Model Selection With Lasso-Zero: Adding Straw to the Haystack to Better Find Needles
Journal of computational and graphical statistics(2021)
摘要
The high-dimensional linear model y = x beta(0) + epsilon is considered and the focus is put on the problem of recovering the support S-0 of the sparse vector beta(0). We introduce a new l(1)-based estimator, called Lasso-Zero, whose novelty resides in the repeated use of noise dictionaries concatenated to X for overfitting the response. Lasso-Zero is an extension of thresholded basis pursuit (TBP), for which we prove sign consistency for correlated Gaussian designs. Both theoretical and empirical results motivate the use of noise dictionaries to improve TBP when the coefficients' amplitude is low. To select the threshold of Lasso-Zero, we suggest to employ a pivotal version of the quantile universal threshold (QUT) that exploits a byproduct of Lasso-Zero to avoid the need to estimate the noise level. Numerical simulations show that Lasso-Zero tuned by QUT performs well in terms of support recovery and provides an excellent trade-off between high power and few false discoveries compared to competitors. Supplemental materials for this article are available online.
更多查看译文
关键词
High dimension,Linear regression,Sparsity,Support recovery,Thresholded basis pursuit,Variable selection
AI 理解论文
溯源树
样例
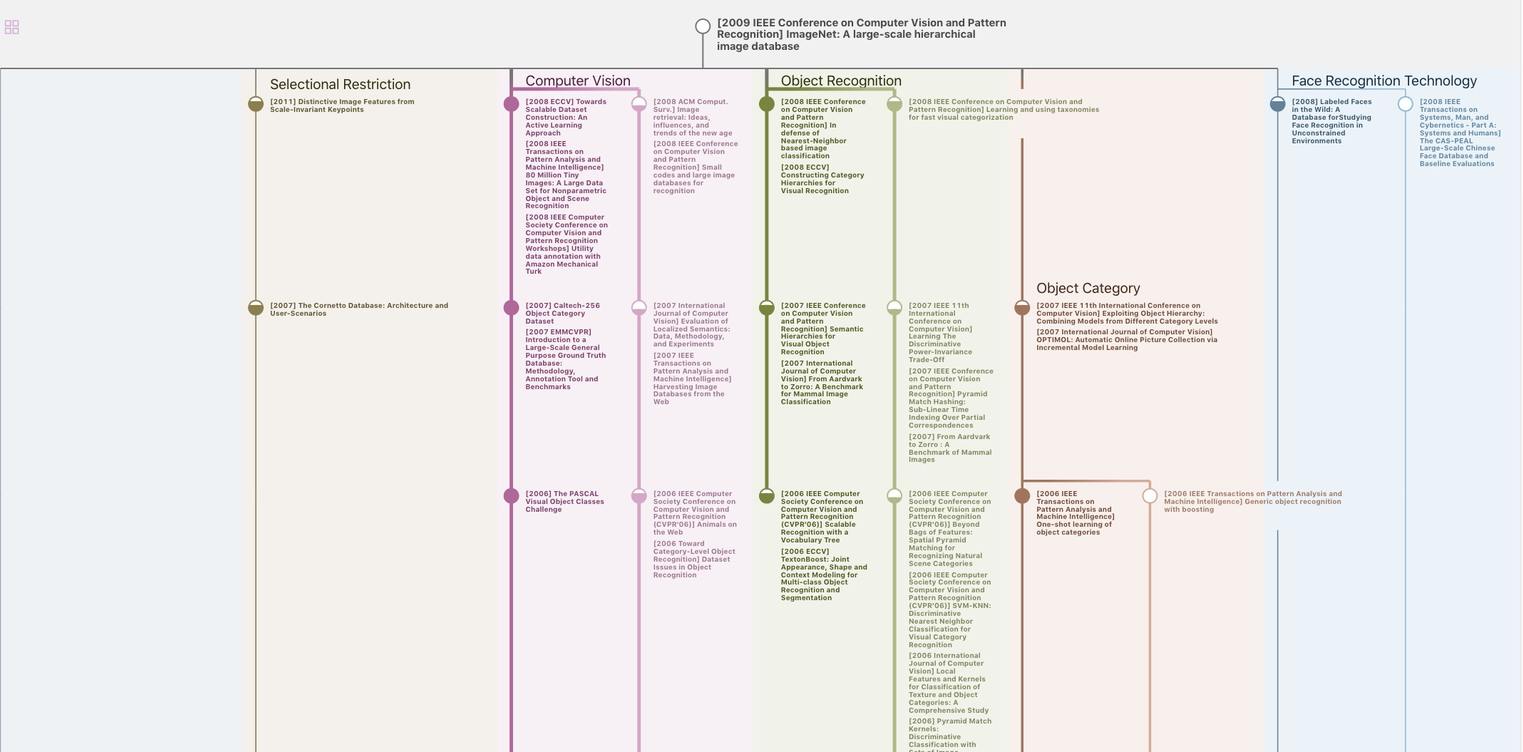
生成溯源树,研究论文发展脉络
Chat Paper
正在生成论文摘要