Neural Forecasting and Optimal Sizing for Hybrid Renewable Energy Systems with Grid-Connected Storage System
Journal of renewable and sustainable energy(2016)
AI 理解论文
溯源树
样例
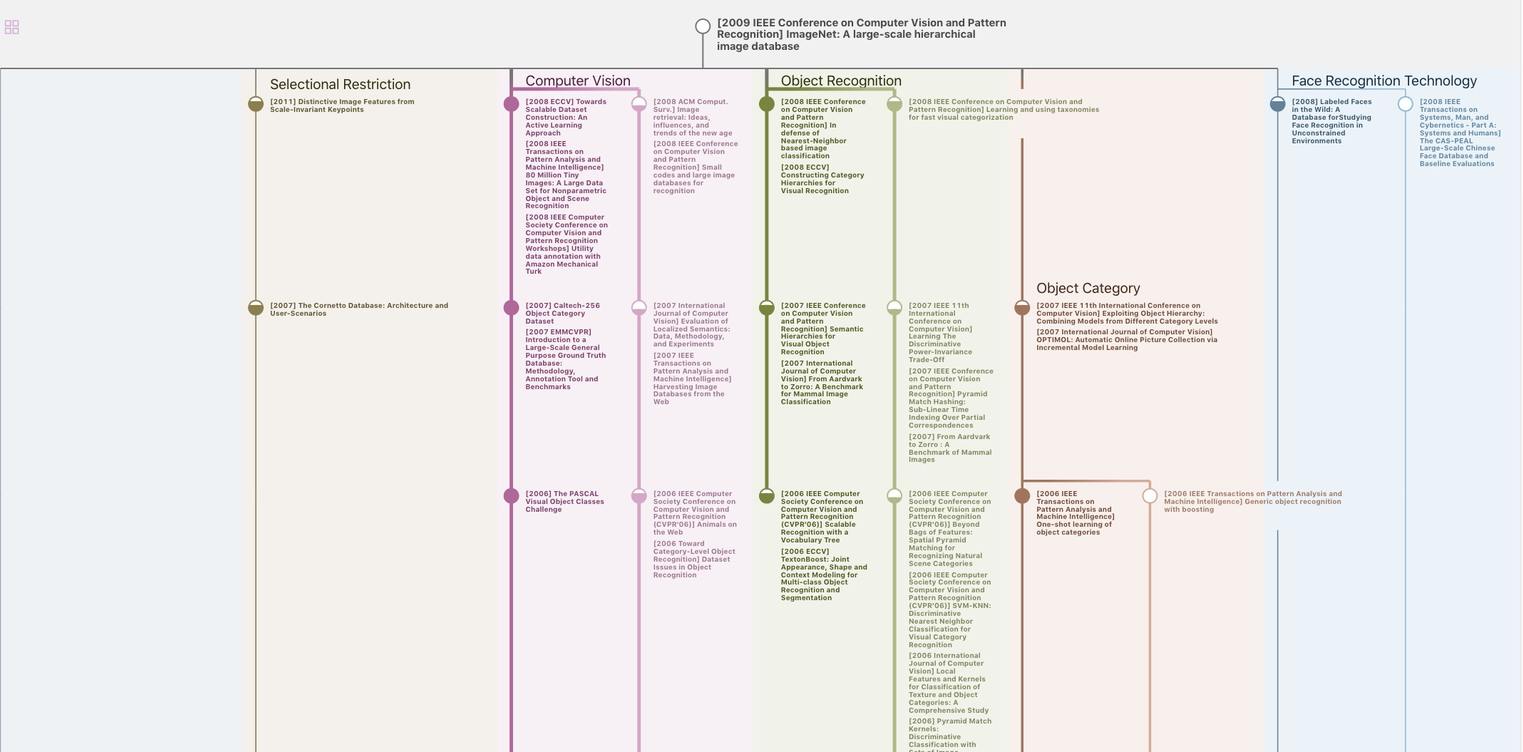
生成溯源树,研究论文发展脉络
Chat Paper
正在生成论文摘要
Journal of renewable and sustainable energy(2016)