Estimating Prevalence Using Indirect Information And Bayesian Evidence Synthesis
CANADIAN JOURNAL OF STATISTICS-REVUE CANADIENNE DE STATISTIQUE(2018)
摘要
We focus on the analysis of health count data, aggregated over disjoint geographical locations, by combining information from data sources in a coherent fashion using a Bayesian hierarchical model. The overall objective is to estimate prevalence of a medical condition in the population given that the sampled counts arise from a subset of all cases, and when there is no additional information available from the data. We develop a hierarchical model to predict the overall prevalence using an external data set for calibration. We demonstrate that the Bayesian methodology can account fully for the uncertainty, variability and spatial dependence for the estimate. We apply our model to dispensing data obtained by the Public Health Agency of Canada for 2014, and assess the prevalence of treated Attention Deficit Hyperactivity Disorder (ADHD) from records of drugs dispensed to treat the condition. We demonstrate that our final model fits the data well in an out-of-sample assessment. We estimate the prevalence of treated ADHD in Canada to be 1.14% with 95% credible interval (0.86%, 1.27%), with prevalence noted to be higher in the eastern part of Canada, most notably in Nova Scotia. The Canadian Journal of Statistics 46: 673-689; 2018 (c) 2018 Societe statistique du Canada
更多查看译文
关键词
Attention Deficit Hyperactivity Disorder,Bayesian evidence synthesis,dispensing records,hierarchical models,prevalence,public health surveillance,spatial analysis
AI 理解论文
溯源树
样例
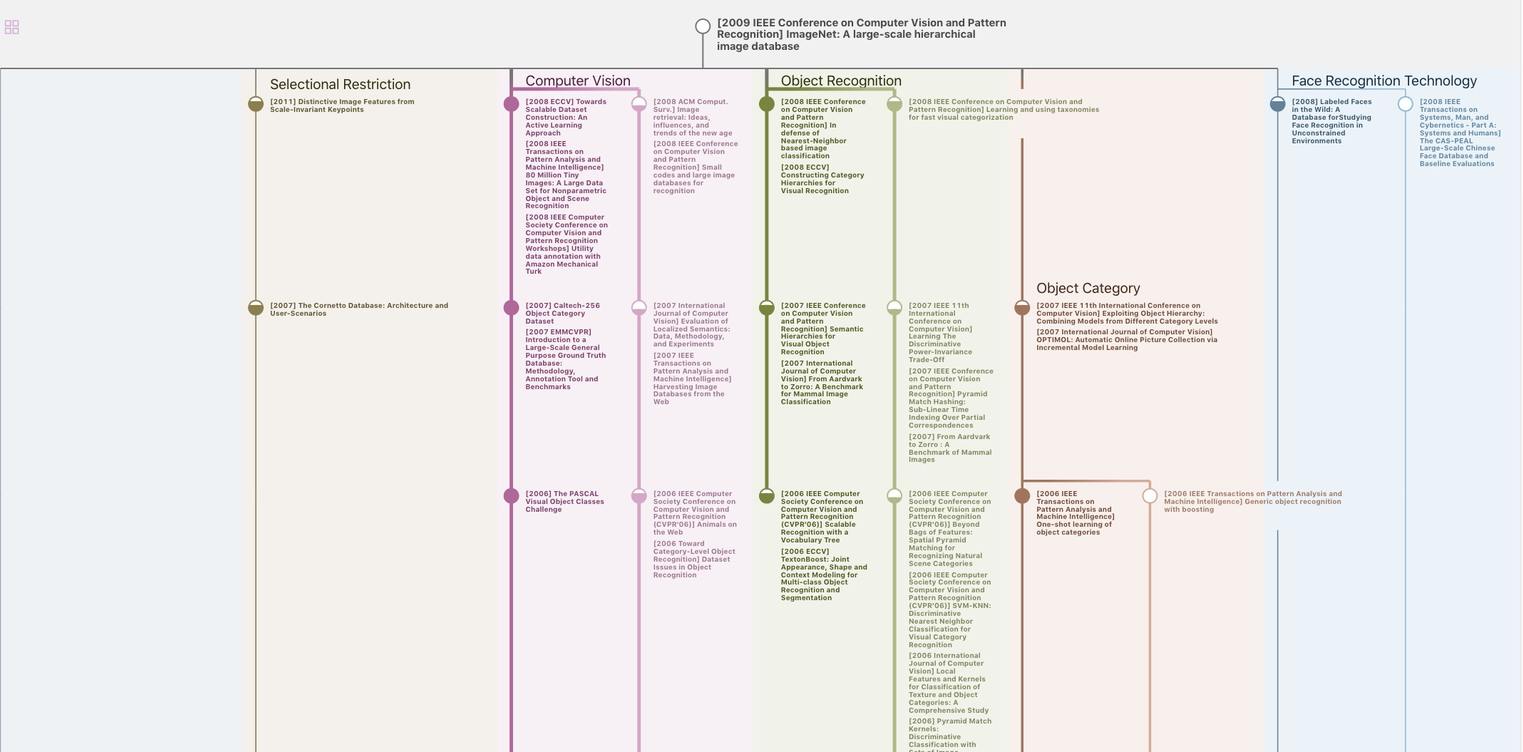
生成溯源树,研究论文发展脉络
Chat Paper
正在生成论文摘要