Quantifying model uncertainty using Bayesian multi-model ensembles.
Environmental Modelling & Software(2019)
摘要
Watershed models are essential tools to understand, quantify, and predict hydrologic processes and water quality responses from scales ranging from field to large river basins. However, the reliability of watershed models in a management context depends largely on inherent uncertainties in model predictions. The objective of this study is to quantify prediction uncertainty for flow, sediment, total nitrogen (TN), and total phosphorus (TP) resulting from model structure. We do this using using three process-based models: the Soil and Water Assessment Tool-Variable Source Area model (SWAT-VSA), the standard Soil and Water Assessment Tool (SWAT-ST), and the Chesapeake Bay Program’s Phase 6 Watershed Model (CBP-Model). We initialize each of the models using meteorological, soil, and land use data, and analyze outputs of flow, sediment, TN, and TP fluxes at the U.S. Geological Survey stream gauge at the downstream end of the Susquehanna River Basin in Conowingo, Maryland. Using these three models, we develop and compare two types of Bayesian models, a Bayesian Generalized (Non-) Linear Multilevel Model (BGMM), and a Bayesian Model Averaging (BMA) for flow, sediment, TN, and TP and 95% credible intervals. We compare the Bayesian models results against the individual model results, and straight model averaging (SMA) using a split time period analysis to assess their predictive strengths. Both Bayesian models provided substantially better predictions than the individual process-based models, and estimates of prediction uncertainty, which can enhance decision-making and improve watershed management by providing a risk based assessment of outcomes.
更多查看译文
关键词
SWAT-VSA,SWAT-Standard,Chesapeake bay watershed model,Bayesian model ensemble,Multilevel models
AI 理解论文
溯源树
样例
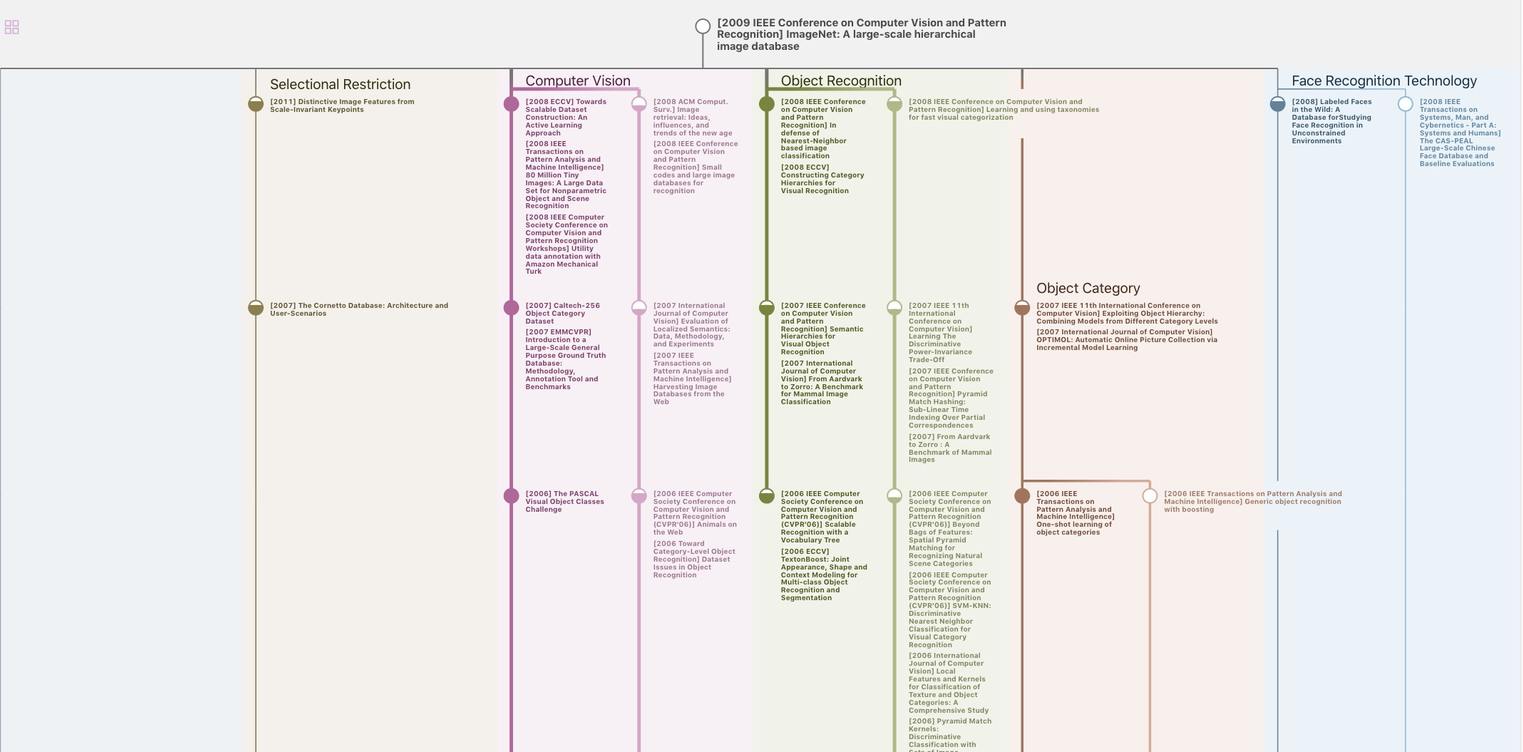
生成溯源树,研究论文发展脉络
Chat Paper
正在生成论文摘要