Reversible Addition-Fragmentation Chain Transfer Polymerization of 2-Chloroethyl Methacrylate and Post-Polymerization Modification
Macromolecular Research(2019)SCI 4区
Beijing Advanced Innovation Center for Soft Matter Science and Engineering | Department of Chemistry | School of Materials Science and Engineering
Abstract
An alkyl halide containing monomer, 2-chloroethyl methacrylate (CEMA) was synthesized via the chorination of 2-hydroethyl methacryalte and polymerized by reversible addition-fragmentation chain transfer (RAFT) polymerization. The kinetics of the controlled/living radical polyemrization (CRP) was systematically investigated. The chain end livingness of poly(2-chloroethyl methacrylate) (PCEMA) was confirmed by the chain extension with methyl methacrylate (MMA) under RAFT polymerization conditions. PCEMA with dangling alkyl chloride groups was directly azidated through a nucleophilic substitution with sodium azide, affording a polymer with an azido group at each repeating unit. The resulting polymer was readily available for post-polymerization modifications by various click reactions. These strategies may open new perspectives toward more effective and milder conditions for azide involving reactions.
MoreTranslated text
Key words
alkyl halide,RAFT polymerization,azidation,post-polymerization modification,click chemistry
PDF
View via Publisher
AI Read Science
AI Summary
AI Summary is the key point extracted automatically understanding the full text of the paper, including the background, methods, results, conclusions, icons and other key content, so that you can get the outline of the paper at a glance.
Example
Background
Key content
Introduction
Methods
Results
Related work
Fund
Key content
- Pretraining has recently greatly promoted the development of natural language processing (NLP)
- We show that M6 outperforms the baselines in multimodal downstream tasks, and the large M6 with 10 parameters can reach a better performance
- We propose a method called M6 that is able to process information of multiple modalities and perform both single-modal and cross-modal understanding and generation
- The model is scaled to large model with 10 billion parameters with sophisticated deployment, and the 10 -parameter M6-large is the largest pretrained model in Chinese
- Experimental results show that our proposed M6 outperforms the baseline in a number of downstream tasks concerning both single modality and multiple modalities We will continue the pretraining of extremely large models by increasing data to explore the limit of its performance
Try using models to generate summary,it takes about 60s
Must-Reading Tree
Example
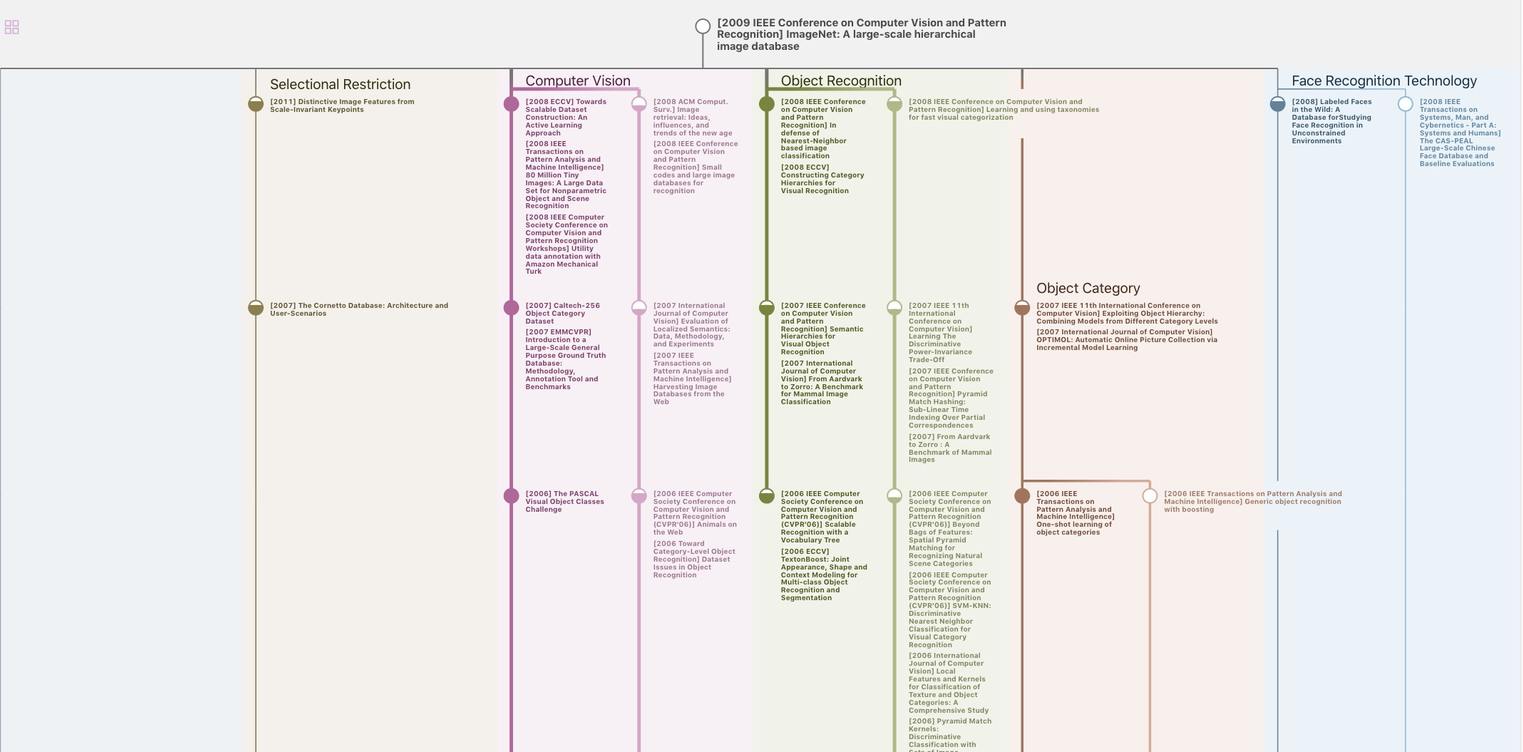
Generate MRT to find the research sequence of this paper
Related Papers
Data Disclaimer
The page data are from open Internet sources, cooperative publishers and automatic analysis results through AI technology. We do not make any commitments and guarantees for the validity, accuracy, correctness, reliability, completeness and timeliness of the page data. If you have any questions, please contact us by email: report@aminer.cn
Chat Paper
GPU is busy, summary generation fails
Rerequest