Causality Reconstruction by an Autonomous Agent
BIOLOGICALLY INSPIRED COGNITIVE ARCHITECTURES 2018(2018)
摘要
Most AI algorithms consider input data as “percepts” that the agent receives from the environment. Constructivist epistemology, however, suggests an alternative approach that considers the algorithm’s input data as feedback resulting from the agent’s actions. This paper introduces a constructivist algorithm to let an agent learn regularities of actions and feedback. The agent organizes its behaviors to fulfill a form of intentionality defined independently of a specific task. The experiment shows that this algorithm constructs a Petri net whose nodes represent hypothetical stable states afforded by the agent/environment coupling, and arcs represent transitions between such states. Since this Petri net allows the algorithm to predict the consequences of the agent’s actions, we argue that it constitutes a rudimentary causal model of the “world” (agent + environment) learned by the agent through experience of interaction. This work opens the way to studying how an autonomous agent can learn more complex causal models of more complex worlds, in particular by explaining regularities of interaction through the presence of objects in the agent’s surrounding space.
更多查看译文
关键词
Feedback,Constructivist paradigm,Interaction,Causality reconstruction,Developmental learning
AI 理解论文
溯源树
样例
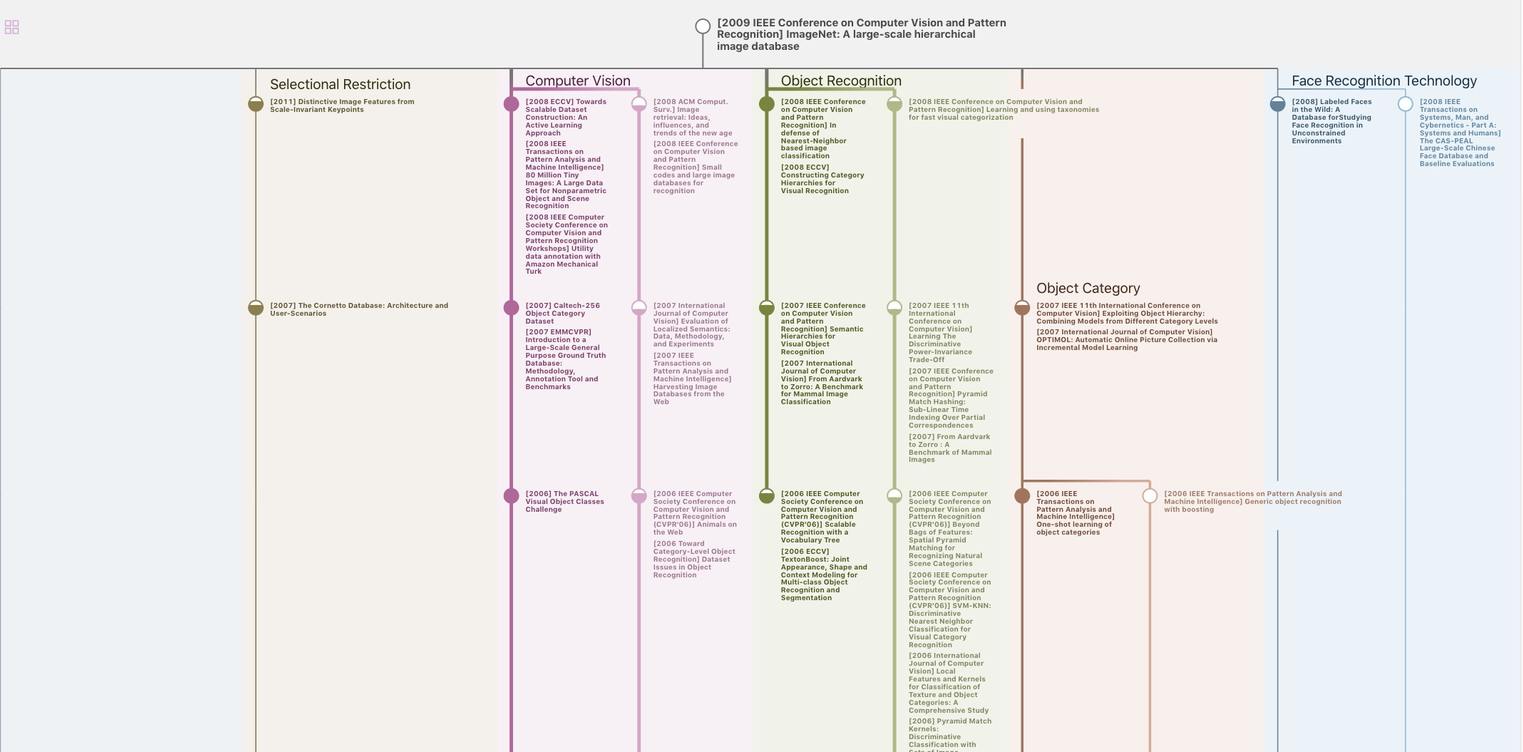
生成溯源树,研究论文发展脉络
Chat Paper
正在生成论文摘要