Semiconductor Parameter Extraction Via Current-Voltage Characterization and Bayesian Inference Methods
2018 IEEE 7th World Conference on Photovoltaic Energy Conversion (WCPEC) (A Joint Conference of 45th IEEE PVSC, 28th PVSEC & 34th EU PVSEC)(2018)
摘要
Defects in semiconductors, although atomistic in scale and often scarce in concentration, frequently represent the performance-limiting factor in optoelectronic devices such as solar cells. However, due to this scale and scarcity, direct experimental characterization of defects is technically challenging, time-consuming, and expensive. Even so, the fact that defects can limit device performance suggests that device-level characterization should be able to lend insight into their properties. In this work, we use Bayesian inference to demonstrate a way to relate experimental device measurements with defect properties (as well as other materials properties affected by the presence of defects, such as minority-carrier lifetime). We apply this method to solve the "inverse problem" to a forward device model - namely, determining which input parameters to the model produce the measured electrical output. This approach has distinct advantages over direct characterization. First, a single set of measurements can be used to determine many parameters (the number of which, in principle, is limited only by the computing resources available), saving time and cost of facilities and equipment. Second, since measurements are performed on materials and interfaces in their relevant device geometries (vs. separately prepared samples), the determined parameters are guaranteed to be physically relevant. We demonstrate application of this method to both tin monosulfide and silicon solar cells and discuss potential for future application in a broader array of systems.
更多查看译文
关键词
Bayes methods,charge carrier lifetime,charge carrier mobility,parameter estimation,photovoltaic cells,silicon
AI 理解论文
溯源树
样例
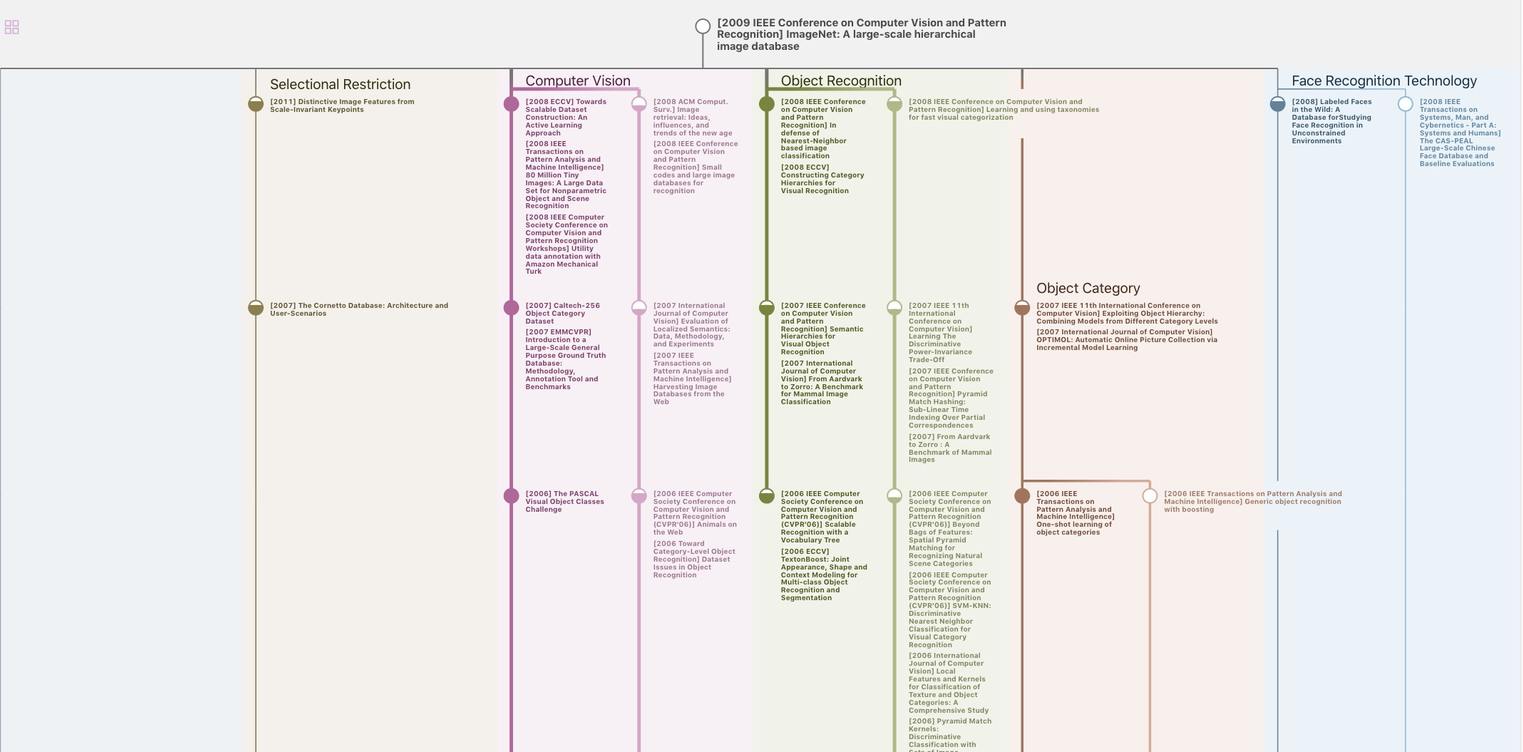
生成溯源树,研究论文发展脉络
Chat Paper
正在生成论文摘要