Evaluating Influence of Neighboring Structures on Spatial Crash Frequency Modeling and Site-Ranking Performance
TRANSPORTATION RESEARCH RECORD(2017)
摘要
Many neighborhood weight matrices have been adopted for modeling crash spatial heterogeneity. However, there has been little evaluation of their influence on crash prediction modeling performance. This study investigated 17 spatial-proximity matrices for development of spatial crash prediction models and site ranking with county-level data in California. Of the group of matrices being evaluated, traffic exposure–weighted and population-weighted distance-based matrices were first proposed in the traffic safety field. Bayesian spatial analysis was conducted with a combination of a first-order autoregressive error process and time trend for crashes to address the serial correlation of crashes in successive years. Two diagnostic measures were used for assessment of goodness of fit and complexity of models, and seven evaluation criteria were employed to assess the benefits associated with better-fitting models in site ranking. The results showed that modeling performance improved with an increase in number of neighbors considered in the weight matrix. However, a larger number of neighbors also led to greater variability of modeling performance. Specifically, Queen-2 and Decay-50 models proved to be superior among the adjacency- and distance-based models, respectively. The significance of incorporating spatial correlations was highlighted by the consistently poor performance of the base model, which included only the heterogeneity random effect. Finally, model-fitting performance seems to be strongly correlated with site-ranking performance. The models with closer goodness of fit tend to yield more consistent ranking results.
更多查看译文
关键词
Crash Prediction Models,Spatial Analysis,Spatial Modeling,Spatial Autocorrelation
AI 理解论文
溯源树
样例
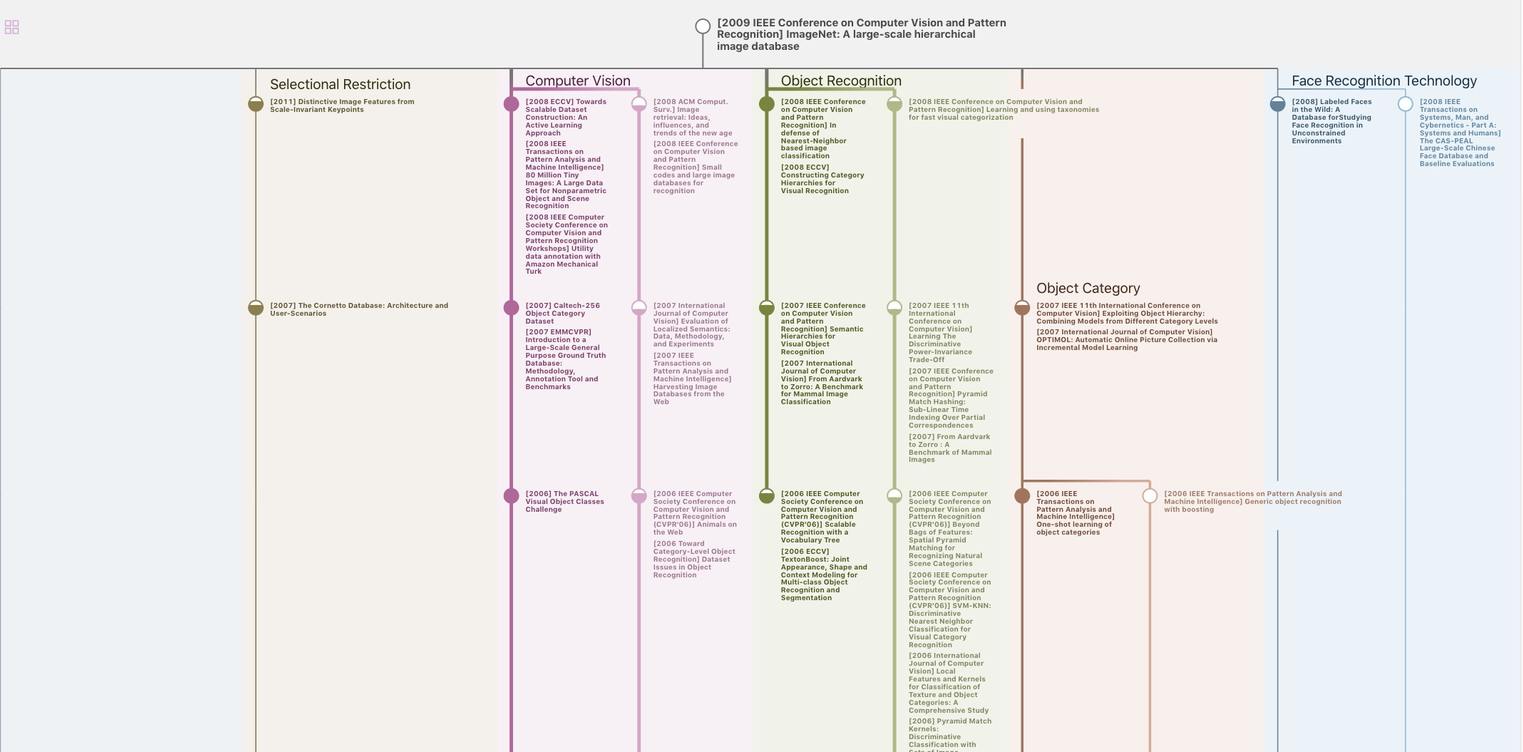
生成溯源树,研究论文发展脉络
Chat Paper
正在生成论文摘要