Plankton Image Classification Via Multi-Class Imbalanced Learning
2018 OCEANS - MTS/IEEE KOBE TECHNO-OCEANS (OTO)(2018)
摘要
The plankton is an incredibly large group of organisms. As a fatal component in the ocean, their distribution and richness can impact on the material cycle of the water ecosystem and the balance of ecological system. Thus it's a valuable work to detect and identify plankton. We will be able to alert against the red tide if our equipment can automatically identify them. The main deficiency is that data in the main plankton datasets are quite imbalanced, i. e. some species have more than 100,000 images while some others have only less than 10 images in one dataset. Imbalanced learning has been a research emphasis in recent years because of the growing number of class-imbalanced classification problems. The problem is particularly problematic when it comes to plankton classification. We proposed a new algorithm EasyEnsemble. D to overcome this deficiency. EasyEnsemble. D samples subsets from majority classes and classify them according to different weight. Then output the combination of each weak learner. The results show that as for ZooScan dataset, EasyEnsemble. D has higher F-measure and G-mean values than other imbalanced learning methods. It can be used in both dichotomy problems and multiple problems.
更多查看译文
关键词
Plankton Classification, Imbalanced Learning, Multi Class Learning, AdaBoost
AI 理解论文
溯源树
样例
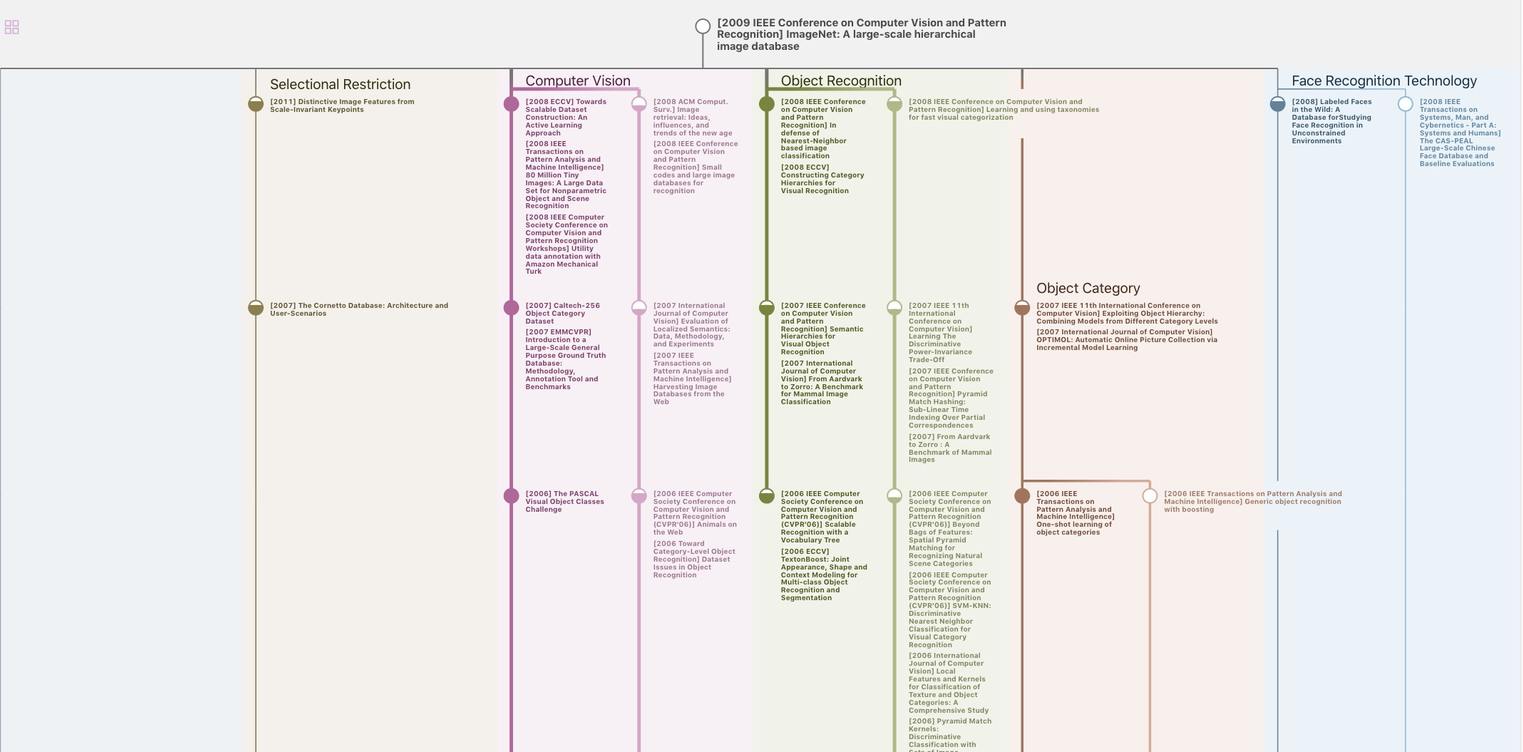
生成溯源树,研究论文发展脉络
Chat Paper
正在生成论文摘要