A New Variable Selection Method Based on SVM for Analyzing Supersaturated Designs
Journal of quality technology(2019)
摘要
Supersaturated designs (SSDs) are designs whose factors exceeds run size; thus, there are not enough runs for estimating all the main effects. They are commonly used in screening experiments, where the primary goal is to identify the few, but dominant, active factors, keeping the cost as low as possible. The development of new statistical methods inspired by machine learning algorithms is increasing rapidly, especially nowadays. One of such methods is the support vector machine recursive feature elimination (SVM-RFE), which manages to extract the informative genes in classification problems, while it achieves extremely high performance. In this article, we study a variable selection method for regression problems, called SVR-RFE, to screen active effects in both two-level and mixed-level designs. Simulation studies demonstrate that this procedure is effective enough, especially in terms of statistical power.
更多查看译文
关键词
linear models,recursive feature elimination,supersaturated designs,support vector machines,SVR-RFE
AI 理解论文
溯源树
样例
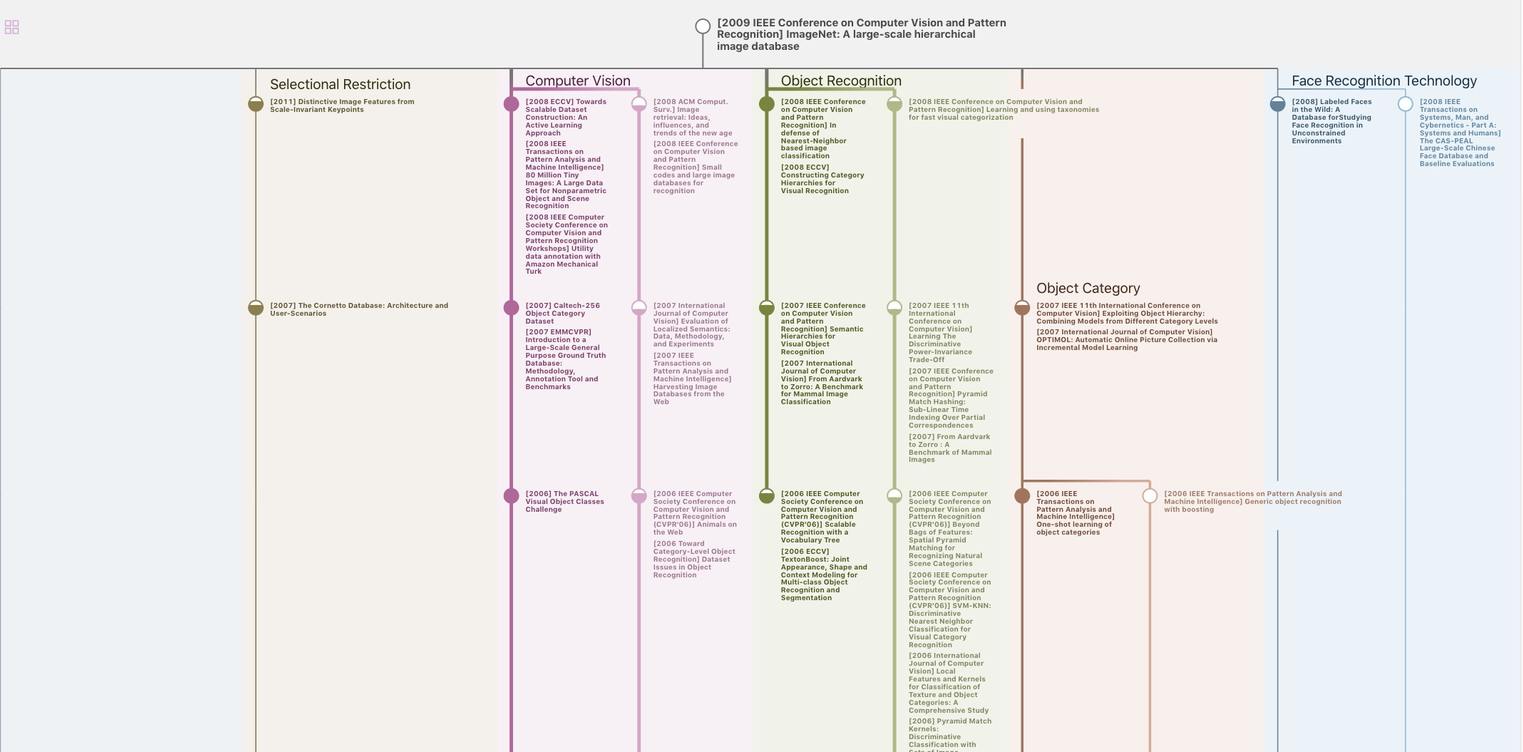
生成溯源树,研究论文发展脉络
Chat Paper
正在生成论文摘要