Machine Learning–based Analysis of In-Cylinder Flow Fields to Predict Combustion Engine Performance
International journal of engine research(2019)
摘要
Cycle-to-cycle variations in an optically accessible four-stroke direct injection spark-ignition gasoline engine are investigated using high-speed scanning particle image velocimetry and in-cylinder pressure measurements. Particle image velocimetry allows to measure in-cylinder flow fields at high spatial and temporal resolution. Binary classifiers are used to predict combustion cycles of high indicated mean effective pressure based on in-cylinder flow features and engineered tumble features obtained during the intake and the compression stroke. Basic in-cylinder flow features of the mid-cylinder plane are sufficient to predict combustion cycles of high indicated mean effective pressure as early as 180 degree crank angle before the top dead center at 0 degree crank angle. Engineered characteristic tumble features derived from the flow field are not superior to the basic flow features. The results are independent of the tested machine learning method (multilayer perceptron and boosted decision trees) and robust to hyper-parameter selection.
更多查看译文
关键词
Gasoline combustion engine,cycle-to-cycle variations,high-speed scanning particle image velocimetry,binary classifier,feature importance,neural network
AI 理解论文
溯源树
样例
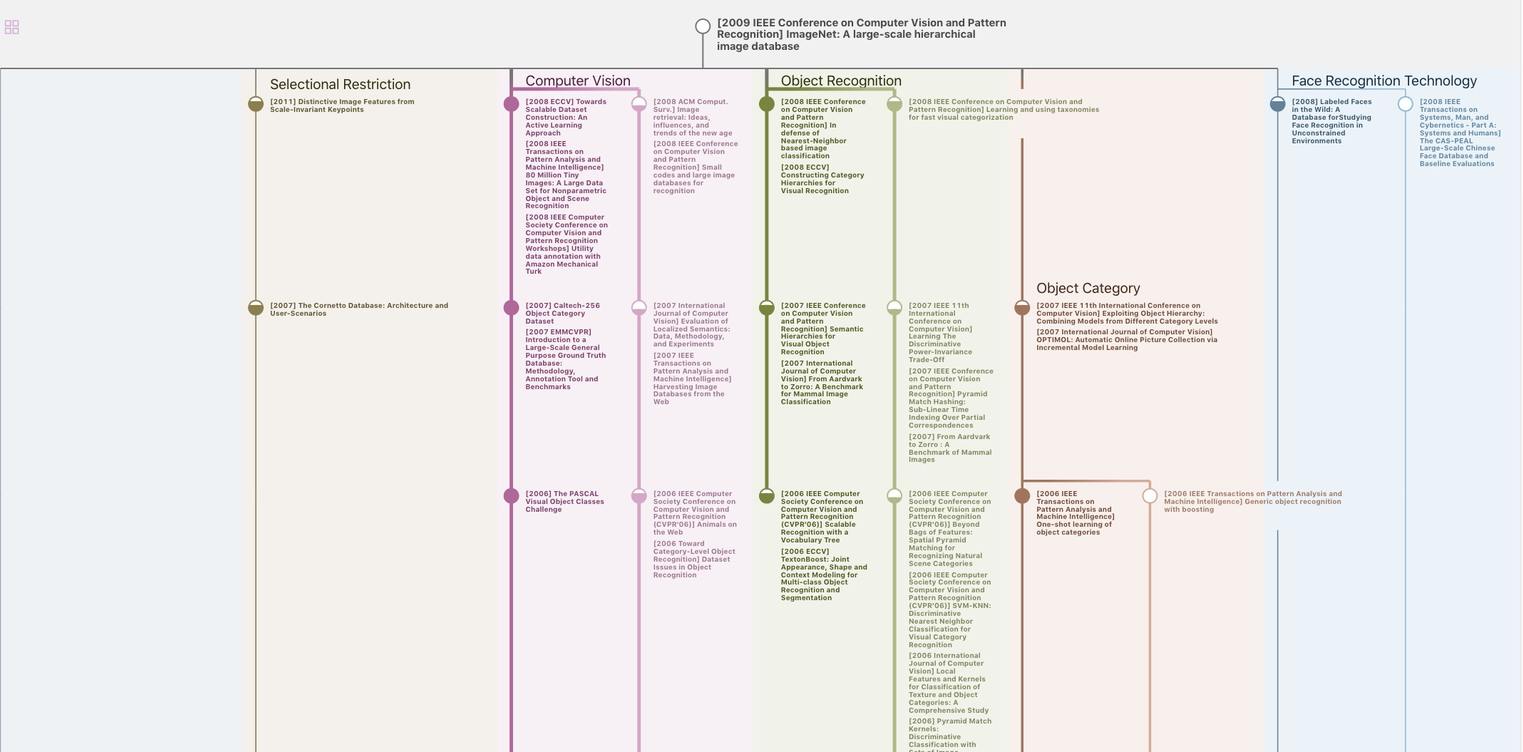
生成溯源树,研究论文发展脉络
Chat Paper
正在生成论文摘要