An Efficient Deep Learning Approach to Pneumonia Classification in Healthcare
Journal of healthcare engineering(2019)SCI 4区
Abstract
This study proposes a convolutional neural network model trained from scratch to classify and detect the presence of pneumonia from a collection of chest X-ray image samples. Unlike other methods that rely solely on transfer learning approaches or traditional handcrafted techniques to achieve a remarkable classification performance, we constructed a convolutional neural network model from scratch to extract features from a given chest X-ray image and classify it to determine if a person is infected with pneumonia. This model could help mitigate the reliability and interpretability challenges often faced when dealing with medical imagery. Unlike other deep learning classification tasks with sufficient image repository, it is difficult to obtain a large amount of pneumonia dataset for this classification task; therefore, we deployed several data augmentation algorithms to improve the validation and classification accuracy of the CNN model and achieved remarkable validation accuracy.
MoreTranslated text
PDF
View via Publisher
AI Read Science
AI Summary
AI Summary is the key point extracted automatically understanding the full text of the paper, including the background, methods, results, conclusions, icons and other key content, so that you can get the outline of the paper at a glance.
Example
Background
Key content
Introduction
Methods
Results
Related work
Fund
Key content
- Pretraining has recently greatly promoted the development of natural language processing (NLP)
- We show that M6 outperforms the baselines in multimodal downstream tasks, and the large M6 with 10 parameters can reach a better performance
- We propose a method called M6 that is able to process information of multiple modalities and perform both single-modal and cross-modal understanding and generation
- The model is scaled to large model with 10 billion parameters with sophisticated deployment, and the 10 -parameter M6-large is the largest pretrained model in Chinese
- Experimental results show that our proposed M6 outperforms the baseline in a number of downstream tasks concerning both single modality and multiple modalities We will continue the pretraining of extremely large models by increasing data to explore the limit of its performance
Try using models to generate summary,it takes about 60s
Must-Reading Tree
Example
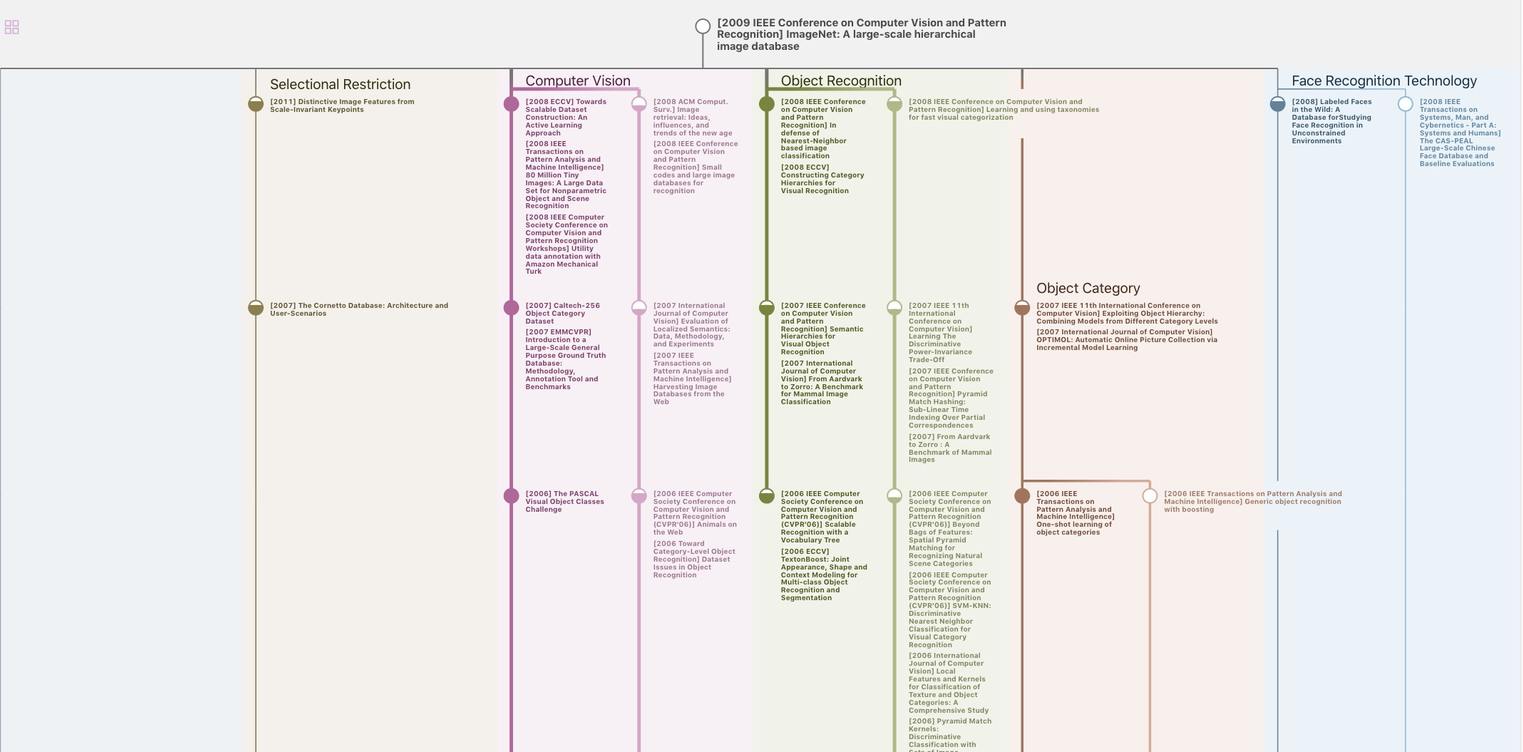
Generate MRT to find the research sequence of this paper
Related Papers
2010
被引用206 | 浏览
2004
被引用528 | 浏览
2014
被引用111 | 浏览
2004
被引用89 | 浏览
2015
被引用228 | 浏览
2017
被引用171 | 浏览
2017
被引用151 | 浏览
2022
被引用155 | 浏览
2017
被引用1360 | 浏览
2016
被引用7849 | 浏览
Data Disclaimer
The page data are from open Internet sources, cooperative publishers and automatic analysis results through AI technology. We do not make any commitments and guarantees for the validity, accuracy, correctness, reliability, completeness and timeliness of the page data. If you have any questions, please contact us by email: report@aminer.cn
Chat Paper
去 AI 文献库 对话