Data-driven Urban Energy Simulation (DUE-S): Integrating machine learning into an urban building energy simulation workflow
Energy Procedia(2017)
摘要
Urban building energy models are emerging tools meant to analyze and understand the energy performance of multiple buildings within a dense urban area. However, accurate performance prediction of these models remains a challenge because of their inability to account for the inter-building energy dynamics and interdependencies in an urban area. This paper analyzes the literature to highlight the limitations of current urban scale energy simulation models and proposes a new Data-driven Urban Energy Simulation (DUE-S) workflow capable of capturing inter-building effects on a building's energy usage. Specifically, DUE-S combines a data-driven machine learning model with a traditional physics-based energy simulation to enable more accurate simulation results on multiple scales (single building, community, urban). More accurate and robust energy performance characterization and simulations of urban buildings could provide valuable insight on early-stage building design, building conservation and portfolio management, and urban energy efficiency policy-making crucial to helping our cities transition to a more sustainable energy future. (C) 2017 The Authors. Published by Elsevier Ltd. Peer-review under responsibility of the scientific committee of the 9th International Conference on Applied Energy.
更多查看译文
关键词
building performance,energy efficiency,energy data,machine learning,simulation,urban sustainability
AI 理解论文
溯源树
样例
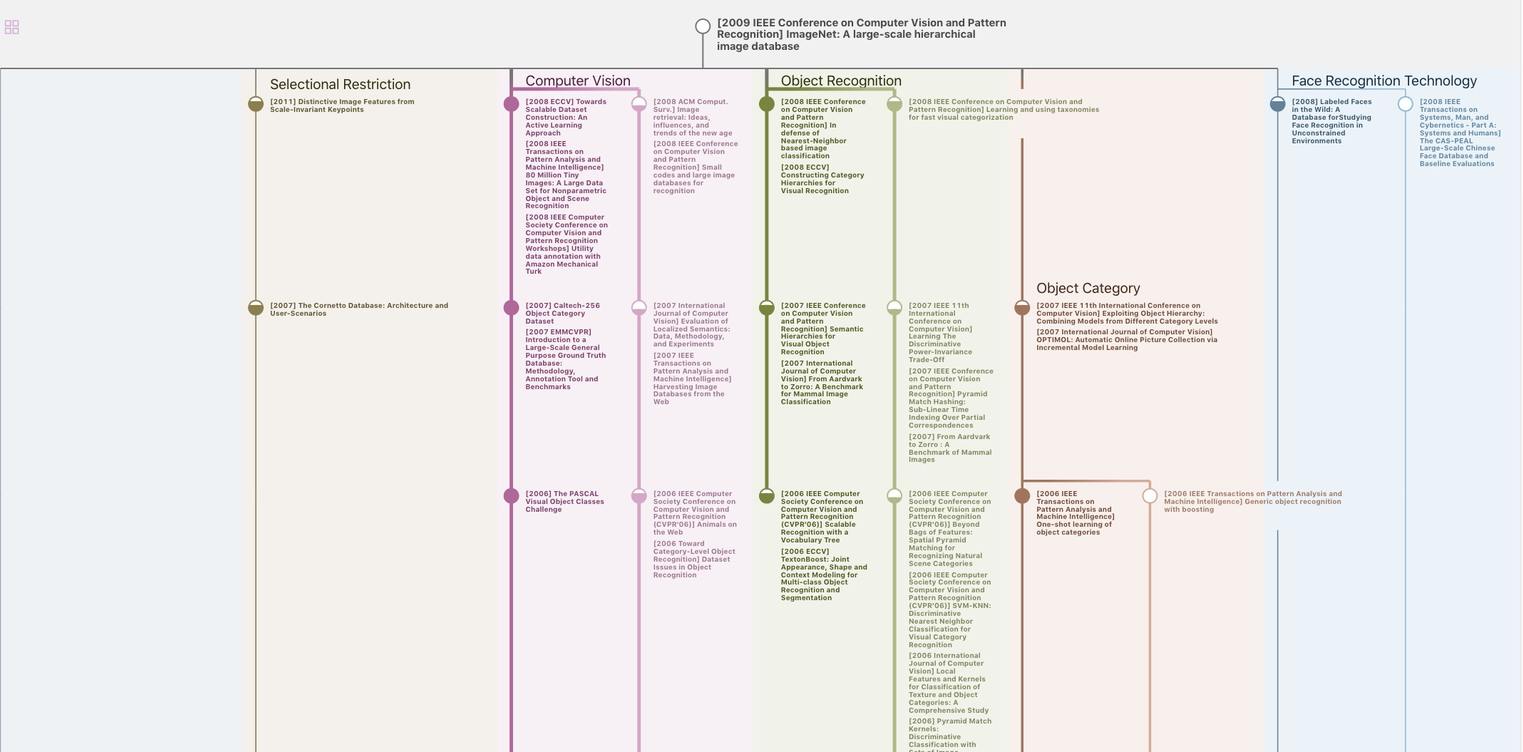
生成溯源树,研究论文发展脉络
Chat Paper
正在生成论文摘要