MixHop: Higher-Order Graph Convolutional Architectures Via Sparsified Neighborhood Mixing
arXiv (Cornell University)(2019)
摘要
Existing popular methods for semi-supervised learning with Graph Neural Networks (such as the Graph Convolutional Network) provably cannot learn a general class of neighborhood mixing relationships. To address this weakness, we propose a new model, MixHop, that can learn these relationships, including difference operators, by repeatedly mixing feature representations of neighbors at various distances. Mixhop requires no additional memory or computational complexity, and outperforms on challenging baselines. In addition, we propose sparsity regularization that allows us to visualize how the network prioritizes neighborhood information across different graph datasets. Our analysis of the learned architectures reveals that neighborhood mixing varies per datasets.
更多查看译文
关键词
Signal Processing on Graphs,Semi-Supervised Learning,Knowledge Graph Embedding,Graph Convolutional Networks,Graph Neural Networks
AI 理解论文
溯源树
样例
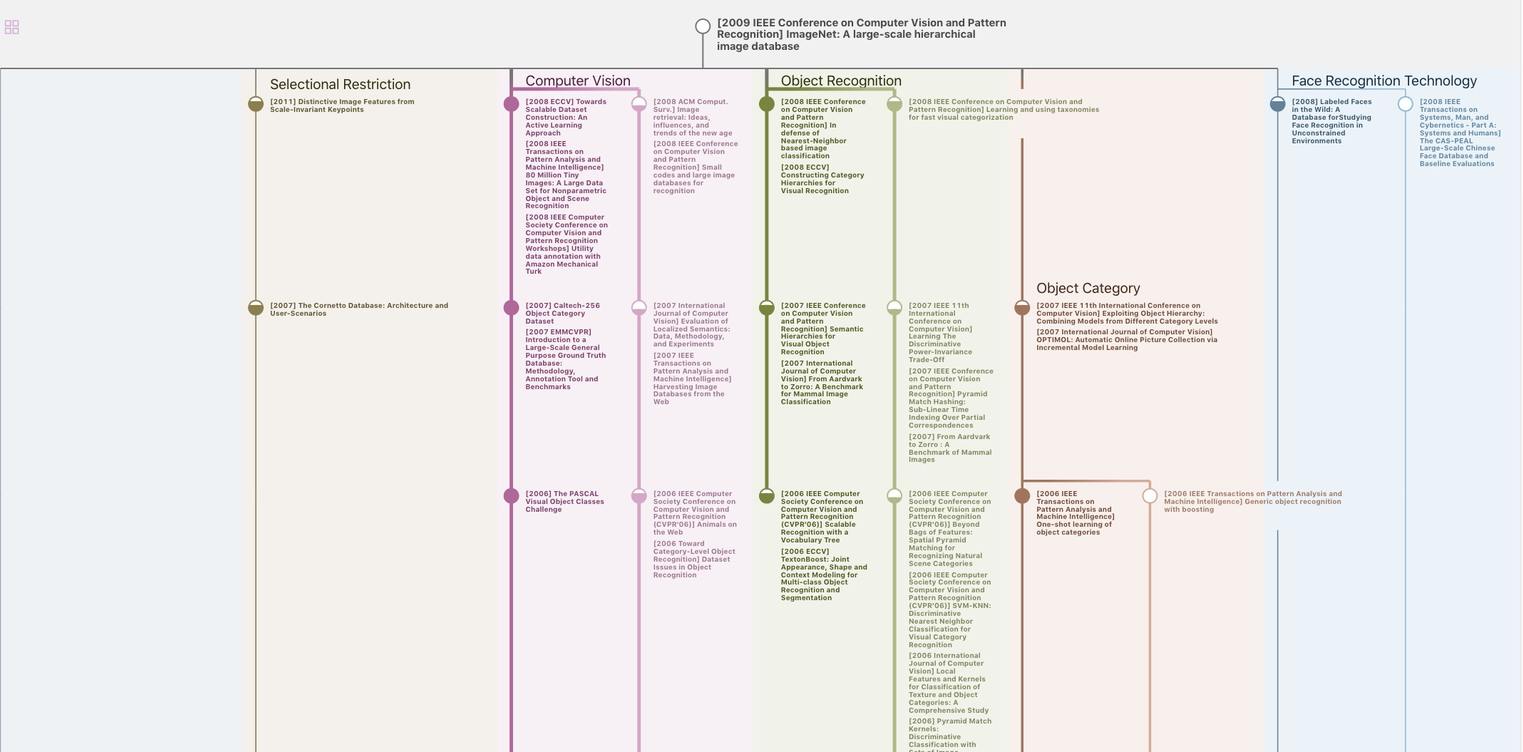
生成溯源树,研究论文发展脉络
Chat Paper
正在生成论文摘要