Leveraging implicit knowledge in neural networks for functional dissection and engineering of proteins
NATURE MACHINE INTELLIGENCE(2019)
摘要
Proteins are nature’s most versatile molecular machines. Deep neural networks trained on large protein datasets have recently been used to tackle the unmet complexity of protein sequence–function relationships. The implicit knowledge contained in these networks represents a powerful, but thus far inaccessible, resource for understanding protein biology. Here, we show that occlusion-based sensitivity analysis can leverage the knowledge present in deep-neural-network-based protein sequence classifiers to identify functionally relevant parts of proteins. We first validated our approach by successfully predicting positions that mediate small molecule binding or catalytic activity across different protein classes. Next, we inferred the impact of point mutations on the activity of ERK and HRas, signalling factors frequently deregulated in cancer. Finally, we used our approach to identify engineering hotspots in CRISPR–Cas9 and anti-CRISPR protein AcrIIA4. Our work demonstrates how implicit knowledge in neural networks can be harnessed for protein functional dissection and protein engineering.
更多查看译文
关键词
Gene ontology,Machine learning,Protein analysis,Protein design,Protein function predictions,Engineering,general
AI 理解论文
溯源树
样例
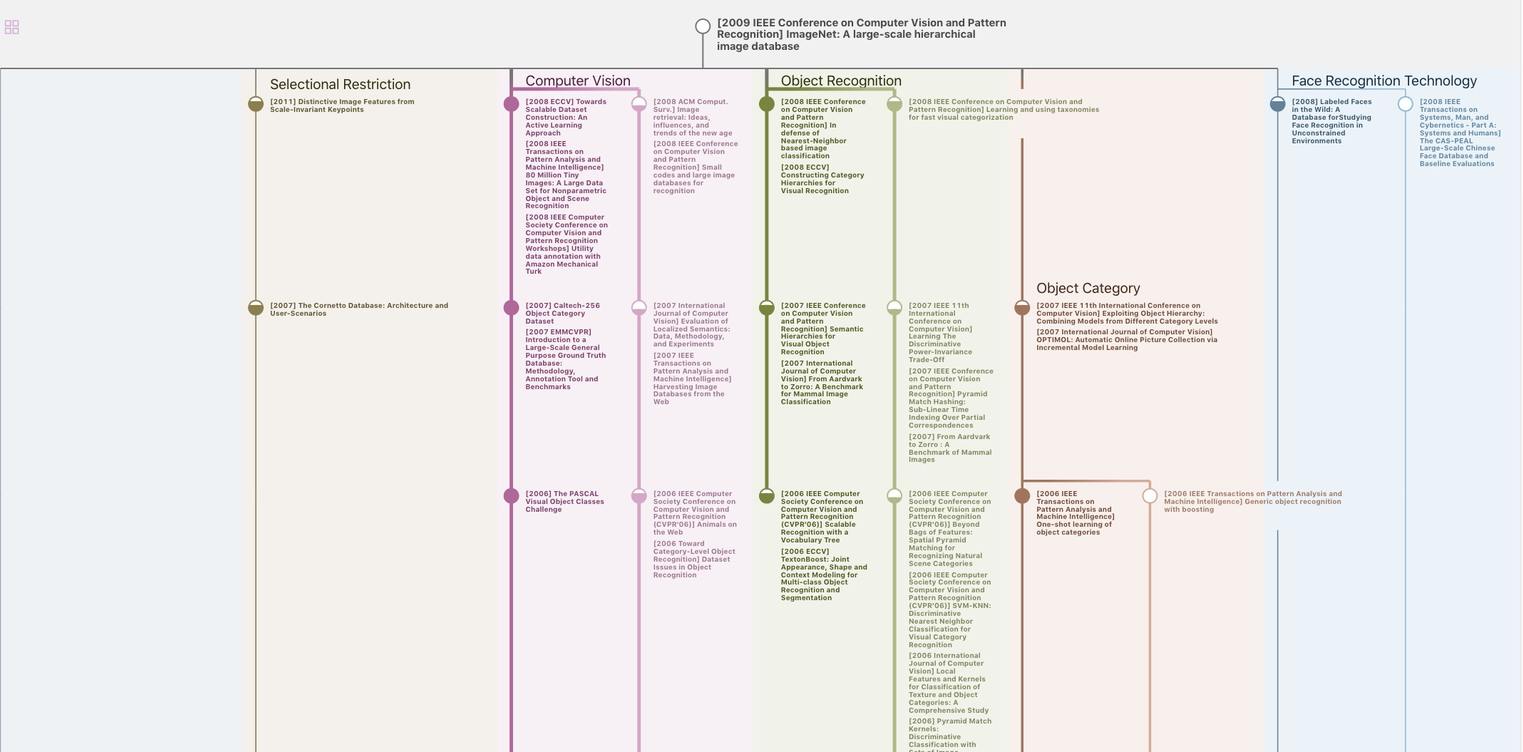
生成溯源树,研究论文发展脉络
Chat Paper
正在生成论文摘要