Fast Graph Centrality Computation Via Sampling: a Case Study of Influence Maximisation over OSNs.
International journal of high performance computing and networking(2019)
摘要
Graph centrality computation, e.g., asking for the most important vertices in a graph, may incur a high time cost with the increasing size of graphs. To address this challenge, this paper presents a sampling-based framework to speed up the computation of graph centrality. As a use case, we study the problem of influence maximisation (IM), which asks for the k most influential nodes in a graph to trigger the largest influence spread, and present an IM-RWS algorithm. We experimentally compare IM-RWS with the state-of-the-art influence maximisation algorithms IMM and IM-RW, and the results show that our solution can bring a significant improvement in efficiency as well as a certain extent of improvement in empirical accuracy. In particular, our algorithm can solve the influence maximisation problem in graphs containing millions of nodes within tens of seconds with an even better performance result in terms of influence spread.
更多查看译文
关键词
random walk,sampling,graph centrality,online social networks,influence maximisation
AI 理解论文
溯源树
样例
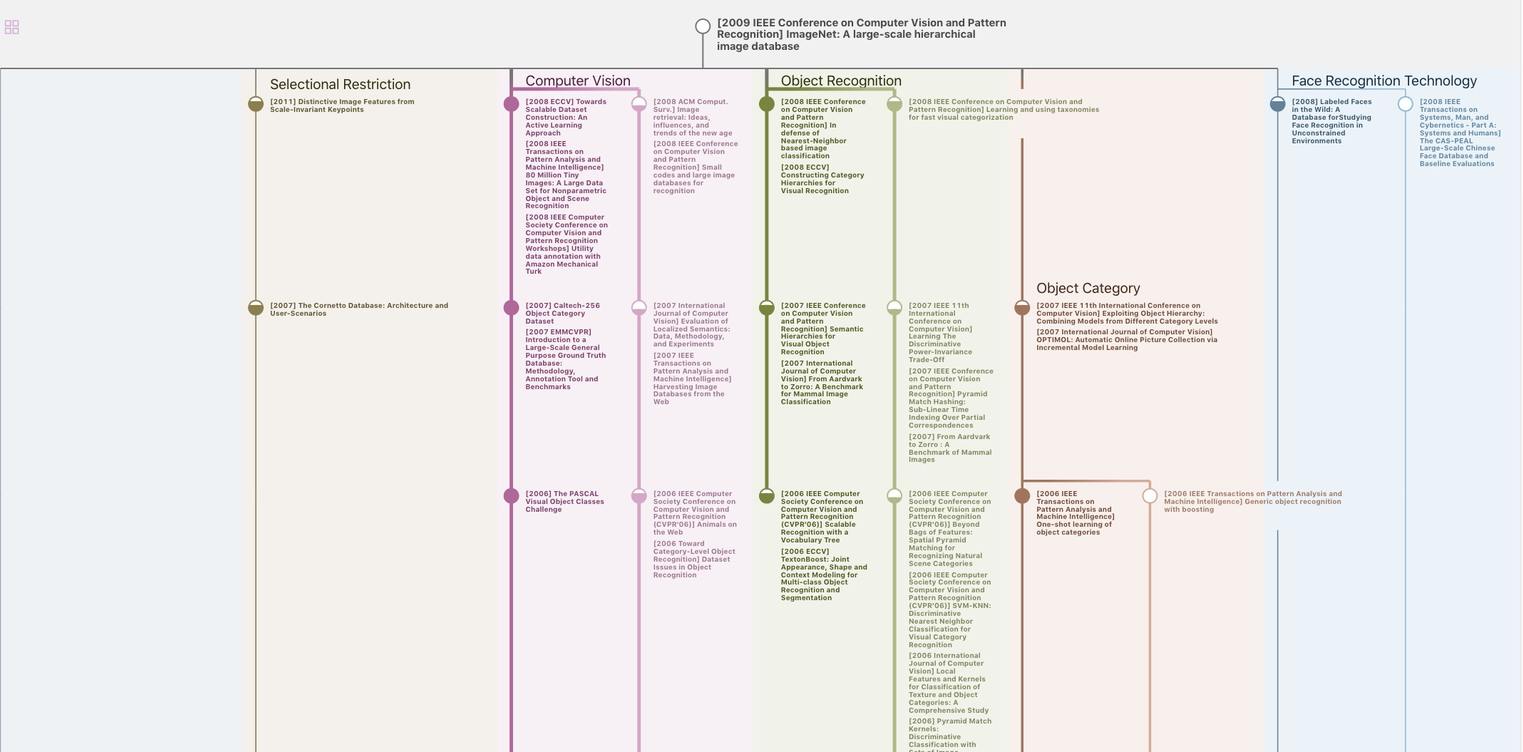
生成溯源树,研究论文发展脉络
Chat Paper
正在生成论文摘要