Aircraft Detection of High-Resolution Remote Sensing Image Based on Faster R-CNN Model and SSD Model
Proceedings of the 2018 International Conference on Image and Graphics Processing(2018)
摘要
With the continuous improvement of the space resolution in remote sensing images, the rapid and accurate detection in high-resolution remote sensing images has become a hotspot in the field of remote sensing application. For nearly 10 years, deep learning has made outstanding achievements in the feature extraction of original image and received attention of a large number of scholars. Among them, the convolutional neural network (CNN) has made breakthrough progress in the field of image classification and detection, and has overcome three shortcomings of the original remote sensing image detection method: low detection efficiency, redundant human resource input, and flawed feature selection. In this paper, Faster R-CNN model and SSD model are trained by high-resolution remote sensing images. The appropriate training time is determined by the detection results of verification set and the loss function. When we get trained models, it will be used to detect the test set images, and the accuracy rate and recall rate of two models were calculated by visual interpretation method. The experimental results show that both the Faster R-CNN model and the SSD model can be applied to aircraft detection in corresponding high-resolution remote sensing images. The SSD model can detect the single scene aircraft quickly and accurately. The Faster R-CNN model has a high accuracy but cannot reach the requirement of real-time detection. Besides, the accuracy rate and recall rate of Faster R-CNN model was significantly higher than the SSD model in the complex scenes, and the Faster R-CNN model has a great advantage for the detection of small aircraft.
更多查看译文
关键词
Convolutional neural network,Faster-RCNN,SSD,object detection
AI 理解论文
溯源树
样例
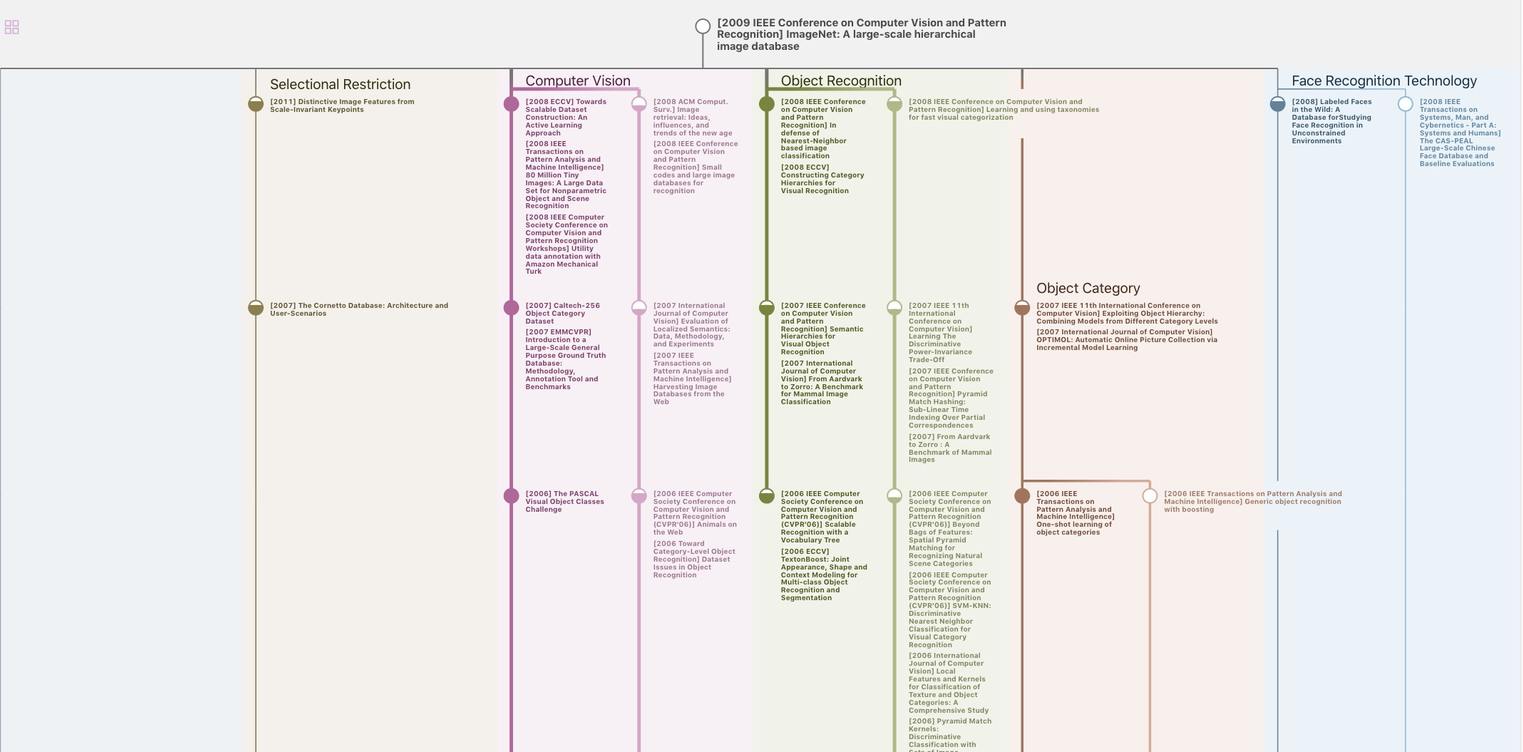
生成溯源树,研究论文发展脉络
Chat Paper
正在生成论文摘要