MULTIFIDELITY DIMENSION REDUCTION VIA ACTIVE
SIAM JOURNAL ON SCIENTIFIC COMPUTING(2020)
摘要
We propose a multifidelity dimension reduction method to identify a low-dimensional structure present in many engineering models. The structure of interest arises when functions vary primarily on a low-dimensional subspace of the high-dimensional input space, while varying little along the complementary directions. Our approach builds on the gradient-based methodology of active subspaces, and exploits models of different fidelities to reduce the cost of performing dimension reduction through the computation of the active subspace matrix. We provide a nonasymptotic analysis of the number of gradient evaluations sufficient to achieve a prescribed error in the active subspace matrix, both in expectation and with high probability. We show that the sample complexity depends on a notion of intrinsic dimension of the problem, which can be much smaller than the dimension of the input space. We illustrate the benefits of such a multifidelity dimension reduction approach using numerical experiments with input spaces of up to two thousand dimensions.
更多查看译文
关键词
dimension reduction,multifidelity,gradient-based,active subspace,intrinsic dimension,effective rank,matrix Bernstein inequality,control variate
AI 理解论文
溯源树
样例
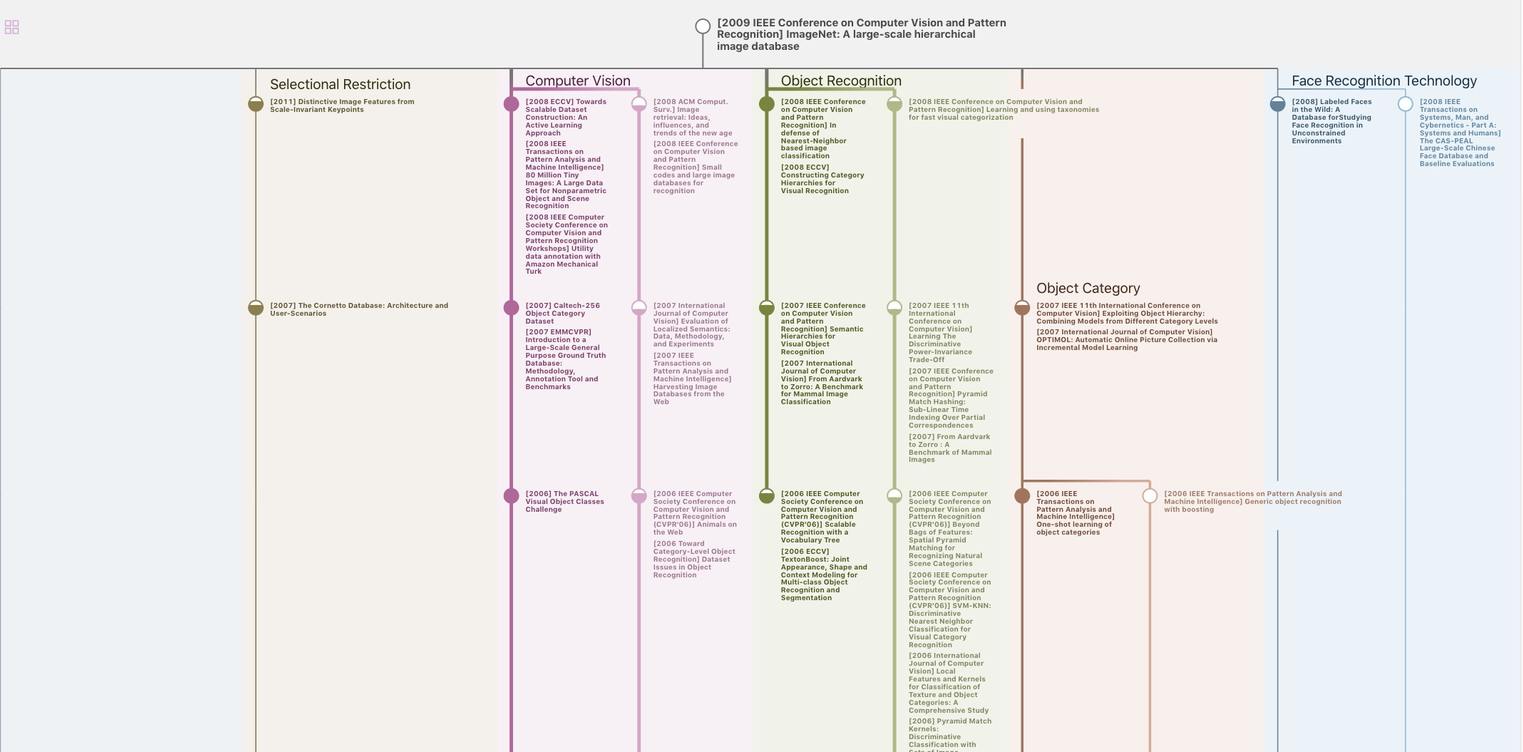
生成溯源树,研究论文发展脉络
Chat Paper
正在生成论文摘要