely to produce the greatest improvements in glycemic control for each patient. Objectives We aimed to identify patient characteristics associated with achieving and maintaining a target glycated hemoglobin (HbA1c) of ≤ 7% using machine learning methodology to analyze clinical trial data on combination therapy for T2DM. By applying a new machine learning methodology to an existing clinical dataset, the practical application of this approach was evaluated and the potential utility of this new approach to clinical decision making was assessed. Methods Data were pooled from two phase III, randomized, double-blind, parallel-group studies of empagliflozin/linagliptin single-pill combination therapy versus each monotherapy in patients who were treatment-naïve or receiving background metformin. Descriptive analysis was used to assess univariate associations between HbA1c target categories and each baseline characteristic. After the descriptive analysis results, a machine learning analysis was performed (classification tree and random forest methods) to estimate and predict target categories based on patient characteristics at baseline, without a priori selection. Results In the descriptive analysis, lower mean baseline HbA1c and fasting plasma glucose (FPG) were both associated with achieving and maintaining the HbA1c target. The machine learning analysis also identified HbA1c and FPG as the strongest predictors of attaining glycemic control. In contrast, covariates including body weight, waist circumference, blood pressure, or other variables did not contribute to the outcome. Conclusions Using both traditional and novel data analysis methodologies, this study identified baseline glycemic status as the strongest predictor of target glycemic control attainment. Machine learning algorithms provide an hypothesis-free, unbiased methodology, which can greatly enhance the search for predictors of therapeutic success in T2DM. The approach used in the present analysis provides an example of how a machine learning algorithm can be applied to a clinical dataset and used to develop predictions that can facilitate clinical decision making.
MoreTranslated text
Key words
glycemic control,target hba1c reduction,diabetes,machine learning,empagliflozin/linagliptin
AI Read Science
Must-Reading Tree
Example
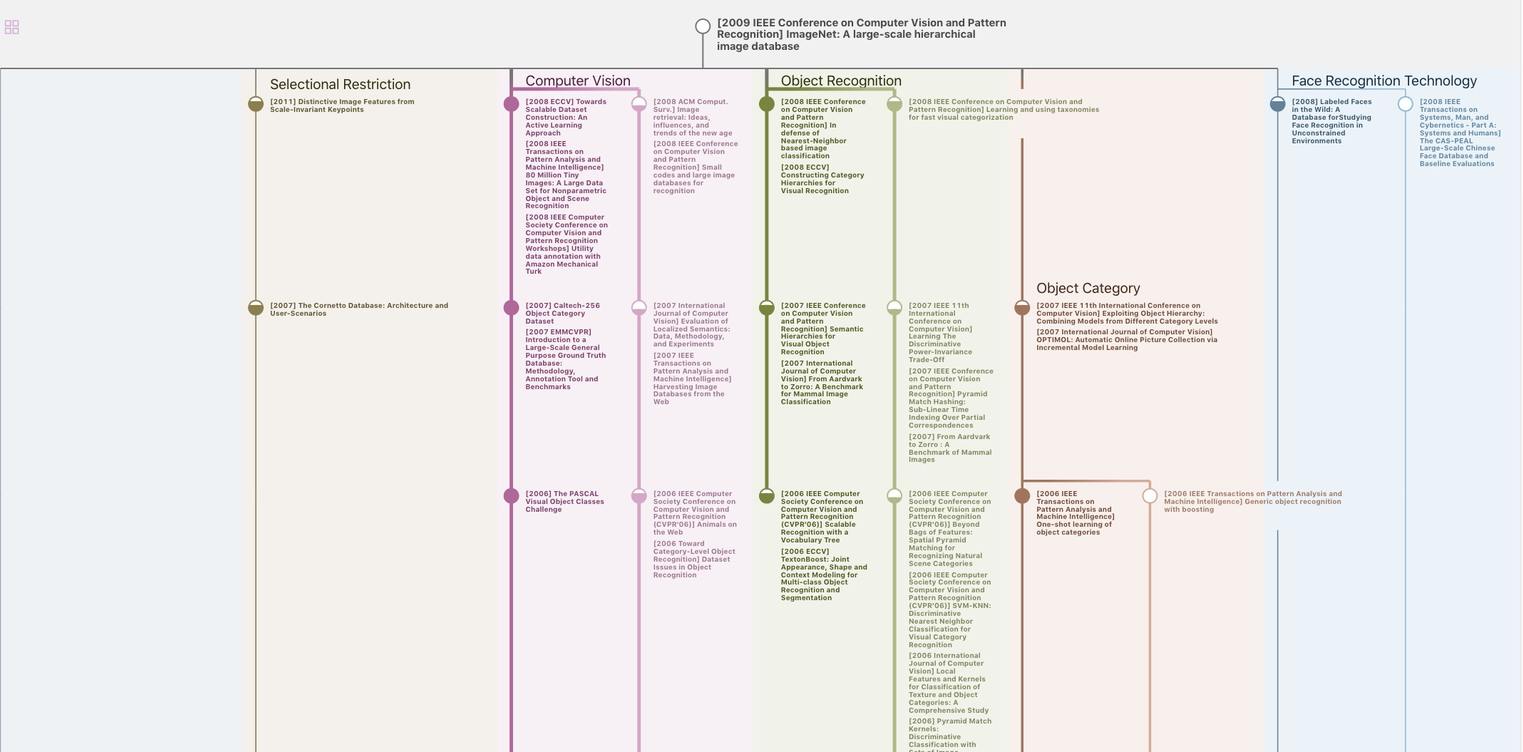
Generate MRT to find the research sequence of this paper
Chat Paper
Summary is being generated by the instructions you defined