Rectal Cancer Outcome Study Based on Multiple NRG Oncology Clinical Trials with Random Survival Forests
International journal of radiation oncology, biology, physics(2017)
摘要
To use the machine learning Random Forest (RF) model and novel Random Survival Forest (RSF) model, to predict overall survival, local recurrence, and distant metastases for patients with locally advanced rectal cancer treated with neoadjuvant chemoradiotherapy followed by surgery, using data from RTOG 0822, 0247, and 0012. The 79 patients from RTOG 0822, 146 patients from RTOG 0247, and 106 patients from RTOG 0012 were included in the study. Data with missing clinical information or duplication were eliminated. Locally advanced rectal cancer patients were treated with neoadjuvant chemoradiotherapy and suggested total mesorectal excision. The following prognosis and clinical data were collected and applied for the RF and RSF method for survival analysis: gender, age, treatment arm, clinical tumor staging (cT, cN, and cM), pathological staging (pT, pN), tumor distance (TD) from the anorectal verge (<5 cm, 5-10 cm, >10 cm), adjuvant chemotherapy (chemo) status (Y/N) and type, radiation dose and treatment duration. Overall survival (OS), distant metastases (DS), and local recurrence (LR) were the outcomes for prediction. Features were ranked correspondingly for OS, LR and DM prediction. RSF incorporates RF and can rank features, and predict survival function over time. To pursue RSF for event-specific variable outcomes, the prediction result was five-fold cross-validated with RF. The RF analysis stabilized at 10,000 randomized decision trees, with concordance index (C-index) of 0.75, 0.80, and 0.70 for OS, LR, and DM using RTOG 0822 data. A five-fold cross-validation was performed on the 0822 patients; the “out of bag” validation training set error was 0.30, corresponding to a C-index of 0.70. The important features for OS (high to low) are pT, TD, pN, cN, cT, adjuvant (adj) chemo, dose, cM, chemo type, surgery procedure, age. For LR, importance lists: TD, cN, adj. chemo, cT, cM, dose, age, pT, sex, surg. procedure, chemo type, pN; for DM are pN, cN, TD, pT, postsurgery complications, cM, adj chemo, dose, cT, sex, age. The RSF model also calculates survival prediction over time, 96.4%, 95.5%, 90.3%, 82.5%, 75.0% at 1-, 2-, 3-, 4-, 5-year survival rate, and aligns with the Kaplan-Meier curve. For trial 0247 and trial 0012 cohorts, OS prediction generated AUC = 0.66 for RTOG 0012 and AUC = 0.65 for RTOG 0247. However, the LR and DM would not be predicted in RTOG 0822 due to the uncollected P-stage information. RF and RSF survival analysis can predict for OS, LR, and DM outcome of locally advanced rectal cancer patients. RF/RSF method features automatic data mining. More clinical trial data for further validations of the model are needed for robustness and reliability. RF/RSF also provides an alternative machine learning method for decision support of precision medicine.
更多查看译文
AI 理解论文
溯源树
样例
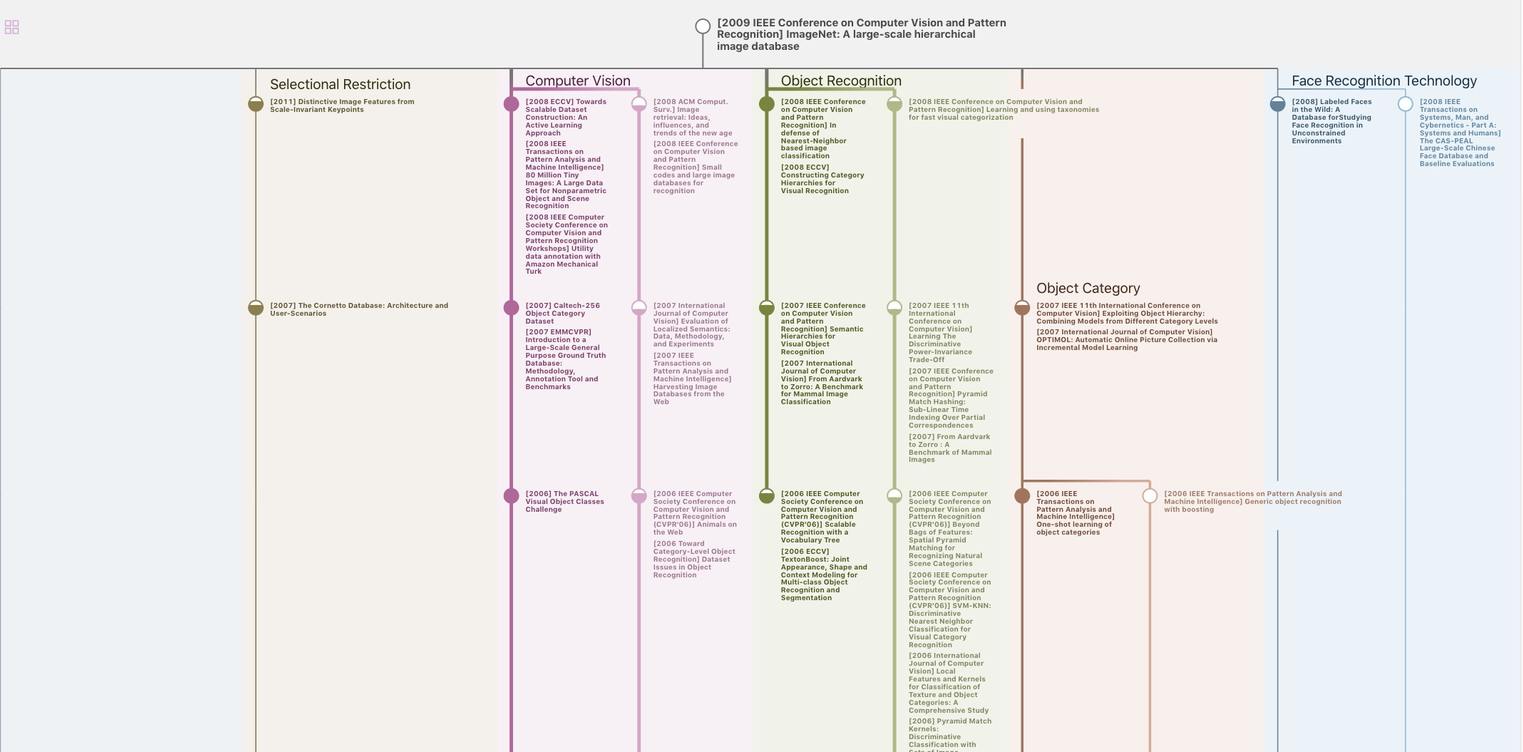
生成溯源树,研究论文发展脉络
Chat Paper
正在生成论文摘要