Accelerated Scale Bridging with Sparsely Approximated Gaussian Learning.
Journal of computational physics(2020)
摘要
Multiscale modeling is a systematic approach to describe the behavior of complex systems by coupling models from different scales. The approach has been demonstrated to be very effective in areas of science as diverse as materials science, climate modeling and chemistry. However, routine use of multiscale simulations is often hindered by the very high cost of individual at-scale models. Approaches aiming to alleviate that cost by means of Gaussian process regression based surrogate models have been proposed. Yet, many of these surrogate models are expensive to construct, especially when the number of data needed is large. In this article, we employ a hierarchical sparse Cholesky decomposition to develop a sparse Gaussian process regression method and apply the method to approximate the equation of state of a material in a multiscale model of dynamic deformation. We demonstrate that the method provides a substantial reduction both in computational cost and solution error as compared with previous methods.
更多查看译文
关键词
Multiscale modeling,Gaussian regression,Scale bridging,Sparse Cholesky decomposition
AI 理解论文
溯源树
样例
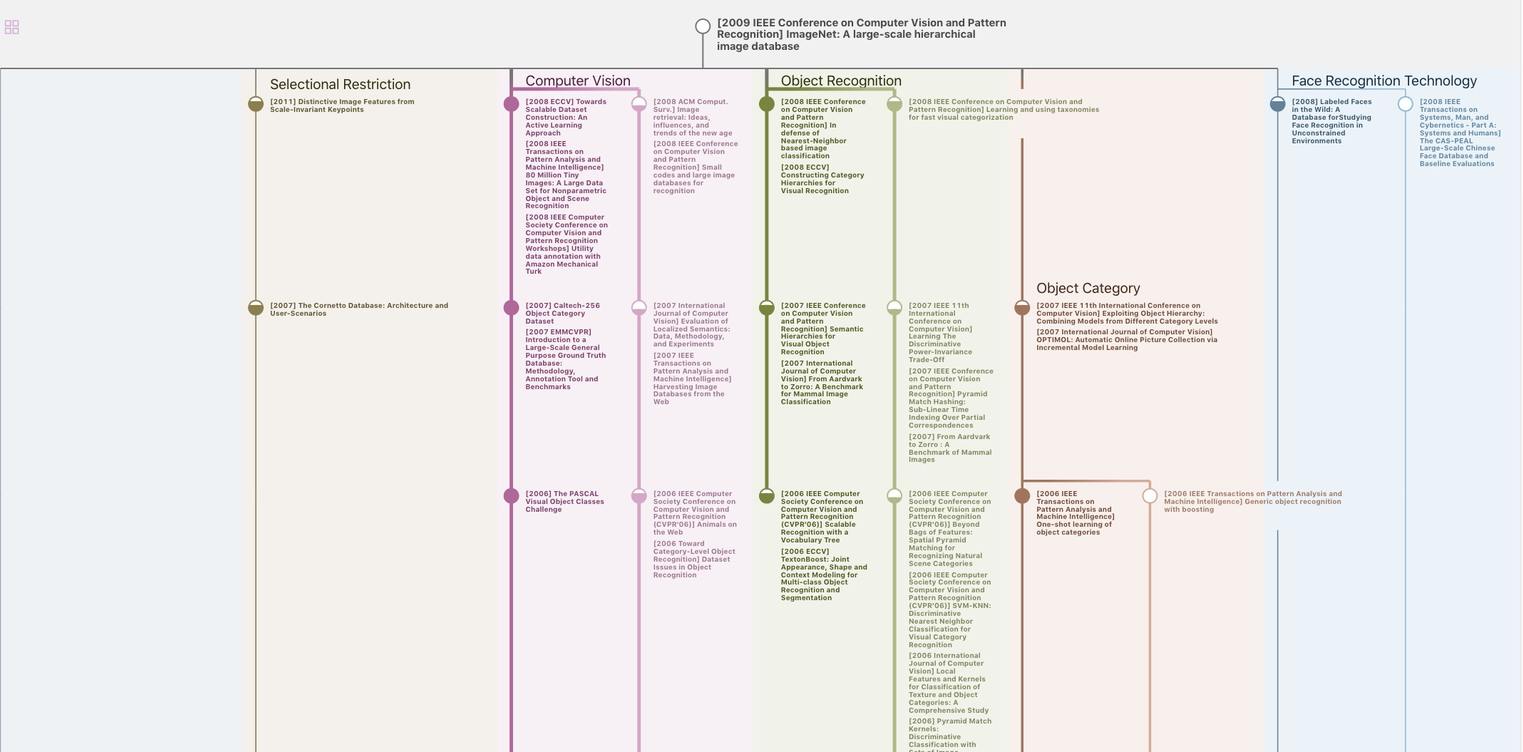
生成溯源树,研究论文发展脉络
Chat Paper
正在生成论文摘要