Aero-engine Bearing Fault Diagnosis Model Based on Optimizing Cost-Sensitive Support Vector Machine
Prognostics and System Health Management Conference(2017)
摘要
Due to the particularity of the aero-engine bearing and the limitation of the test conditions, it is difficult to get enough fault class sample data and the misclassification cost that misjudge fault sample to normal sample is higher than the opposite misjudgment, therefore the diagnosis of aero-engine bearing belongs to the typical small sample problem which is also unbalance. In order to solve this problem, this paper proposed an optimizing cost-sensitive support vector machine (CS-SVM) model. The proposed method is based on cost-sensitive support vector machine. The improved genetic immune particle swarm optimization (PSO) algorithm is applied to select the best regularized constants C+, C-, and kernel function parameter g. This paper use the optimizing CS-SVM proposed to train and predict the bearing vibration signals. The experimental results showed that the CS-SVM can effectively deal with unbalanced small fault class sample in aero-bearing fault diagnosis, it can also improve the diagnosis accuracy of the fault class sample.
更多查看译文
关键词
Bearing Fault Diagnosis,Particle Swarm Optimization (PSO),Cost-Sensitive Support Vector Machine (CS-SVM)
AI 理解论文
溯源树
样例
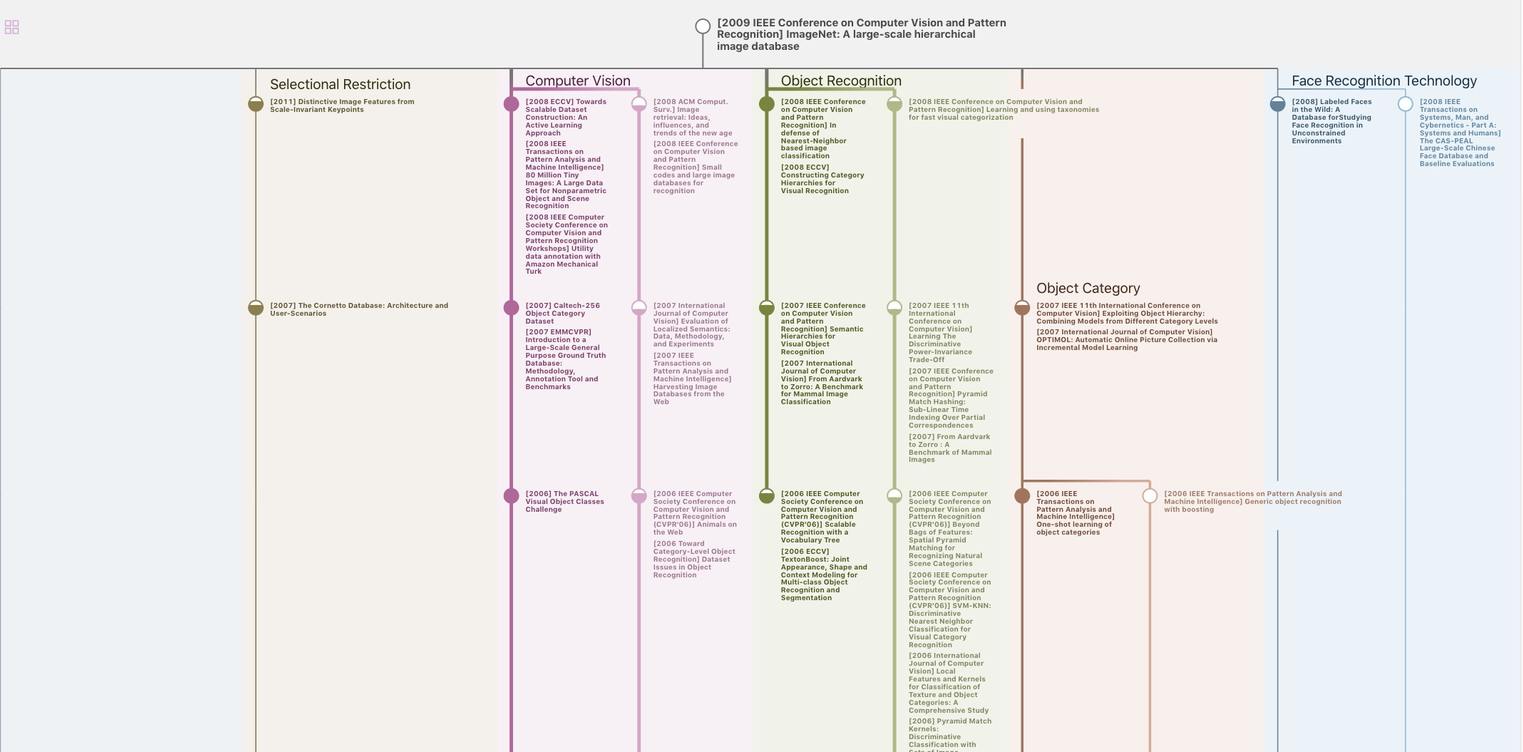
生成溯源树,研究论文发展脉络
Chat Paper
正在生成论文摘要