Fully Convolutional Network Method Of Semantic Segmentation Of Class Imbalance Remote Sensing Images
ACTA OPTICA SINICA(2019)
摘要
A fully convolutional network (FCN) model based on U-Net is proposed to implement the semantic segmentation of remote sensing images with high resolution, in which the data standardization and data augmentation arc adopted for data preprocessing. In addition, the Adam optimizer is used for the model training and the average Jaccard index is used as the evaluation metric. A weighted cross entropy loss function and an adaptive threshold algorithm arc employed to improve the classification accuracy of small classes. The experimental results on the DSTI, dataset show that the proposed method can increase the average Jaccard index of prediction results from 0.611 to 0.636, and produces an accurate end-to-end classification for high-resolution remote sensing images.
更多查看译文
关键词
image processing, remote sensing images, semantic segmentation, class imbalance, fully convolutional network, deep learning
AI 理解论文
溯源树
样例
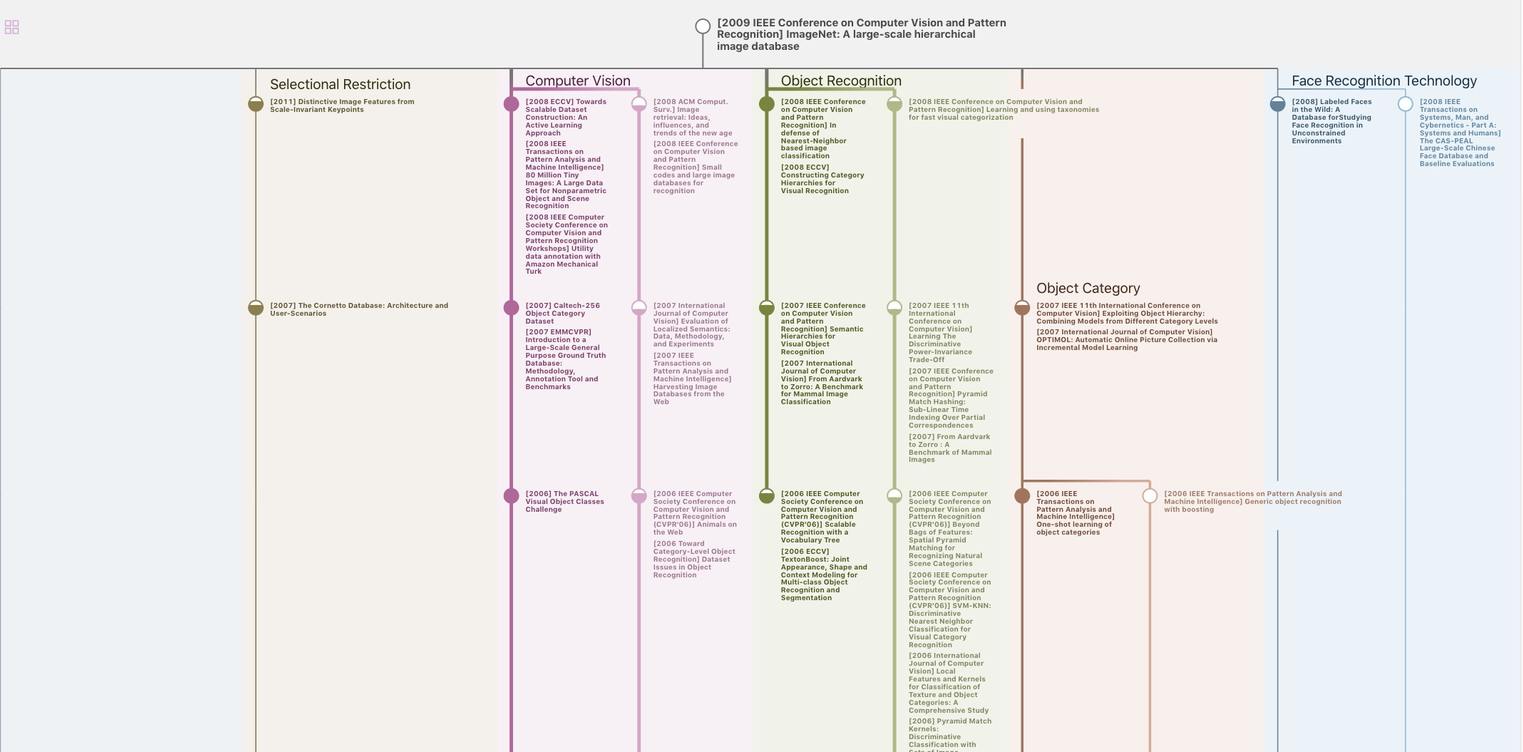
生成溯源树,研究论文发展脉络
Chat Paper
正在生成论文摘要