Mesoscopic population equations for spiking neural networks with synaptic short-term plasticity
The Journal of Mathematical Neuroscience(2020)
摘要
Coarse-graining microscopic models of biological neural networks to obtain mesoscopic models of neural activities is an essential step towards multi-scale models of the brain. Here, we extend a recent theory for mesoscopic population dynamics with static synapses to the case of dynamic synapses exhibiting short-term plasticity (STP). The extended theory offers an approximate mean-field dynamics for the synaptic input currents arising from populations of spiking neurons and synapses undergoing Tsodyks–Markram STP. The approximate mean-field dynamics accounts for both finite number of synapses and correlation between the two synaptic variables of the model (utilization and available resources) and its numerical implementation is simple. Comparisons with Monte Carlo simulations of the microscopic model show that in both feedforward and recurrent networks, the mesoscopic mean-field model accurately reproduces the first- and second-order statistics of the total synaptic input into a postsynaptic neuron and accounts for stochastic switches between Up and Down states and for population spikes. The extended mesoscopic population theory of spiking neural networks with STP may be useful for a systematic reduction of detailed biophysical models of cortical microcircuits to numerically efficient and mathematically tractable mean-field models.
更多查看译文
关键词
Short-term plasticity,Multi-scale modeling,Mesoscopic population dynamics
AI 理解论文
溯源树
样例
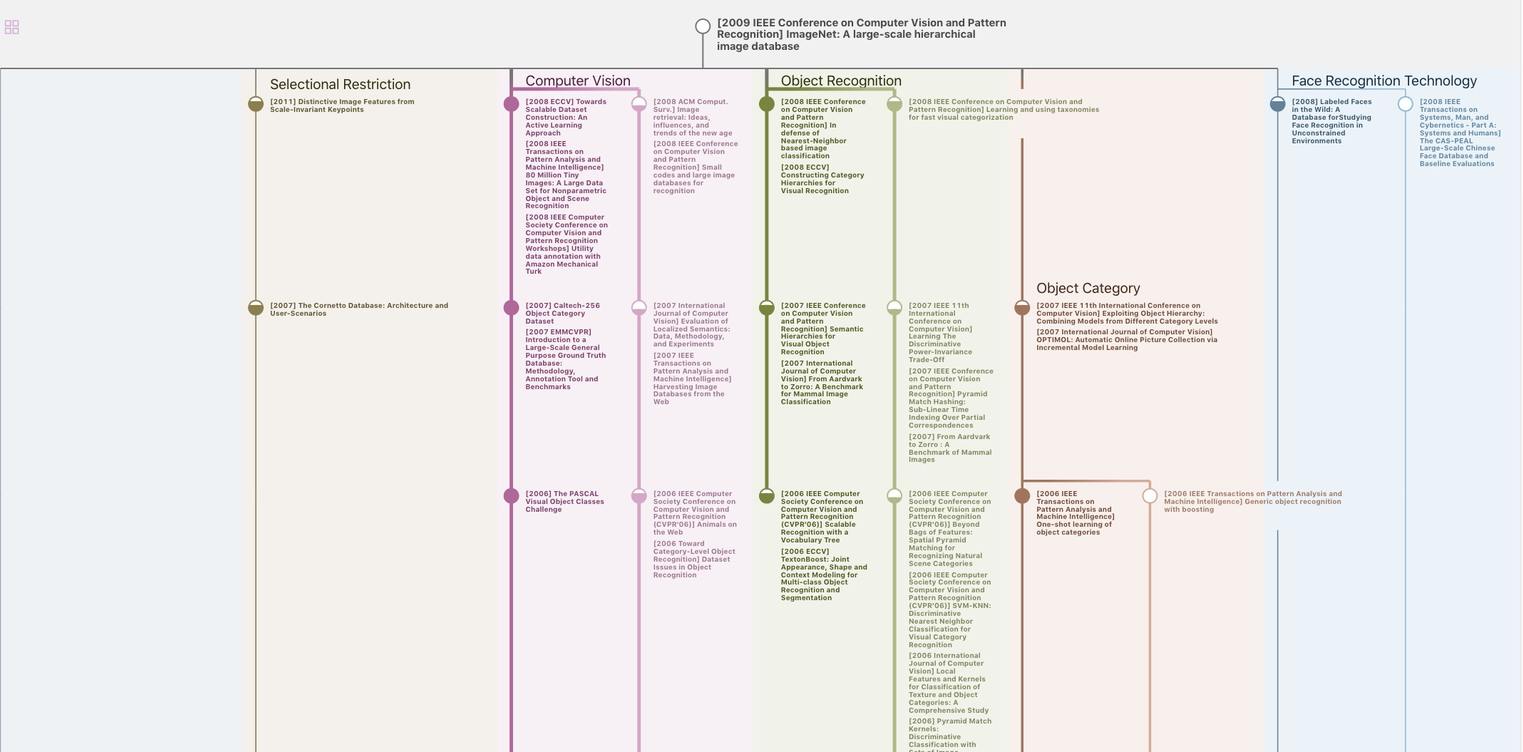
生成溯源树,研究论文发展脉络
Chat Paper
正在生成论文摘要