Increasing Precision for French Forest Inventory Estimates using the k-NN Technique with Optical and Photogrammetric Data and Model-Assisted Estimators.
REMOTE SENSING(2019)
摘要
Multisource forest inventory methods were developed to improve the precision of national forest inventory estimates. These methods rely on the combination of inventory data and auxiliary information correlated with forest attributes of interest. As these methods have been predominantly tested over coniferous forests, the present study used this approach for heterogeneous and complex deciduous forests in the center of France. The auxiliary data considered included a forest type map, Landsat 8 spectral bands and derived vegetation indexes, and 3D variables derived from photogrammetric canopy height models. On a subset area, changes in canopy height estimated from two successive photogrammetric models were also used. A model-assisted inference framework, using a k nearest-neighbors approach, was used to predict 11 field inventory variables simultaneously. The results showed that among the auxiliary variables tested, 3D metrics improved the precision of dendrometric estimates more than other auxiliary variables. Relative efficiencies (RE) varying from 2.15 for volume to 1.04 for stand density were obtained using all auxiliary variables. Canopy height changes also increased RE from 3% to 26%. Our results confirmed the importance of 3D metrics as auxiliary variables and demonstrated the value of canopy change variables for increasing the precision of estimates of forest structural attributes such as density and quadratic mean diameter.
更多查看译文
关键词
multisource forest inventory,national forest inventory,non-parametric models,statistical inference,digital photogrammetry
AI 理解论文
溯源树
样例
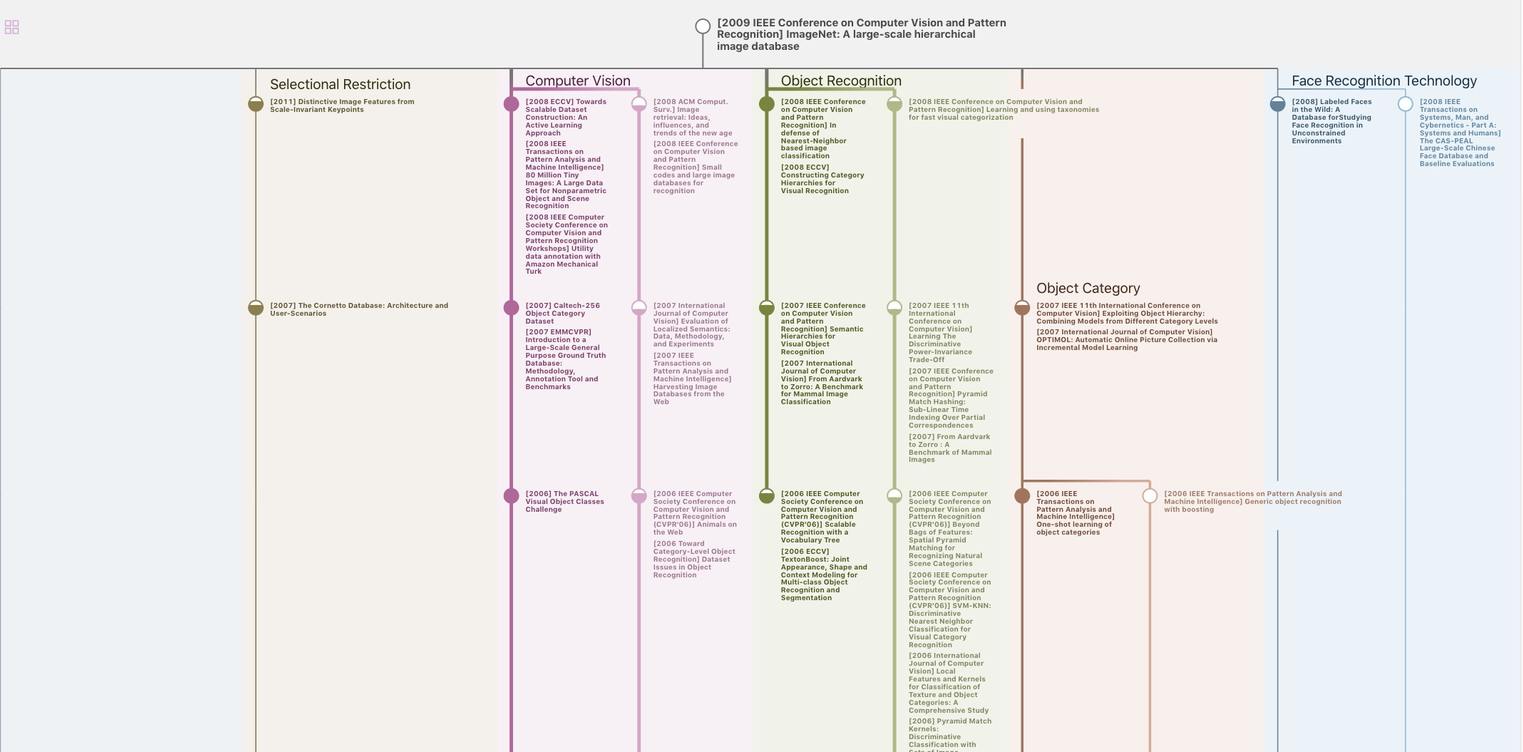
生成溯源树,研究论文发展脉络
Chat Paper
正在生成论文摘要