Biophysical and Biomolecular Determination of Cellular Age in Humans
Nature Biomedical Engineering(2017)SCI 1区
Department of Chemical and Biomolecular Engineering | Johns Hopkins Physical Sciences—Oncology Center | Department of Biomedical Engineering | Department of Medicine
Abstract
Ageing research has focused either on assessing organ- and tissue-based changes, such as lung capacity and cardiac function, or on changes at the molecular scale such as gene expression, epigenetic modifications and metabolism. Here, by using a cohort of 32 samples of primary dermal fibroblasts collected from individuals between 2 and 96 years of age, we show that the degradation of functional cellular biophysical features-including cell mechanics, traction strength, morphology and migratory potential-and associated descriptors of cellular heterogeneity predict cellular age with higher accuracy than conventional biomolecular markers. We also demonstrate the use of high-throughput single-cell technologies, together with a deterministic model based on cellular features, to compute the cellular age of apparently healthy males and females, and to explore these relationships in cells from individuals with Werner syndrome and Hutchinson-Gilford progeria syndrome, two rare genetic conditions that result in phenotypes that show aspects of premature ageing. Our findings suggest that the quantification of cellular age may be used to stratify individuals on the basis of cellular phenotypes and serve as a biological proxy of healthspan.
MoreTranslated text
Key words
Biomedical engineering,Cell biology,Biomedicine,general,Biomedical Engineering/Biotechnology
PDF
View via Publisher
AI Read Science
AI Summary
AI Summary is the key point extracted automatically understanding the full text of the paper, including the background, methods, results, conclusions, icons and other key content, so that you can get the outline of the paper at a glance.
Example
Background
Key content
Introduction
Methods
Results
Related work
Fund
Key content
- Pretraining has recently greatly promoted the development of natural language processing (NLP)
- We show that M6 outperforms the baselines in multimodal downstream tasks, and the large M6 with 10 parameters can reach a better performance
- We propose a method called M6 that is able to process information of multiple modalities and perform both single-modal and cross-modal understanding and generation
- The model is scaled to large model with 10 billion parameters with sophisticated deployment, and the 10 -parameter M6-large is the largest pretrained model in Chinese
- Experimental results show that our proposed M6 outperforms the baseline in a number of downstream tasks concerning both single modality and multiple modalities We will continue the pretraining of extremely large models by increasing data to explore the limit of its performance
Try using models to generate summary,it takes about 60s
Must-Reading Tree
Example
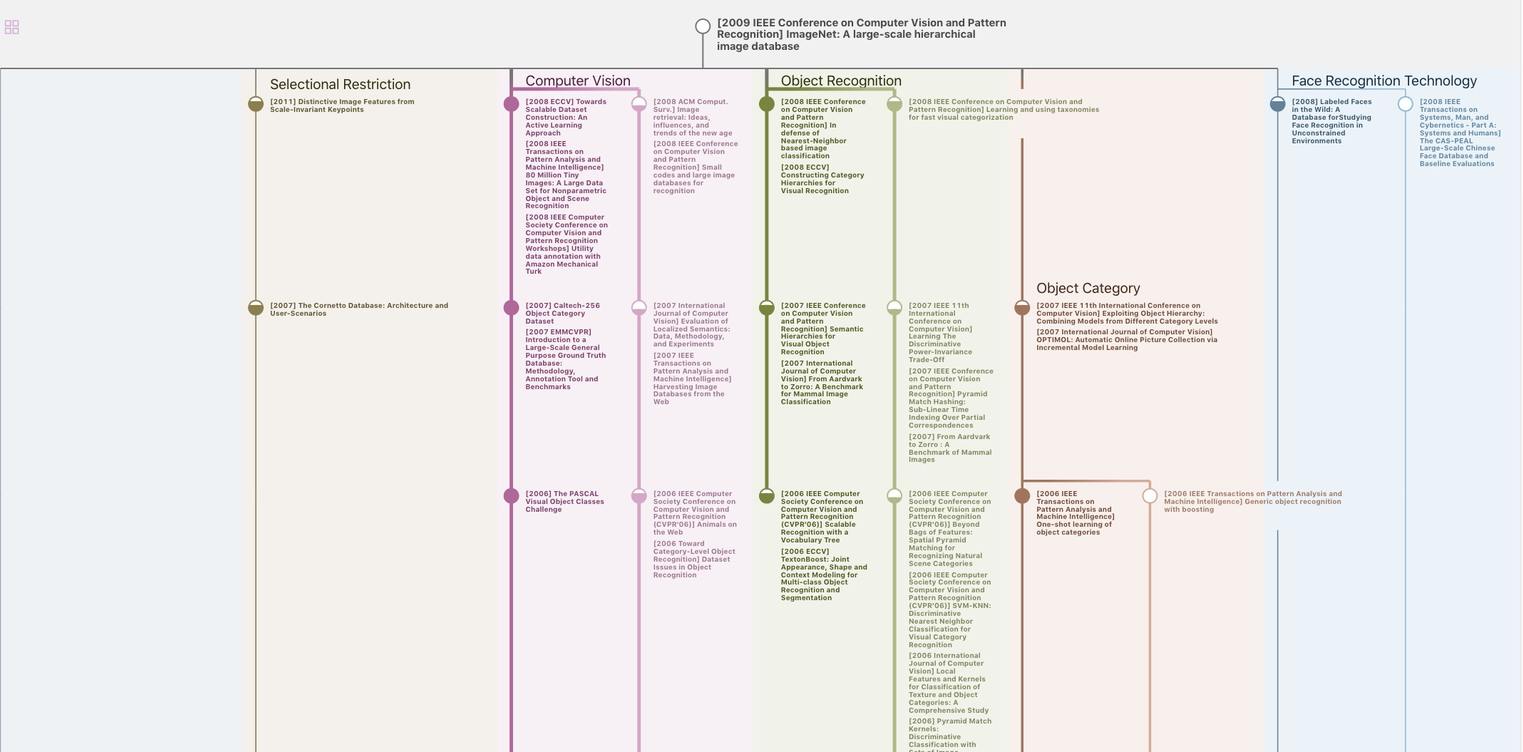
Generate MRT to find the research sequence of this paper
Related Papers
2005
被引用128 | 浏览
2005
被引用115 | 浏览
2006
被引用230 | 浏览
2007
被引用271 | 浏览
2001
被引用504 | 浏览
2013
被引用39 | 浏览
2010
被引用86 | 浏览
2006
被引用106 | 浏览
2011
被引用1293 | 浏览
2008
被引用194 | 浏览
2009
被引用1575 | 浏览
Data Disclaimer
The page data are from open Internet sources, cooperative publishers and automatic analysis results through AI technology. We do not make any commitments and guarantees for the validity, accuracy, correctness, reliability, completeness and timeliness of the page data. If you have any questions, please contact us by email: report@aminer.cn
Chat Paper
GPU is busy, summary generation fails
Rerequest