Radiomics for Survival Analysis and Prediction in Glioblastoma (GBM)—A Preliminary Study
International journal of radiation oncology, biology, physics(2016)
AI 理解论文
溯源树
样例
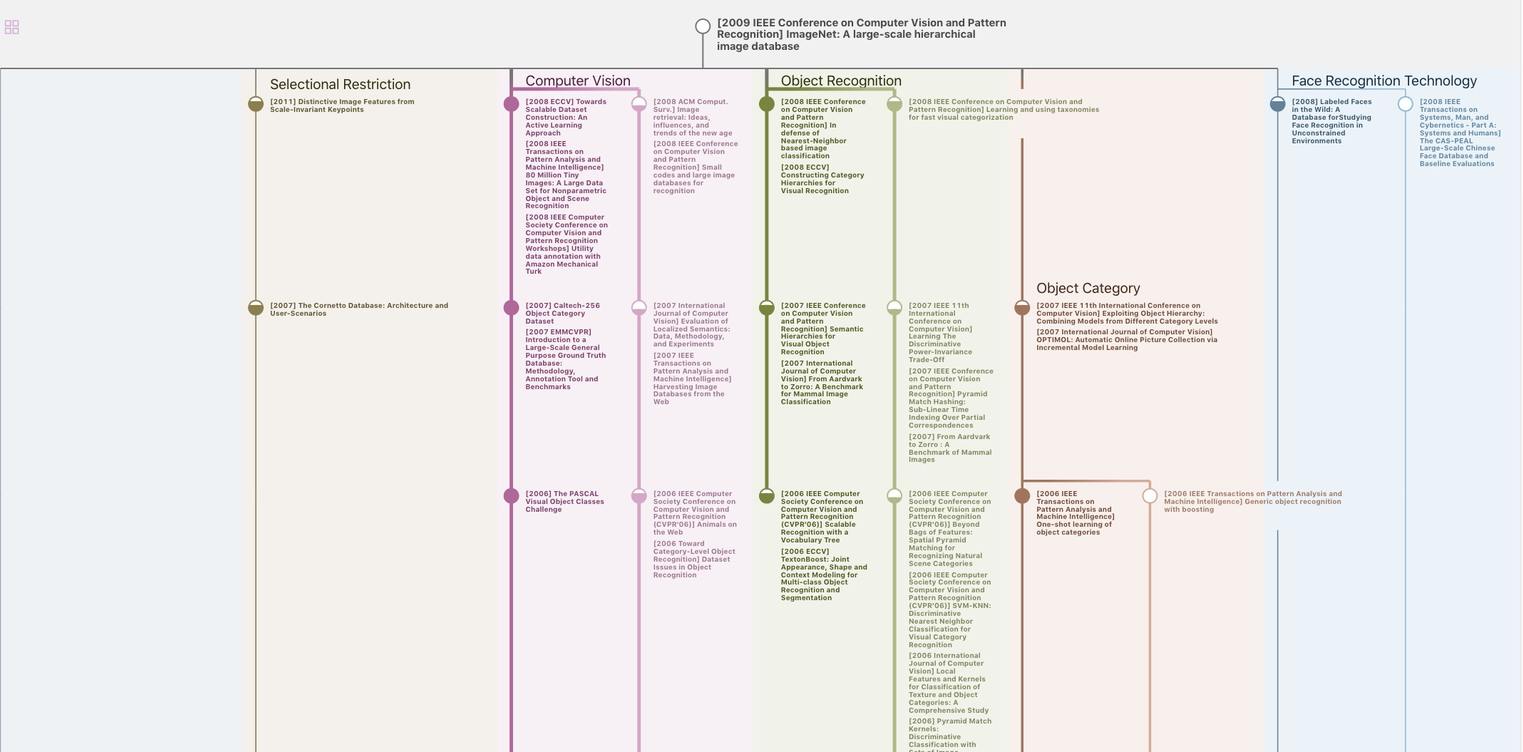
生成溯源树,研究论文发展脉络
Chat Paper
正在生成论文摘要
International journal of radiation oncology, biology, physics(2016)