A Compact Framework For Voice Conversion Using Wavenet Conditioned On Phonetic Posteriorgrams
2019 IEEE INTERNATIONAL CONFERENCE ON ACOUSTICS, SPEECH AND SIGNAL PROCESSING (ICASSP)(2019)
摘要
Voice conversion can benefit from WaveNet vocoder with improvement in converted speech's naturalness and quality. However, nowadays approaches segregate the training of conversion module and WaveNet vocoder towards different optimization objectives, which might lead to the difficulty in model tuning and coordination. In this paper, we propose a compact framework to unify the conversion and the vocoder parts. Multi-head self-attention structure and bidirectional long short-term memory (BLSTM) recurrent neural network (RNN) are employed to encode speaker independent phonetic posteriorgrams (PPGs) into an intermediate representation which is used as the condition input of WaveNet to generate target speaker's waveform. In this way, we unify the conversion and vocoder parts into a compact system in which all parameters can be tuned simultaneously for global optimization. We compared the proposed method with the baseline system that consists of separately trained conversion module and WaveNet vocoder. Subjective evaluations show that the proposed method can achieve better results in both naturalness and speaker similarity.
更多查看译文
关键词
Voice conversion, WaveNet, phonetic posteriorgrams(PPGs), self-attention, BLSTM
AI 理解论文
溯源树
样例
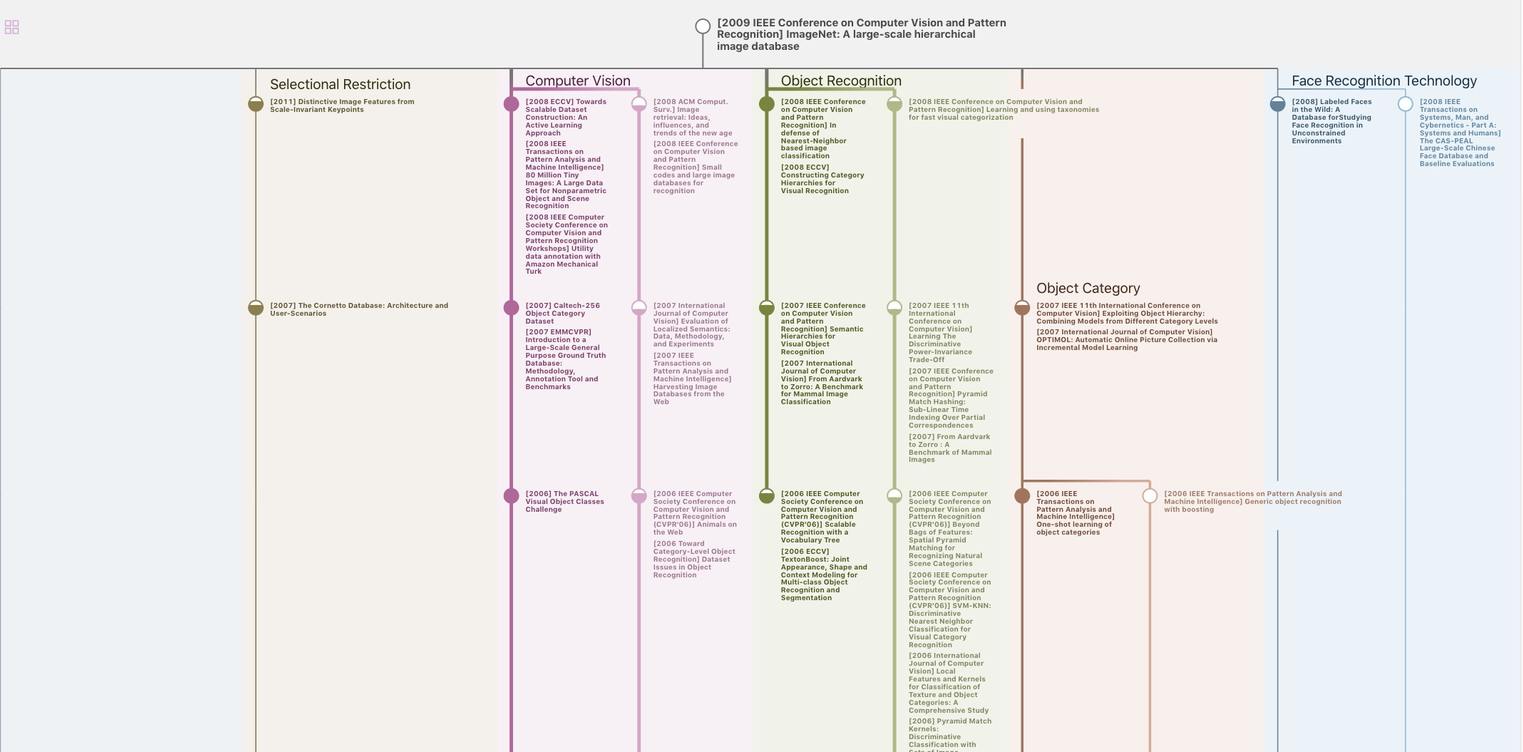
生成溯源树,研究论文发展脉络
Chat Paper
正在生成论文摘要