Improving galaxy morphology with machine learning
arXiv: Astrophysics of Galaxies(2017)
Abstract
This paper presents machine learning experiments performed over results of galaxy classification into elliptical (E) and spiral (S) with morphological parameters: concetration (CN), assimetry metrics (A3), smoothness metrics (S3), entropy (H) and gradient pattern analysis parameter (GA). Except concentration, all parameters performed a image segmentation pre-processing. For supervision and to compute confusion matrices, we used as true label the galaxy classification from GalaxyZoo. With a 48145 objects dataset after preprocessing (44760 galaxies labeled as S and 3385 as E), we performed experiments with Support Vector Machine (SVM) and Decision Tree (DT). Whit a 1962 objects balanced dataset, we applied K- means and Agglomerative Hierarchical Clustering. All experiments with supervision reached an Overall Accuracy OA u003e= 97%.
MoreTranslated text
Key words
galaxy morphology,machine learning
AI Read Science
Must-Reading Tree
Example
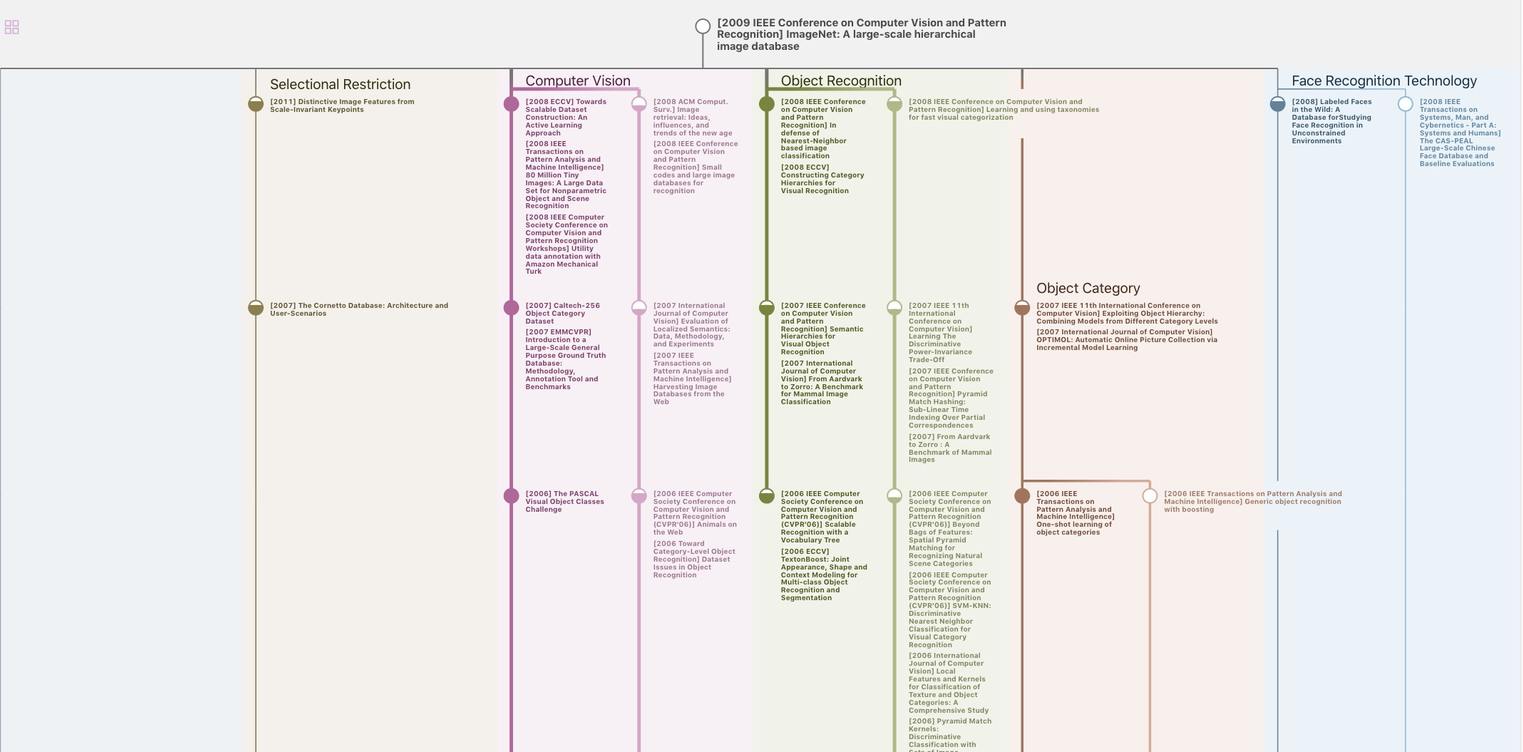
Generate MRT to find the research sequence of this paper
Chat Paper
Summary is being generated by the instructions you defined