Predicting the Electrochemical Properties of Lithium-Ion Battery Electrode Materials with the Quantum Neural Network Algorithm
The Journal of Physical Chemistry C(2019)
Sejong Univ | Sunchon Natl Univ
Abstract
Discovery of new inorganic solid materials can be accelerated with the aid of a reliable computational tool for predicting the associated electrochemical properties. Hence, we propose a quantitative structure-property relationship model by combining the three-dimensional (3D) quantum mechanical descriptors of materials and the artificial neural network algorithm, which is termed the 3D-QANN model. 3D distribution of electrostatic potentials (ESPs) in the super cell of each inorganic solid material serves as the unique numerical descriptor to derive the 3D-QANN model. The optimized prediction model is then validated in terms of estimating the discharge energy density (D) and the capacity fading (Q) of lithium-ion battery (LIB) cathode materials with the layered structure. The 3D-QANN model reveals good performance in predicting both D and Q values with high correlation with the corresponding experimental data. This indicates the suitability of the quantum mechanical ESP distribution as the numerical descriptor for LIB cathode materials. Because of the simplicity in model building and the high predictive capability, the 3D-QANN model is anticipated to serve as a useful computational tool for estimating the electrochemical properties and accordingly for designing new materials.
MoreTranslated text
PDF
View via Publisher
AI Read Science
Must-Reading Tree
Example
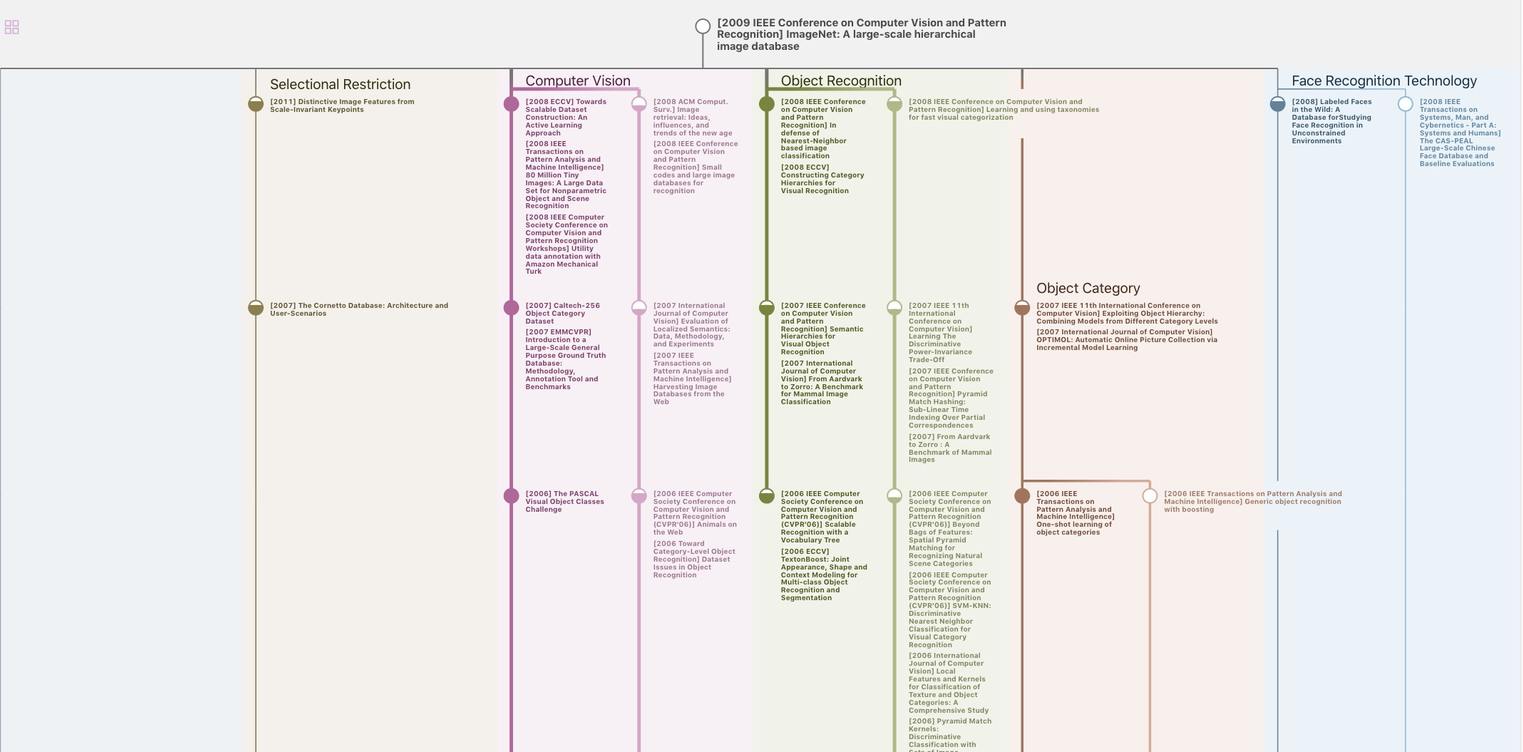
Generate MRT to find the research sequence of this paper
Related Papers
Physical review B/Physical review B 2019
被引用38
The Journal of Physical Chemistry C 2020
被引用19
Structure and Ion Conductivity Study of Argyrodite (li5.5ps4.5cl1.5) According to Cooling Method
Korean Journal of Metals and Materials 2021
被引用5
Journal of Materials Chemistry A 2023
被引用5
AIN SHAMS ENGINEERING JOURNAL 2024
被引用4
Data Disclaimer
The page data are from open Internet sources, cooperative publishers and automatic analysis results through AI technology. We do not make any commitments and guarantees for the validity, accuracy, correctness, reliability, completeness and timeliness of the page data. If you have any questions, please contact us by email: report@aminer.cn
Chat Paper
去 AI 文献库 对话