Stochastic Wasserstein Autoencoder for Probabilistic Sentence Generation.
2019 CONFERENCE OF THE NORTH AMERICAN CHAPTER OF THE ASSOCIATION FOR COMPUTATIONAL LINGUISTICS: HUMAN LANGUAGE TECHNOLOGIES (NAACL HLT 2019), VOL. 1(2019)
摘要
The variational autoencoder (VAE) imposes a probabilistic distribution (typically Gaussian) on the latent space and penalizes the Kullback--Leibler (KL) divergence between the posterior and prior. In NLP, VAEs are extremely difficult to train due to the problem of KL collapsing to zero. One has to implement various heuristics such as KL weight annealing and word dropout in a carefully engineered manner to successfully train a VAE for text. In this paper, we propose to use the Wasserstein autoencoder (WAE) for probabilistic sentence generation, where the encoder could be either stochastic or deterministic. We show theoretically and empirically that, in the original WAE, the stochastically encoded Gaussian distribution tends to become a Dirac-delta function, and we propose a variant of WAE that encourages the stochasticity of the encoder. Experimental results show that the latent space learned by WAE exhibits properties of continuity and smoothness as in VAEs, while simultaneously achieving much higher BLEU scores for sentence reconstruction.
更多查看译文
AI 理解论文
溯源树
样例
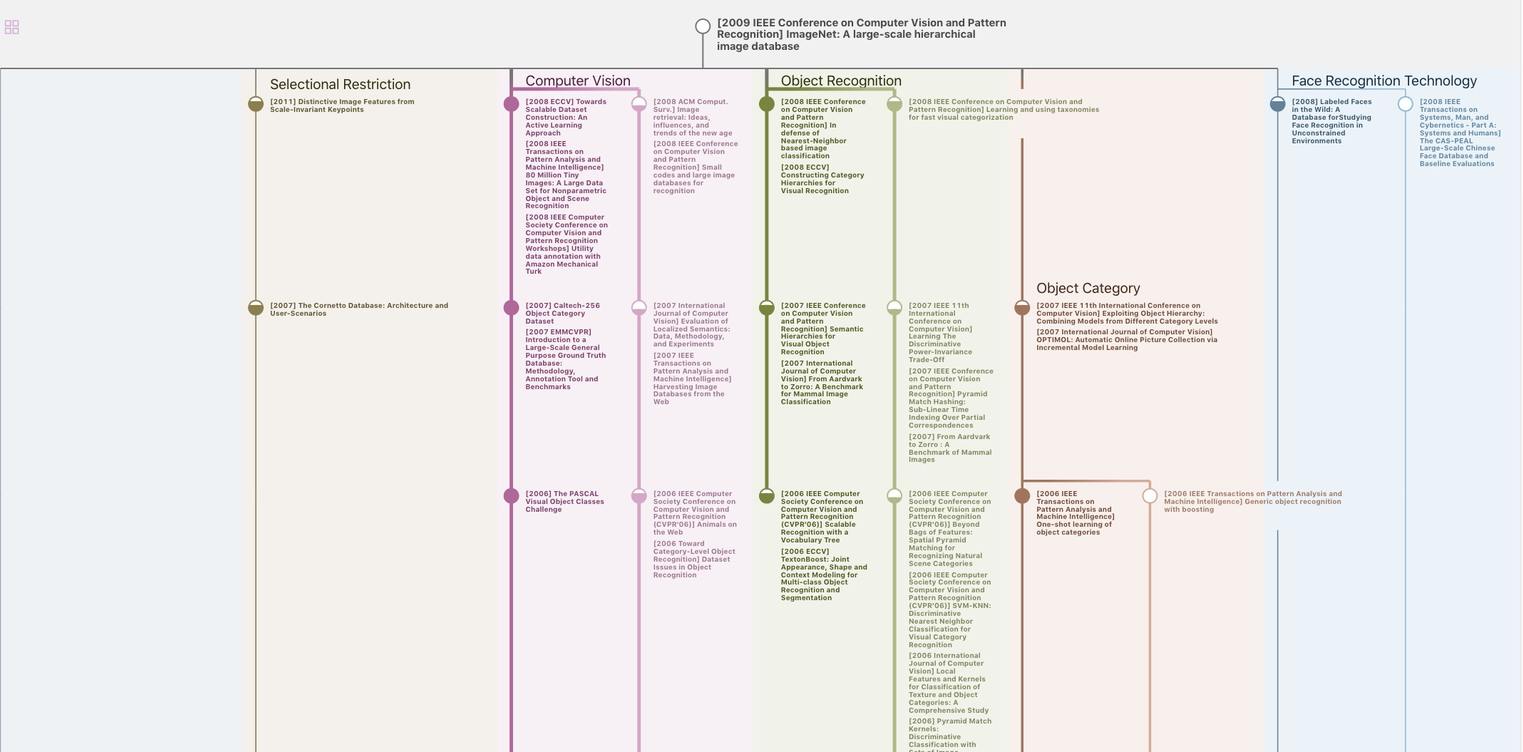
生成溯源树,研究论文发展脉络
Chat Paper
正在生成论文摘要