The Radius Inflation Problem in Short-Period Low-Mass Binaries: a Large Sample Analysis
Monthly Notices of the Royal Astronomical Society(2019)SCI 2区
Univ ECCI | Instituto de Astronomia | Univ Mil Nueva Granada
Abstract
Because of the recent increase in the availability of photometric time-series data bases, it is now possible to characterize low-mass eclipsing binaries in large samples and with good precision, in order to study their orbital and physical parameters. We have identified and photometrically characterized a sample of 230 detached close-orbiting eclipsing binaries with low-mass main-sequence components in the Catalina Sky Survey. These low-mass stars have masses of M <= 1.0 M-circle dot and orbital periods shorter than 2 d. The adopted method provides a robust estimate of stellar parameters (such as mass and fractional radius) by using only light curves and photometric colours, as no spectroscopic information was available for these objects. A ten-colour grid of composite synthetic and observed colours and the K-nearest neighbours method were employed to identify main-sequence stars and to estimate their effective temperatures, typically T-eff <= 5720 K. Each light curve was modelled with the JKTEBOP code, together with an asexual genetic algorithm to obtain the most coherent values for the fitted parameters. The present work provides an unprecedented number of homogeneous estimates of main stellar parameters in short-period low-mass binary systems. The distribution of the components of the investigated detached eclipsing binaries in the mass-radius diagram supports a trend of radius inflation on low-mass main-sequence stars. A relative increase of inflation for lower masses is also found and our results suggest that the secondaries are more inflated; that is, they present larger radii than the primary components of same mass, when compared with stellar evolutionary models.
MoreTranslated text
Key words
techniques: photometric,binaries: eclipsing,stars: late-type
PDF
View via Publisher
AI Read Science
AI Summary
AI Summary is the key point extracted automatically understanding the full text of the paper, including the background, methods, results, conclusions, icons and other key content, so that you can get the outline of the paper at a glance.
Example
Background
Key content
Introduction
Methods
Results
Related work
Fund
Key content
- Pretraining has recently greatly promoted the development of natural language processing (NLP)
- We show that M6 outperforms the baselines in multimodal downstream tasks, and the large M6 with 10 parameters can reach a better performance
- We propose a method called M6 that is able to process information of multiple modalities and perform both single-modal and cross-modal understanding and generation
- The model is scaled to large model with 10 billion parameters with sophisticated deployment, and the 10 -parameter M6-large is the largest pretrained model in Chinese
- Experimental results show that our proposed M6 outperforms the baseline in a number of downstream tasks concerning both single modality and multiple modalities We will continue the pretraining of extremely large models by increasing data to explore the limit of its performance
Try using models to generate summary,it takes about 60s
Must-Reading Tree
Example
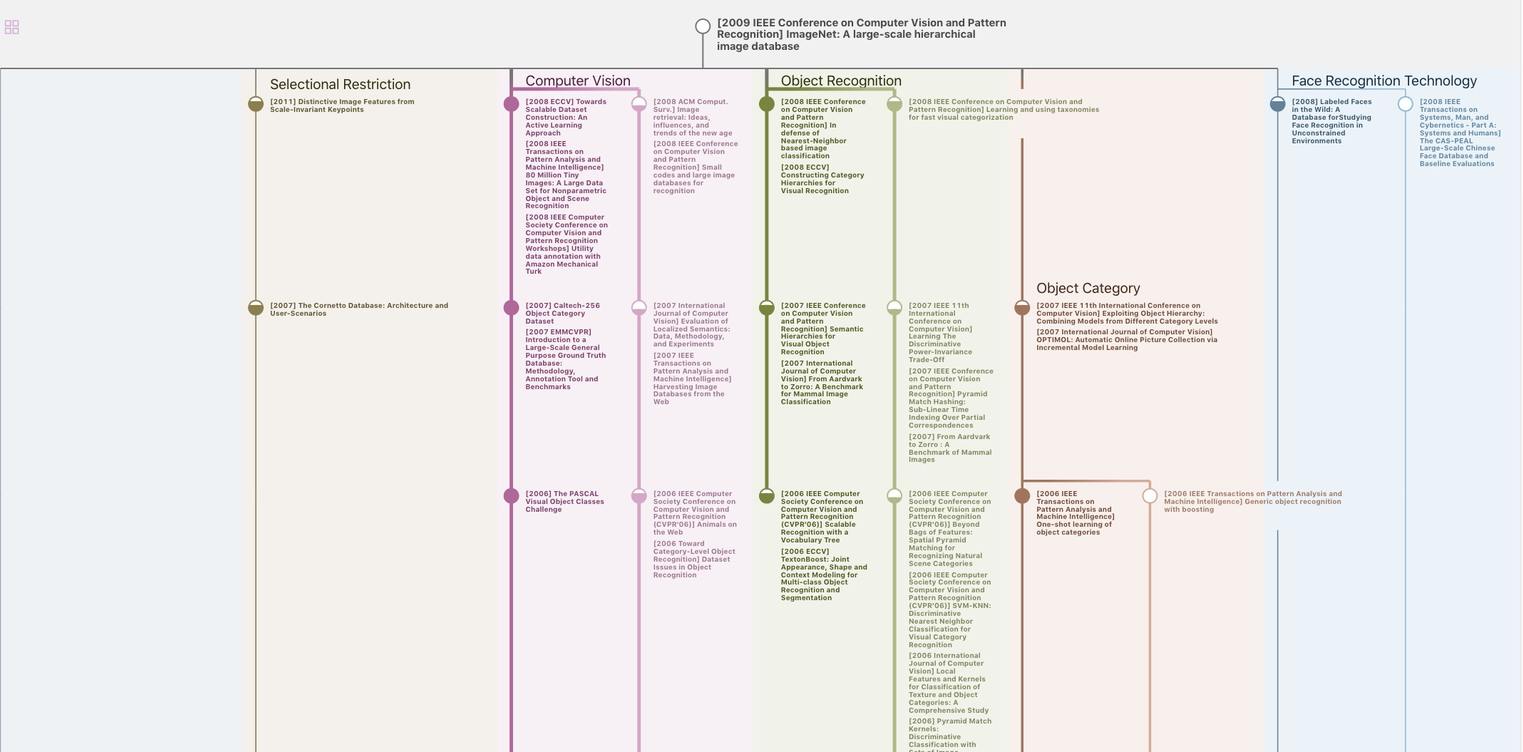
Generate MRT to find the research sequence of this paper
Related Papers
Recovering Variable Stars in Large Surveys: EA_ Up Algol-type Class in the Catalina Survey
Monthly Notices of the Royal Astronomical Society 2020
被引用5
Photodynamical Modeling of the Fascinating Eclipses in the Triple-Star System KOI-126
The Astrophysical Journal 2022
被引用4
Monthly Notices of the Royal Astronomical Society 2022
被引用3
Stellar Characterization and Radius Inflation of Hyades M Dwarf Stars from the APOGEE Survey
ASTROPHYSICAL JOURNAL 2023
被引用0
Data Disclaimer
The page data are from open Internet sources, cooperative publishers and automatic analysis results through AI technology. We do not make any commitments and guarantees for the validity, accuracy, correctness, reliability, completeness and timeliness of the page data. If you have any questions, please contact us by email: report@aminer.cn
Chat Paper
GPU is busy, summary generation fails
Rerequest