Sampling Method for GMM Curve Fitting in Multi Object Tracking
2017 IEEE International Conference on Imaging Systems and Techniques (IST)(2017)
摘要
Gaussian Mixture Models (GMM) have been widely used in computer vision problems due to the isotropic nature of the Gaussian functions, and more importantly, mathematical tractability. Fitting the shape of the data based on GMM is a common method to get regional information by extracting the resulting parameters in each single Gauss. However, most technology used to deal with this problem is a traditional method of curve fitting, ignoring the unique character of GMM. In this paper, we successfully translate the fitting issue into density estimation problem, and thus we can employ the powerful iteration algorithm Expectation Maximization (EM) under the Maximum Likelihood frame. Two ways of sampling are proposed to construct density estimation: deterministic sample and Monte Carlo sample. Both simulation and object tracking data experiments show that when dealing with data of obvious Mixture of Gaussian or no fat tail involved, the deterministic sample approach have a better and stable result, but when it comes to heavy fat tail data, the latter would be a better choice.
更多查看译文
关键词
curve fitting,density estimation,EM,GMM,target tracking
AI 理解论文
溯源树
样例
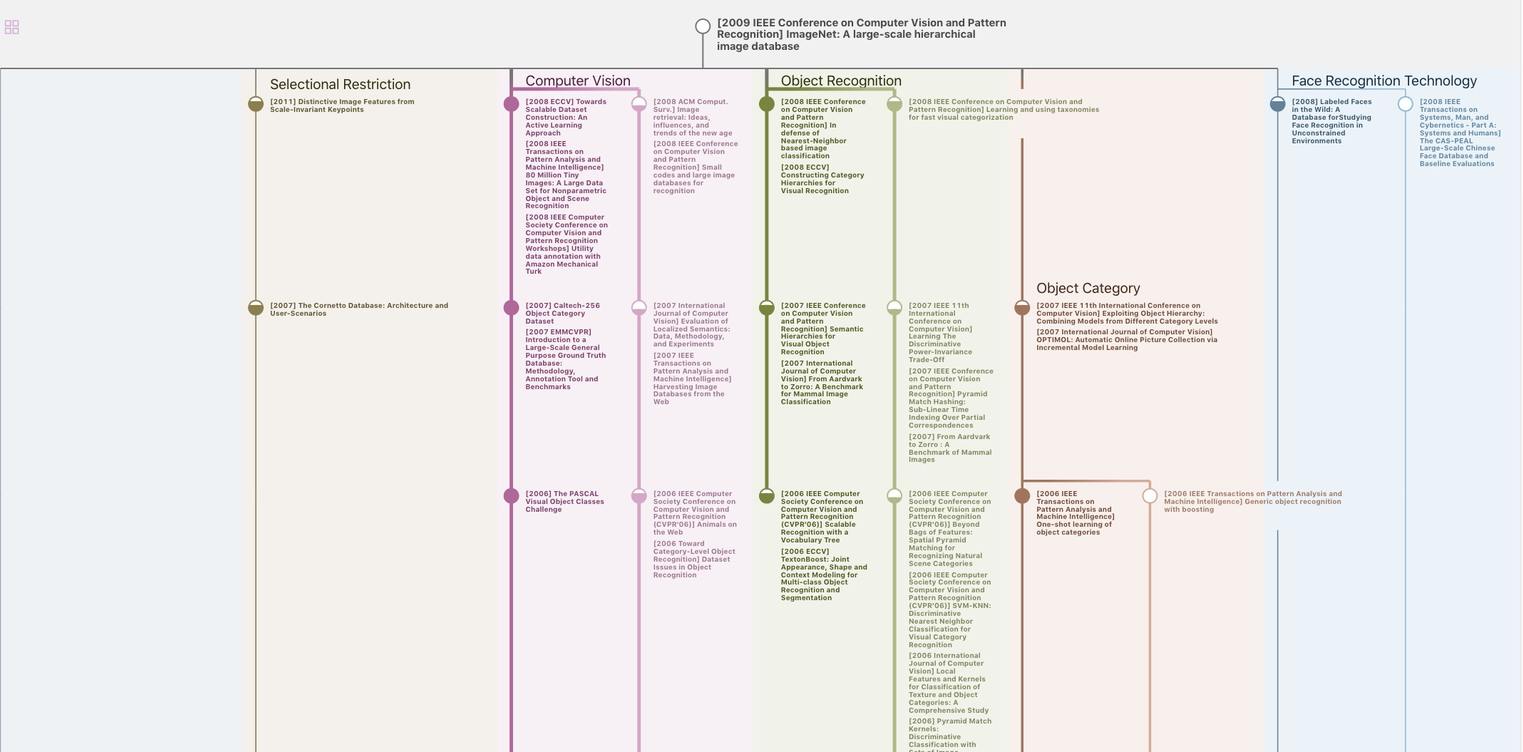
生成溯源树,研究论文发展脉络
Chat Paper
正在生成论文摘要